Concept explainers
i.
To identify:
The independent variable
i.

Answer to Problem 13.4E
Independent variable
Explanation of Solution
Given information:
Formula used:
The student’s t- statistics is given by the following expression:
The values:
Calculation:
To identify which independent variable
The
To test the null hypothesis:
Vs
And,
1. At
The student’s t- statistics is calculated as:
The corresponding
Reject the null hypothesis
Hence, independent variable
2. At
The student’s t- statistics is calculated as:
The corresponding
Reject the null hypothesis
Hence, independent variable
3. At
The student’s t- statistics is calculated as:
The corresponding
Reject the null hypothesis
Hence, independent variable
Conclusion:
Therefore, all independent variable
ii.
The least-square prediction equation
ii.

Answer to Problem 13.4E
The least-square prediction equation is
Explanation of Solution
Given information:
Calculation:
The line which makes the vertical distance from the data points to the regression line is known as least-square regression. This distance is as small as possible.
The least-square prediction equation is given by the following eq:
Put the given values:
Conclusion:
Hence, least-square prediction equation is derived as
iii.
To explain:
The relationship between lines shown by the graph the relationship between
iii.

Answer to Problem 13.4E
Lines shown by the graph is appearing to be parallel with each other
Explanation of Solution
Given information:
Predictor variables:
Calculation:
The given equation is
When
When
The below graph depicting the relationship between
Two lines shown by graph are seems to be parallel with each other.
Conclusion:
The above graph depicting the relationship between the two lines and they appear to be parallel to each other.
iv.
To explain:
The practical interpretation of
iv.

Answer to Problem 13.4E
The measurement of changes in
Explanation of Solution
Three-dimensional extension line of means is depicted by the given eq.
When
Partial slopes of the model is denoted by
The measurement of changes occurs in
The slope estimated by a fit line with
Conclusion:
Hence, the unknown constant values are estimated by using the sample data.
Want to see more full solutions like this?
Chapter 13 Solutions
EP INTRODUCTION TO PROBABILITY+STAT.
- Olympic Pole Vault The graph in Figure 7 indicates that in recent years the winning Olympic men’s pole vault height has fallen below the value predicted by the regression line in Example 2. This might have occurred because when the pole vault was a new event there was much room for improvement in vaulters’ performances, whereas now even the best training can produce only incremental advances. Let’s see whether concentrating on more recent results gives a better predictor of future records. (a) Use the data in Table 2 (page 176) to complete the table of winning pole vault heights shown in the margin. (Note that we are using x=0 to correspond to the year 1972, where this restricted data set begins.) (b) Find the regression line for the data in part ‚(a). (c) Plot the data and the regression line on the same axes. Does the regression line seem to provide a good model for the data? (d) What does the regression line predict as the winning pole vault height for the 2012 Olympics? Compare this predicted value to the actual 2012 winning height of 5.97 m, as described on page 177. Has this new regression line provided a better prediction than the line in Example 2?arrow_forwardFor the following exercises, use Table 4 which shows the percent of unemployed persons 25 years or older who are college graduates in a particular city, by year. Based on the set of data given in Table 5, calculate the regression line using a calculator or other technology tool, and determine the correlation coefficient. Round to three decimal places of accuracyarrow_forwardFor the following exercises, consider the data in Table 5, which shows the percent of unemployed in a city ofpeople25 years or older who are college graduates is given below, by year. 41. Based on the set of data given in Table 7, calculatethe regression line using a calculator or othertechnology tool, and determine the correlationcoefficient to three decimal places.arrow_forward
- Using the lengths (in.), chest sizes (in.), and weights (lb) of bears from a data set, the resulting regression equation is Weight= -274 +0.426 Length + 12.1 Chest Size. The P-value is 0.000 and the adjusted R² value is 0.925. If an additional predictor variable of neck size (in.) is included, the P-value becomes 0.000 and the adjusted R² becomes 0.933. Why is it better to use values of adjusted R² instead of simply using values of R²? Choose the correct answer below. C O A. The unadjusted R² can only be calculated for regression equations with P-values greater than 0, while the adjusted R² can be calculated for regression equations with any manner of P-value. O B. The unadjusted R² increases or remains the same as more variables included, but the adjusted R² is adjusted for the number of variables and sample size. OC. The unadjusted R² decreases or remains the same as more variables are included, but the adjusted R² is adjusted for the number of variables and sample size. O D. The…arrow_forwardThe St. Lucian Government is interested in predicting the number of weekly riders on the public buses using the following variables: • • • • Price of bus trips per weekThe population in the cityThe monthly income of ridersAverage rate to park your personal vehicle Determine the multiple regression equation for the data. What is the predicted value of the number of weekly riders if: price of bus trips per week = $24; population = $2,000,000; the monthly income of riders = $13,500; and average rate to park your personal vehicle = $150. Interpret the coefficient of determination.arrow_forwardThe manufacturer of Beanie Baby dolls used quarterly price data for 2012/- 2020/V (t = 1, ..., 36) and the regression equation Pt = a + bt + c₁D1 t + c2 D2t + c3D3 t to forecast doll prices in the year 2021. Pt is the quarterly price of dolls, and D1, D2t, and D3 are dummy variables for quarters I, II, and III, respectively. DEPENDENT VARIABLE: PT R-SQUARE P-VALUE ON F 0.0001 OBSERVATIONS: 36 0.9078 F-RATIO 76.34 STANDARD ERROR PARAMETER ESTIMATE T-RATIOP-VALUE VARIABLE INTERCEPT 24.0 6.20 3.87 0.0005 T 0.8 0.240 3.33 0.0022 D1 -8.0 2.60 -3.08 0.0043 D2 -6.0 1.80 -3.33 0.0022 D3 -4.0 0.60 -6.67 0.0001 In any given year price tends to vary from quarter to quarter as follows: Multiple Choice O O O P₁ > P|| > P||| > PIV PI> PIV> Pill > PII Pll > Pill > PIV> PI Pill > PI>PII > PIV PIV> Pill > PII > PIarrow_forward
- Q: The dataset posted below lists a sample of months and the advertising budget (in hundreds of dollars) for TV, radio and newspaper advertisements. Also included is whether a coupon was published for that month and the resulting sales (in thousands of dollars). a) Develop a multiple regression model predicting the sales based off the four predictor variables: TV, radio, and newspaper advertising budget and whether a coupon is used. Recode Coupon as 0 = No and 1 = Yes. Report the estimated regression equation (Solve in Excel) TV ($100) radio ($100) newspaper ($100) Coupon sales ($1000) 0.7 39.6 8.7 No 1.6 230.1 37.8 69.2 No 22.1 4.1 11.6 5.7 Yes 3.2 44.5 39.3 45.1 No 10.4 250.9 36.5 72.3 No 22.2 8.6 2.1 1 No 4.8 17.2 45.9 69.3 Yes 9.3 104.6 5.7 34.4 No 10.4 216.8 43.9 27.2 Yes 22.3 5.4 29.9 9.4 No 5.3 69 9.3 0.9 No 9.3 70.6 16 40.8 No 10.5 151.5 41.3 58.5 No 18.5 195.4 47.7 52.9 Yes 22.4 13.1 0.4 25.6 Yes 5.3 76.4 0.8…arrow_forwardGiven below are results from the regression analysis where the dependent variable is the number of weeks a worker is unemployed due to a layoff (Unemploy) and the independent variables are the age of the worker (Age), the number of years of education received (Edu), the number of years at the previous job (Job Yr), a dummy variable for marital status (Married: 1=married, 0=otherwise), a dummy variable for head of household (Head: 1=yes, 0=no) and a dummy variable for management position (Manager: 1=yes, 0=no). We shall call this Model 1. The coefficient of partial determination (R2Yj.(All variables except j)) of each of the six predictors are, respectively, 0.2807, 0.0386, 0.0317, 0.0141, 0.0958, and 0.1201. Model 2 is the regression analysis where the dependent variable is Unemploy and the independent variables are Age and Manager. The results of the regression analysis are given. Refer to model 1. Which of the following is the correct null hypothesis to test…arrow_forwardA vending machine company operates coffee vending machines in office buildings. The company wants to study the relationship between the number of cups of coffee sold per day and the number of persons working in each building. Data were collected were collected by the company and presented below. Number of Number of Cups of f. Make a copy of the scatter diagram in this item. Draw the line that best fits in the scatter diagram using the regression equation. persons Coffee Sold working at Location g. Locate the (X,). Describe its location in relation to the other plotted data. 15 20 10 15 h. Give a practical interpretation of the values of a and b. 16 20 i. Show that the regression equation can be expressed in the form: (y - y) = b(x - x) 18 25 22 30arrow_forward
- The manufacturer of Beanie Baby dolls used quarterly price data for 2005 - 2013 IV (t= 1, ..., 36) and the regression equation Pt = a + bt+ c ₁ D1 t + c ₂ D2t+ c3 D3 t to forecast doll prices in the year 2014. Pt is the quarterly price of dolls, and D1 t, D2 t, and D3 tare dummy variables for quarters I, II, and III, respectively. DEPENDENT VARIABLE: PT R-SQUARE 0.9078 P-VALUE ON F 0.0001 OBSERVATIONS: 36 PARAMETER ESTIMATE F-RATIO 76.34 STANDARD ERROR 6.20 VARIABLE T-RATIO INTERCEPT 24.0 3.87 T 0.800 0.240 3.33 D1 -8.0 2.60 -3.08 -6.00 D2 1.80 -3.33 -4.0 0.60 -6.67 D3 What is the estimated intercept of the trend line in the 1st quarter? 32 O 24 O-8 16 Activa Go to S O none of the above P-VALUE 0.0005 0.0022 0.0043 0.0022 0.0001arrow_forwardThe accompanying data represent the weights of various domestic cars and their gas mileages in the city. The linear correlation coefficient between the weight of a car and its miles per gallon in the city is r= - 0.984. The least-squares regression line treating weight as the explanatory variable and miles per gallon as the response variable is y = - 0.0066x + 43.3954. Complete parts (a) and (b) below. Click the icon to view the data table. (a) What proportion of the variability in miles per gallon is explained by the relation between weight of the car and miles per gallon? Data Table The proportion of the variability in miles per gallon explained by the relation between weight of the car and miles per gallon is %. (Round to one decimal place as needed.) (b) Interpret the coefficient of determination. Full data set % of the variance in is by the linear model. Miles per Miles per Weight (pounds), x Weight (pounds), x Car Car (Round to one decimal place as needed.) Gallon, y Gallon, y…arrow_forwardLast years Data Management class decided to see if there was a relationship between the score (out of 10) a student got on the two-variable stats quiz, and their score (out of 30) on the unit test. Use the given data to Quiz Score Test Score 6 8 10 9 10 20 26 29 26 30 a) Calculate the correlation coefficient. b) Perform a linear regression.arrow_forward
- Glencoe Algebra 1, Student Edition, 9780079039897...AlgebraISBN:9780079039897Author:CarterPublisher:McGraw HillLinear Algebra: A Modern IntroductionAlgebraISBN:9781285463247Author:David PoolePublisher:Cengage Learning
- Elementary Linear Algebra (MindTap Course List)AlgebraISBN:9781305658004Author:Ron LarsonPublisher:Cengage LearningAlgebra & Trigonometry with Analytic GeometryAlgebraISBN:9781133382119Author:SwokowskiPublisher:Cengage
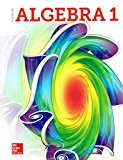
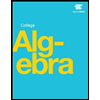
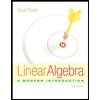
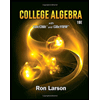
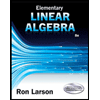