A company reports bi-annual (twice a year) sales data. The sales data for the last three years is shown in below Table. The intercept (a) of the regressions line (DV: Sales, IV: Obs) is equal to A 2 B 4 C 3 D 1 The slope (b) of the regressions line (DV: Sales, IV: Obs) is equal to a 4 b 2 c 3 d 1
Questions 1-30 refer to the following scenario: A company reports bi-annual (twice a year) sales data. The sales data for the last three years is shown in below Table.
The intercept (a) of the regressions line (DV: Sales, IV: Obs) is equal to
A
|
2 |
B
|
4 |
C
|
3 |
D
|
1 |
The slope (b) of the regressions line (DV: Sales, IV: Obs) is equal to
a |
4 |
b |
2 |
c |
3 |
d |
1 |
The standard error of the regression is
a |
5.34 |
b |
3.54 |
c |
4.35 |
d |
2.51 |
The standard error of the intercept is
a |
1.30 |
b |
2.30 |
c |
0.30 |
d |
3.30 |
You want to test the hypothesis that the intercept is statistically significantly different from zero. To do so, you use the attached t-table. Your alpha (the risk you accept to make a Type I error) is a=0.1. How many degrees of freedom do you have?
a |
n |
b |
n-2 |
c |
4 |
d |
both b. and c. are correct |
You want to test the hypothesis that the intercept is statistically significantly different from zero. To do so, you use the attached t-table. Your alpha (the risk you accept to make a Type I error) is a=0.1. What is your t-statistic to conduct the hypothesis test?
a |
t=0.71 |
b |
t=0.81 |
c |
t=0.91 |
d |
t=1.01 |
The standard error of the slope is
a |
0.95 |
b |
1.15 |
c |
1.05 |
d |
0.85 |
You want to test the hypothesis that the slope coefficient is statistically significantly different from zero. To do so, you use the attached t-table. Your alpha (the risk you accept to make a Type I error) is a=0.1. What is your t-statistic to conduct the hypothesis test?
a |
4.35 |
b |
3.35 |
c |
2.35 |
d |
1.35 |
Which statement is true? Given your alpha of a=0.1, you
a |
Reject the null for the intercept, and fail to reject the null for the slope |
b |
Reject the null for the slope, and fail to reject the null for the intercept |
c |
Fail to reject the null for both the intercept and the slope |
d |
Reject the null for both the intercept and the slope |
The explained sum of squares of the regression is ESS =
a |
20 |
b |
120 |
c |
70 |
d |
50 |


Trending now
This is a popular solution!
Step by step
Solved in 5 steps with 1 images

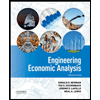
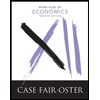
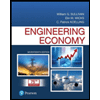
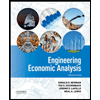
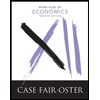
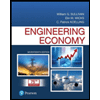
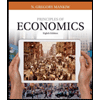
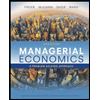
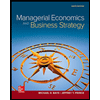