a)
To find: The possibility of the cause of autocorrelation.
a)

Explanation of Solution
The causes of autocorrelation are:
1. Bias in the data
2. The data is not reliable there must be some change in the data.
b)
To find: The effect of autocorrelation.
b)

Explanation of Solution
The results are:
1. two or more independent variables are correlated, i.e., multicollinearity.
2. the function might be sometimes non-linear.
c)
To find: the affect of autocorrelation on the accuracy of
c)

Explanation of Solution
- autocorrelation might underestimate the true variance.
- The null hypothesis might be rejected although it is true.
d)
To find: remedial for autocorrelation removal
d)

Explanation of Solution
The remedy is to increase the number of observations, find the missing values and estimators although linear is not the efficient estimator.
Want to see more full solutions like this?
Chapter 4A Solutions
Bundle: Managerial Economics: Applications, Strategies And Tactics, 14th + Mindtap Economics, 1 Term (6 Months) Printed Access Card
- A multiple OLS regression of maize output (Y) on improved maize seed (X1) and fertiliser (X2) inputs (all variables in kilograms) produced the following results: Y = -342 + 12.1X1 + 46X2 se = (17.23) (0.912) (8.713) R2 = 0.861 a) Calculate the t statistics associated with the constant, improved maize seed and fertiliser coefficientsarrow_forwardSuppose there are 2 quantitative free variables and 1 variable non free category. Non-free variables have 2 categories, namely 1 for the success category and 1 for the fail category. The method used to create models that describe relationships between variables is a binary logistic regression model. Perform parameter recovery for the model. Explain the stage until the alleged value is obtainedarrow_forwardNumerical Answer Only Type Question Enter the numerical value only for the correct answer in the blank box. If a decimal point appears, round it to two decimal places. Assume that the number of visits by a particular customer to a mall located in downtown Toronto is related to the distance from the customer's home. The following regression analysis shows the relationship between the number of times a customer visits(Y)per month and the distance(X, measured in km) from the customer's home to the mall. \[ Y=15-0.5 X \] A customer who lives30 kmaway from the mall will visi______ who lives10 km away. less times than a customerarrow_forward
- This regression is based on cross-section data of 1744 individuals and the relationship between their weekly earnings (in dollars) and age (in years) during 2020. The regression yielded the following result: Estimated(EARN) = 239.16 + 5.20(Age) , R = 0.05, SER = 287.21 Standard errors are reported as hereunder: SE(intercept) = (20.24) SE(Age) = (0.57) (a) Is the relationship between "Age" and "EARN" statistically significant? (b) Explain the meaning of heterskedasticity. Is there any reason to be concerned about heteroskedasticity in this model? Briefly explain your reasons. (c) Construct a 95% confidence interval for the slope coefficient, and use it to test for the statistical significance of the slope coefficient. (d) Construct a 95% confidence interval for the intercept coefficient, and use it to test for the statistical significance of the intercept coefficient.arrow_forwardQUESTION 10 Answer questions 10 to 16 based on the regression outputs given in Table 1& 2. Table 1 DATA4-1: Data on single family homes in University City community of San Diego, in 1990. price - sale price in thousands of dollars (Range 199. 9 505) sqft - square feet of living area (Range 1065 - 3000) Table 2 Model 1: OLS, using observations 1-14 Dependent variable: price coefficient std. error t-ratio p-value 52. 3509 0.138750 37. 2855 0.0187329 0. 1857 8. 20e-06 *** const sqft 7. 407 Me dependent var Sun squared resid R-squared F(1, 12) Log-likelihood Schwarz criterion 317. 4929 18273. 57 0. 820522 54. 86051 -70. 08421 145. 4465 Hannan-Quinn S.D. dependent var S.E. of regression Adjusted R-squared P-value (F) Akaike criterion 88. 49816 39. 02304 0. 805565 8. 20e-06 144. 1684 144. 0501 There are observations included in this dataset. It is a. data. O 12; cross-sectional 13; time-series data 14; cross-sectional In this regression model, sale price of a single-family house is the. the…arrow_forwardRefer to the following computer output from estimating the parameters of the nonlinear model Y=aRbsc7d The computer output from the regression analysis is: DEPENDENT VARIABLE: LNY R-SQUARE 32 0.7766 OBSERVATIONS: VARIABLE INTERCEPT LNR P-VALUE ON F 0.0001 PARAMETER ESTIMATE STANDARD ERROR T-RATIO -0.6931 F-RATIO 4.66 -0.44 8.28 32.44 0.32 1.36 -2.17 3.43 -1.83 P-VALUE 1.80 0.0390 LNS 0.24 LNT 4.60 Based on the information in the table, the nonlinear relation can be transformed into the following linear regression model: Multiple Choice in Y= 1n a.ln R.1n S.1n T in Y= 1na + b1nR+ cins + din T 1n Y = 1n(aRb SC7d) Y = 1n(aRb Sc7d) 0.0019 0.0774 0.0826arrow_forward
- A manufacturer of computer workstations gathered average monthly sales figures from its 56 dealerships across the country and estimated the demand for its product using the following regression equation: Q=B0+ B₁ PRICE + ẞ2 ADV + ẞ3 PRICE C where Q is the number of computer workstations sold monthly, PRICE is the price of the computer workstation, ADV is the advertising expenditures, and PRICE, is the average price of a leading competitor's computer workstation. The regression results are as follows: DEPENDENT VARIABLE: Q PROB(F-STATISTIC) OBSERVATIONS: 56 R-SQUARED 0.68 F-STATISTIC 21.25 Standard 0.04 Variable Coefficient error Intercept 15,000.0 5234.0 PRICE -2.8 1.29 ADV 150.0 175.0 PRICEC 0.2 0.13 a. Which coefficients have a statistically significant effect on the number of workstations sold? Substantiate your answers appropriately. b. Calculate the expected number of workstations sold when PRICE = $7,000, ADV = $52, and PRICE $8,000. c. Calculate the own-price elasticity of…arrow_forwardSuppose the Sherwin-Williams Company is interested in developing a simple regression model with paint sales (Y) as the dependent variable and selling price (P) as the independent variable. Complete the following worksheet and then use it to determine the estimated regression line. Sales Region Selling Price ($/Gallon) Sales (x 1000 Gal) i 2 Zi Yi Zith 1 15 160 2,400 225 25,600 2 13.5 220 2,970 182.25 48,400 3 16.5 140 2,310 272.25 19,600 4 14.5 190 2,755 210.25 36,100 5 17 140 2,380 289 19,600 6 16 160 2,560 256 25,600 7 13 200 2,600 169 40,000 8 18 150 2,700 324 22,500 9 12 220 2,640 144 48,400 10 15.5 190 2,945 240.25 36,100 Total 151 1,770 2,312 Regression Parameters Estimations Slope (B) Intercept (a) In words, for a dollar increase in the selling price, the expected sales will What is the standard error of the estimate (&)? O 14.889 12.180 13.342 gallons in a given sales region. What is the estimate of the standard deviation of the estimated slope (86)? O 2.636 2.157 2.362 Can you…arrow_forwardAn economist believes that price, x, (in dollars) is the biggest factor affecting quantity sold, y. To support his argument, he collected data on price and quantity sold from a sample of 29 stores, selling the same product, and generated the regression output in Excel. The regression equation is reported as y = and the correlation coefficient r = - 0.333. 9.45x + 20.86 What proportion of the variation in quantity sold y can be explained by the variation in price? R² = % Report answer as a percentage accurate to one decimal place.arrow_forward
- In the multiple regression model, if X1 is correlated with μ but the other independent variables are uncorrelated with μ, then all of the OLS estimators are generally consistent. O True ○ Falsearrow_forwardA marketing analyst wants to examine the relationship between sales (in $1,000s) and advertising (in $100s) for firms in the food and beverage industry and collects monthly data for 25 firms. He estimates the modet: Sales- Bo + B1 Advertising +t. The following table shows a portion of the regression results. Coefficients Standard Error t-stat p-value Intercept 40.10 14.08 2.848 0.0052 Advertising 2.88 1.52 -1.895 0.0608 Which of the following are the competing hypotheses used to test whether the slope coefficient differs from 3? Multiple Choice Ho i bị 3; HAtbi3 Họ ib - 2.88; HAibi 2.88arrow_forwardYou are given the following data: The regression equation is: A. -0.66 B. -1.20 (X'X)*¹ C. 1.12 O D. 2.06 = 1.3 2.1 0.8 -1.4 1.9 2.1 -1.4 s² = 0.86. T = 103 The correlation between ₁ and 3 (i.e., corr(Â₁, Â3)) is: -1.6] 1.9 (X'y) = 2.9 3.4 0.8 Yt = B₁ + B₂X2+ + B3X3t + Ut.arrow_forward
- Managerial Economics: Applications, Strategies an...EconomicsISBN:9781305506381Author:James R. McGuigan, R. Charles Moyer, Frederick H.deB. HarrisPublisher:Cengage Learning
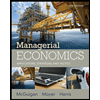