What is Statistical Bias?
Statistics is the study of data collection, organization, analysis, interpretation, and presentation. Statistical bias is a characteristic of a statistical technique or its findings in which the expected value deviates from the actual root quantitative parameter being estimated. According to the actual definition of bias, it refers to the tendency of a statistic to overestimate or underestimate a parameter.
Biased /Unbiased Estimators
An estimator is a rule in statistics that calculates an estimate of a volume based on the observed data. The bias of an estimator is the difference between the expected value of the statistic and the real value of the sample statistic. An unbiased estimator is one that is a true reflection of a sample statistic. A biased estimator is one that is not a real reflection of a population parameter.
The diagram below depicts the difference between a biased estimator and an unbiased estimator. In the diagram, PDF refers to the probability density function.
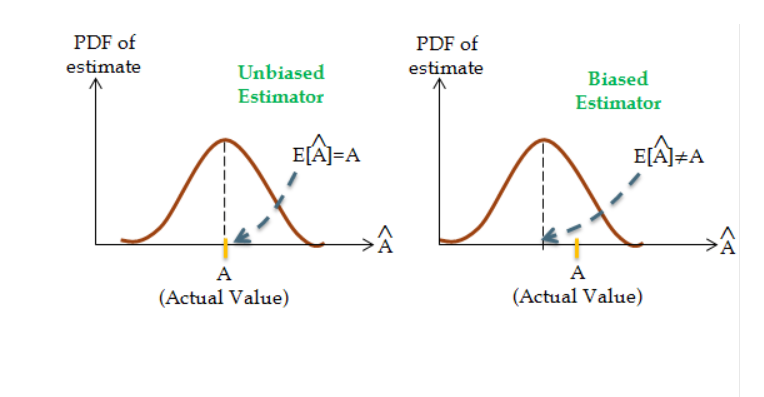
Hypothesis
Statistical Hypothesis - A statistical hypothesis is the statement about the characteristics of a population. It is frequently expressed in terms of a population parameter.
Null Hypothesis - A statistical hypothesis that is to be tested is known as a null hypothesis.
Alternative Hypothesis - The alternative statement to the null hypothesis is the alternative hypothesis.
Types of Bias
The types of bias are listed below.
- Selection Bias - The bias introduced by selecting individuals, groups, or data for analysis in such a way that proper randomization is not achieved, resulting in a sample that is not representative of the population intended to be analyzed. The selection effect is another name for it.
Sampling bias is commonly regarded as a subtype of selection bias. Sampling bias is a statistical bias that occurs when a sample is collected in such a way that some participants of the intended population have a lower or higher sampling probability than others. It yields a biased sample, a non-random sample of a population (or non-human factors) in which all individuals or instances were not equally likely to be chosen.
- Cognitive Bias- A cognitive bias is a systematic pattern of judgmental deviation from norm or rationality. Based on their perception of the input, individuals construct their own "subjective reality". An individual's perception of reality, rather than objective input, may influence their behaviour in the world. As a result, cognitive biases can sometimes result in perceptual distortion, incorrect judgement, and illogical interpretation.
Types of Cognitive Bias
1. Hindsight Bias- Hindsight bias is the after-the-fact belief that one "always knew" they were correct. Someone may also mistakenly believe they have special insight or talent for predicting outcomes. This is a key concept in behavioural finance theory.
2. Confirmation Bias- Confirmation bias occurs when a decision-maker has serious preconceptions and listens only to the part of your demonstration that confirms their beliefs, ignoring the rest. Suggestion: always have a one-sentence takeaway for your presentations, which is impossible to miss even if someone's eyes are obscured by preconceptions.
3. Dunning–Kruger Effect- The Dunning–Kruger effect is a fictitious cognitive bias in which people with low potential overestimate their ability. The bias is caused by an inbuilt illusion in people with low potential and an external misconception in people with high ability; that is, "the miscalibration of the incompetent stems forms an error about the self, whereas the miscalibration of the highly competent stems forms an error about others".
- Observer Bias- In research, observer bias is a type of detection bias that occurs during the observation or recording stage of a study. For example, in the evaluation of medical images, one observer may record a disorder while another does not. Different observers may have a proclivity to round up or round down a measurement scale.
- Survivorship Bias- Survivorship bias is a type of statistical bias in which the researcher focuses only on the part of the data set that has already been pre-selected – and ignores the data points that have dropped off during this process (because they are not visible anymore).
- Omitted-Variable Bias- Omitted-variable bias can occur when one or more significant variables are excluded from your model. This issue arises frequently in the context of predictive analytics. Machine learning is frequently used in conjunction with predictive analytics (PA), which uses the insights gleaned from ML to make predictions. The methods to avoid bias in machine learning are as follows:
- Make use of a representative dataset. When it comes to preventing bias in machine learning, feeding your algorithm representative data is the most important aspect.
- Select the appropriate model. Every AI algorithm is unique, and no single model can be used to avoid bias.
- Keep an eye on things and go over them again.
- The Halo Effect-The halo effect (also known as the halo error) is the tendency for positive impressions of a person, company, brand, or product in one area to influence one's opinion or feelings in another. This bias refers to our proclivity to let our perception of a person, company, or business in one domain influence our overall perception of the individual or entity.
For example, a consumer who is pleased with the performance of a microwave purchased from a specific brand seems to be more likely to purchase other products from that brand as a result of their positive experience with the microwave.
- Implicit Bias- The pre-reflective attribution of specific qualities by a person to a representative of some social out-group is known as implicit bias.
Experience is thought to shape implicit stereotypes, which are based on learned associations between specific qualities and social categories such as race and/or gender. Individuals' conceptions and behavioural patterns can be influenced by implicit stereotypes.
- Heuristics Statistical- Individuals use statistical heuristics to deal with different situations, which are judgmental tools that are rough insightful equivalents of statistical principles. Training increases the possibility that people will use statistics to solve a problem as well as the quality of the statistical solutions. According to Tversky and Kahneman, people are frequently biased in their decisions under unpredictability because, in a speed-accuracy tradeoff, they frequently rely on fast and intuitive heuristics with vast margins of error rather than slow calculations based on statistical principles. It is used in performance review statistical analysis.
- Hornes Effect- When "people believe that negative outcomes are linked to each other," the horn effect occurs. It is an occurrence in which an observer's judgement of a person is influenced negatively by the presence of an undesirable aspect of that person (for the observer). This is used in statistical analysis.
- Logical Fallacy- A data scientist's core competency is the ability to transform data effects and patterns into a real-world context. An essential skill for business managers who must analyze data on a regular basis is ensuring that an interpretation is correct and not tainted by one of the many logical fallacies or mental errors that we all make in everyday life. Some fallacies are listed below.
- The cherry-picking fallacy is when you purposefully choose your data or statistics to prove your point, or when you use confirmation bias and motivated reasoning in your analysis rather than a deductive approach.
- The Texas Sharpshooter fallacy involves looking for patterns while ignoring contradictions.
Common Mistakes
- Improper sample selection may lead to faulty outcomes.
- This bias is caused by a flaw in the measurement instrument itself.
- Overfitting is a model that is overly complicated and generates a lot of noise.
Context & Applications
The concept of types of bias is useful in the following fields:
- Scientific research
- Business accounting
- Statistical survey and analysis of data
Related Concepts
- Sensitivity and specificity
- Hypothesis testing
Want more help with your statistics homework?
*Response times may vary by subject and question complexity. Median response time is 34 minutes for paid subscribers and may be longer for promotional offers.
Search. Solve. Succeed!
Study smarter access to millions of step-by step textbook solutions, our Q&A library, and AI powered Math Solver. Plus, you get 30 questions to ask an expert each month.
Types of Bias Homework Questions from Fellow Students
Browse our recently answered Types of Bias homework questions.
Search. Solve. Succeed!
Study smarter access to millions of step-by step textbook solutions, our Q&A library, and AI powered Math Solver. Plus, you get 30 questions to ask an expert each month.