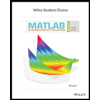
3.
You estimated a regression with the following output.
Source | SS df MS Number of obs = 400
-------------+---------------------------------- F(1, 398) = 716.92
Model | 22118655.1 1 22118655.1 Prob > F = 0.0000
Residual | 12279166.2 398 30852.1764 R-squared = 0.6430
-------------+---------------------------------- Adj R-squared = 0.6421
Total | 34397821.3 399 86210.0784 Root MSE = 175.65
------------------------------------------------------------------------------
Y | Coef. Std. Err. t P>|t| [95% Conf. Interval]
-------------+----------------------------------------------------------------
X | 5.299576 .1979268 26.78 0.000 4.910464 5.688689
_cons | 67.86719 18.3827 3.69 0.000 31.72786 104.0065
------------------------------------------------------------------------------
Which of the following is the estimated regression line?
Y = 0.20 + 18.38*X
Y = 5.30 + 67.87*X
Y = 67.87 + 5.30*X
Y = 18.38 + 0.20*X

Step by stepSolved in 2 steps

- Nonearrow_forwardYou believe that the price of Zoom Videoconferencing stock and the price of American Airlines stock will move in opposite directions. In order to test this relationship, we do a simple regression with the following variables:A - dependent variable : month end price of American Airlines stockZ - independent variable: month end price of Zoom Videoconferencing stock Data from April 2019 through December 2020 (21 observations) is availableBased on the data, we compute the following:Var (Z) = 20927.702Cov (A,Z) = -899.153E(A) = 20.790E(Z) = 187.530Std Error of Estimate = 6.088TSS = 1476.830 Consider the equation At = b0 + b1 Zt + εtBased on the numbers given above, complete the following table Variable Estimate Std error t-statistic Slope b1 .00941 Constant b0 2.2088 R-square N/A N/A F statistic N/A N/A Are the coefficients (slope and/or constant) significant at the .05 level?arrow_forwardYou estimated a regression with the following output. Source | SS df MS Number of obs = 325 -------------+---------------------------------- F(1, 323) = 42850.36 Model | 285905003 1 285905003 Prob > F = 0.0000 Residual | 2155111.65 323 6672.17228 R-squared = 0.9925 -------------+---------------------------------- Adj R-squared = 0.9925 Total | 288060115 324 889074.429 Root MSE = 81.683 ------------------------------------------------------------------------------ Y | Coef. Std. Err. t P>|t| [95% Conf. Interval] -------------+---------------------------------------------------------------- X | 11.83842 .0571895 207.00 0.000 11.72591 11.95093 _cons | 52.14457 5.949458 8.76 0.000 40.43999 63.84915…arrow_forward
- 10. You estimated a regression with the following output. Source | SS df MS Number of obs = 333 -------------+---------------------------------- F(1, 331) = 4608.21 Model | 32636494.1 1 32636494.1 Prob > F = 0.0000 Residual | 2344225.8 331 7082.25316 R-squared = 0.9330 -------------+---------------------------------- Adj R-squared = 0.9328 Total | 34980719.9 332 105363.614 Root MSE = 84.156 ------------------------------------------------------------------------------ Y | Coef. Std. Err. t P>|t| [95% Conf. Interval] -------------+---------------------------------------------------------------- X | 30.79902 .4537022 67.88 0.000 29.90652 31.69153 _cons | 20.85313 42.1964 0.49 0.621 -62.1538 103.8601…arrow_forwardIn a regression analysis if SSE = 64 and SSR = 364, then what is the coefficient of determination? (please keep 2 decimal places)arrow_forwardHello! Please analyse the table 3 and 4 in the image attached. the data is supposed to see if there is a relationship between the prevalence of obesity(table3) or life expectancy (table4) between males and females. thank you!arrow_forward
- You estimated a regression with the following output. Source | SS df MS Number of obs = 246 -------------+---------------------------------- F(1, 244) = 16642.70 Model | 187647307 1 187647307 Prob > F = 0.0000 Residual | 2751112.55 244 11275.0514 R-squared = 0.9856 -------------+---------------------------------- Adj R-squared = 0.9855 Total | 190398419 245 777136.405 Root MSE = 106.18 ------------------------------------------------------------------------------ Y | Coef. Std. Err. t P>|t| [95% Conf. Interval] -------------+---------------------------------------------------------------- X | 13.79687 .106947 129.01 0.000 13.58621 14.00753 _cons | 17.60822 9.208341 1.91 0.057 -.5297613 35.7462…arrow_forwardYou estimated a regression with the following output. Source | SS df MS Number of obs = 494 -------------+---------------------------------- F(1, 492) = 38566.69 Model | 803403712 1 803403712 Prob > F = 0.0000 Residual | 10249120.6 492 20831.546 R-squared = 0.9874 -------------+---------------------------------- Adj R-squared = 0.9874 Total | 813652832 493 1650411.42 Root MSE = 144.33 ------------------------------------------------------------------------------ Y | Coef. Std. Err. t P>|t| [95% Conf. Interval] -------------+---------------------------------------------------------------- X | 23.00296 .1171325 196.38 0.000 22.77281 23.2331 _cons | 34.71944 13.12788 2.64 0.008 8.925808 60.51307…arrow_forwardYou estimated a regression with the following output. Source | SS df MS Number of obs = 423 -------------+---------------------------------- F(1, 421) = 267.80 Model | 8758968.84 1 8758968.84 Prob > F = 0.0000 Residual | 13769523.8 421 32706.7074 R-squared = 0.3888 -------------+---------------------------------- Adj R-squared = 0.3873 Total | 22528492.7 422 53385.0537 Root MSE = 180.85 ------------------------------------------------------------------------------ Y | Coef. Std. Err. t P>|t| [95% Conf. Interval] -------------+---------------------------------------------------------------- X | 6.150402 .3758334 16.36 0.000 5.411658 6.889145 _cons | -8.022201 24.02003 -0.33 0.739 -55.23632 39.19192…arrow_forward
- The slope, b represents * O predicted value of Y when X = 0. O the estimated average change in Y per unit change in X. O variation around the line of regression. O the predicted value of Y.arrow_forward2. You estimated a regression with the following output. Source | SS df MS Number of obs = 332 -------------+---------------------------------- F(1, 330) = 2.32 Model | 71599.1822 1 71599.1822 Prob > F = 0.1284 Residual | 10170207.7 330 30818.8111 R-squared = 0.0070 -------------+---------------------------------- Adj R-squared = 0.0040 Total | 10241806.9 331 30942.0147 Root MSE = 175.55 ------------------------------------------------------------------------------ Y | Coef. Std. Err. t P>|t| [95% Conf. Interval] -------------+---------------------------------------------------------------- X | .7575183 .4969893 1.52 0.128 -.2201484 1.735185 _cons | 28.87757 42.80371 0.67 0.500 -55.32498 113.0801…arrow_forward
- MATLAB: An Introduction with ApplicationsStatisticsISBN:9781119256830Author:Amos GilatPublisher:John Wiley & Sons IncProbability and Statistics for Engineering and th...StatisticsISBN:9781305251809Author:Jay L. DevorePublisher:Cengage LearningStatistics for The Behavioral Sciences (MindTap C...StatisticsISBN:9781305504912Author:Frederick J Gravetter, Larry B. WallnauPublisher:Cengage Learning
- Elementary Statistics: Picturing the World (7th E...StatisticsISBN:9780134683416Author:Ron Larson, Betsy FarberPublisher:PEARSONThe Basic Practice of StatisticsStatisticsISBN:9781319042578Author:David S. Moore, William I. Notz, Michael A. FlignerPublisher:W. H. FreemanIntroduction to the Practice of StatisticsStatisticsISBN:9781319013387Author:David S. Moore, George P. McCabe, Bruce A. CraigPublisher:W. H. Freeman
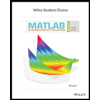
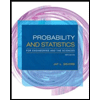
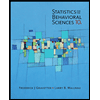
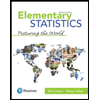
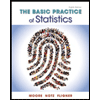
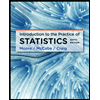