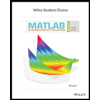
Concept explainers
What problems might we encounter when using regression to measure causal effects?

Given:
Regression is the Statistical method of detecting exact relationship between dependent variable and independent variable(or variables) i.e. it measures how changing independent variable(or variables) affects the dependent variable and quantifies the actual relationship between two using correlation coefficient.
Regression cares about correlation relationships but correlation does not necessary mean causation but causation implies correlation. For eg, Age and shoe size are correlated but age does not causes increase in shoe size. Causal analysis tries to estimate the effect of interventions and measures how X affects Y. A cause is the variable that produces an event or condition and effect is that variable which is result of that event.
Step by stepSolved in 2 steps

- 1. Sue is conducting a regression analysis to predict the amount of salaries expense using the number of employees and the average rate of pay. This type of regression analysis is called a(n) independent regression. linear regression. multiple regression. dependent regression.arrow_forwardIf a regression line for two variables has a small positive slope, then the: variables are positively associated? variables are negatively associated? association of the variables cannot be determined. variables have no association with each other.arrow_forwardPlease see problem with the solution: after reviewing the information below, assess the appropriateness and accuracy of using a linear regression model. Discuss the meaning of the standard error of the estimate and how it affects the predicted values of Y for that analysis. Many people are also using online shopping to avoid going to stores in person. I decided to use my own data. I am going to add how many Amazon transactions I have made each month from March- August. X= the month Y= amount of purchases Simple linear regression results: Dependent Variable: saleIndependent Variable: monthsale = 0.53333333 + 1.6 monthSample size: 6R (correlation coefficient) = 0.99410024R-sq = 0.98823529Estimate of error standard deviation: 0.36514837 Parameter estimates: Parameter Estimate Std. Err. Alternative DF T-Stat P-value Intercept 0.53333333 0.50269117 ≠ 0 4 1.0609562 0.3485 Slope 1.6 0.087287156 ≠ 0 4 18.330303 <0.0001 Analysis of…arrow_forward
- What is the difference between the following two regression equations? y=b0+b1x y=B0+B1xarrow_forwardDiscuss the reasons and situations in which researchers would want to use linear regression. How would a researcher know whether linear regression would be the appropriate statistical technique to use? What are some of the benefits of fitting the relationship between two variables to an equation for a straight line?arrow_forwardIf the R-squared for a regression model relating the outcome y to an explanatory variable x is 0.9. This implies that there is a positive linear relationship between y and x. True or false?arrow_forward
- Part 5 onlyarrow_forwardDuring the 1950's and 1960's the average weight of vehicles sold in the U.S. was well over 4,000 pounds. There was a dip in the average weights in the 1970's and 1980's, due possibly to both higher demand for better gas mileage and a world-wide shortage of crude oil. Then in the 1990's and early 2000's the average weight of vehicles had a steady increase. A regression analysis was completed on the average weight of the 10 most commonly sold vehicles in the U.S. from the years 2012 through the year 2020 and yielded the following results, where the independent variable is the year and the predicted variable is the average weight of the 10 most popular vehicles. Correlation of "Average Weight" and "Year" = r = 0.9283 The regression equation is "Average Weight" = –124,960.73 + 63.82(Year) Predict the Average Weight to the nearest pound for the 2022 Year. Group of answer choices A. 4057 pounds B. 4015 pounds C. This value of Year is beyond the scope of the…arrow_forwardWhich statement is not correct for multiple regression model? When we interpret this categorical variable, we would say the change we observe when one switches from the reference category to the specific category. The reference category will not be displayed in our model results. If we include a categorical variable with more than two values (e.g., religion) as an independent variable, we want to include a dummy variable for all categories except for two. We can include more than two categorical variables in the model. The value of the independent variable not included in the model is called "reference category."arrow_forward
- To properly examine the effect of a categorical independent variable in a multiple linear regression model we use an interaction term. True O Falsearrow_forwardAccording to an article, one may be able to predict an individual's level of support for ecology based on demographic and ideological characteristics. The multiple regression model proposed by the authors was the following. y = 3.60-.01x₁+.01.₂-.07x3+.12x4+.02xs-.04x6-01-.04.xg-.02.xg+c The variables are defined as follows. y = ecology score (higher values indicate a greater concern for ecology) X₁ = age times 10 x₂ = income (in thousands of dollars) x3 = gender (1 = male, 0 = female) X4 = race (1 = white, 0 = nonwhite) X5 = education (in years) x6 = ideology (4 = conservative, 3 = right of center, 2 = middle of the road, 1 = left of center, and 0 = liberal) X7 = social class (4 = upper, 3 = upper middle, 2 = middle, 1 = lower middle, 0 = lower) xg = postmaterialist (1 if postmaterialist, 0 otherwise) x9 = materialist (1 if materialist, 0 otherwise) (a) Suppose you knew a person with the following characteristics: a 30 year old, white female with a college degree (20 years of…arrow_forwardA new footwear manufacturer is trying to compete with the major brands by investing heavily in Research and Development (R&D). Their goal is to produce a premium lifestyle sneaker that is more comfortable, longer lasting and more stylish than any of their competitors. Below are some data on dollars spent on R&D and new customers acquired for the last 5 quarters. Regression Equation: Y= 0.5 + 1.35X New Customers R&D Dollars Acquired (in millions) (in thousands) 8 12 12 15 13 18 14 20 18 25 Based on looking at the numbers and/or sketching a scatter-plot, what conclusion can we draw about the data? The variables are negatively correlated You cannot tell until we perform some calculations There does not appear to be a strong correlation between the variables The variables are positively correlatedarrow_forward
- MATLAB: An Introduction with ApplicationsStatisticsISBN:9781119256830Author:Amos GilatPublisher:John Wiley & Sons IncProbability and Statistics for Engineering and th...StatisticsISBN:9781305251809Author:Jay L. DevorePublisher:Cengage LearningStatistics for The Behavioral Sciences (MindTap C...StatisticsISBN:9781305504912Author:Frederick J Gravetter, Larry B. WallnauPublisher:Cengage Learning
- Elementary Statistics: Picturing the World (7th E...StatisticsISBN:9780134683416Author:Ron Larson, Betsy FarberPublisher:PEARSONThe Basic Practice of StatisticsStatisticsISBN:9781319042578Author:David S. Moore, William I. Notz, Michael A. FlignerPublisher:W. H. FreemanIntroduction to the Practice of StatisticsStatisticsISBN:9781319013387Author:David S. Moore, George P. McCabe, Bruce A. CraigPublisher:W. H. Freeman
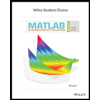
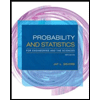
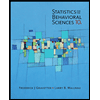
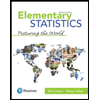
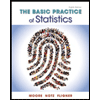
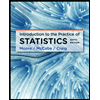