You estimated the following regression. Which of the following is the estimated regression line? Source | SS df MS Number of obs = 161 -------------+---------------------------------- F(1, 159) = 541.37 Model | 18320668.9 1 18320668.9 Prob > F = 0.0000 Residual | 5380812.42 159 33841.5875 R-squared = 0.7730 -------------+---------------------------------- Adj R-squared = 0.7715 Total | 23701481.3 160 148134.258 Root MSE = 183.96 ------------------------------------------------------------------------------ Y | Coef. Std. Err. t P>|t| [95% Conf. Interval] -------------+---------------------------------------------------------------- X | 13.8887 .5969203 23.27 0.000 12.70979 15.06762 _cons | 88.26833 41.00599 2.15 0.033 7.281658 169.255 ------------------------------------------------------------------------------ Group of answer choices a Y = 41.01 + 0.60*X b Y = 88.27 + 13.89*X c Y = 13.89 + 88.27*X d Y = 0.60 + 41.01*X
Correlation
Correlation defines a relationship between two independent variables. It tells the degree to which variables move in relation to each other. When two sets of data are related to each other, there is a correlation between them.
Linear Correlation
A correlation is used to determine the relationships between numerical and categorical variables. In other words, it is an indicator of how things are connected to one another. The correlation analysis is the study of how variables are related.
Regression Analysis
Regression analysis is a statistical method in which it estimates the relationship between a dependent variable and one or more independent variable. In simple terms dependent variable is called as outcome variable and independent variable is called as predictors. Regression analysis is one of the methods to find the trends in data. The independent variable used in Regression analysis is named Predictor variable. It offers data of an associated dependent variable regarding a particular outcome.
You estimated the following regression. Which of the following is the estimated regression line?
Source | SS df MS Number of obs = 161
-------------+---------------------------------- F(1, 159) = 541.37
Model | 18320668.9 1 18320668.9 Prob > F = 0.0000
Residual | 5380812.42 159 33841.5875 R-squared = 0.7730
-------------+---------------------------------- Adj R-squared = 0.7715
Total | 23701481.3 160 148134.258 Root MSE = 183.96
------------------------------------------------------------------------------
Y | Coef. Std. Err. t P>|t| [95% Conf. Interval]
-------------+----------------------------------------------------------------
X | 13.8887 .5969203 23.27 0.000 12.70979 15.06762
_cons | 88.26833 41.00599 2.15 0.033 7.281658 169.255
------------------------------------------------------------------------------
a Y = 41.01 + 0.60*X
b Y = 88.27 + 13.89*X
c Y = 13.89 + 88.27*X
d Y = 0.60 + 41.01*X

Trending now
This is a popular solution!
Step by step
Solved in 2 steps with 1 images

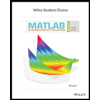
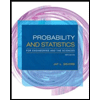
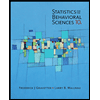
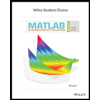
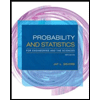
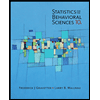
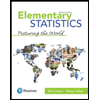
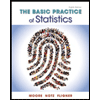
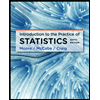