What this particular plot shows about the model being analysed?
Algebra & Trigonometry with Analytic Geometry
13th Edition
ISBN:9781133382119
Author:Swokowski
Publisher:Swokowski
Chapter1: Fundamental Concepts Of Algebra
Section1.1: Real Numbers
Problem 49E
Related questions
Question
The CSV file modeldata.csv contains 200 observations of 4 explanatory variables (x1, x2, x3, x4) and a response variable (y). A multiple linear regression model is built in R using the following code,
> modeldata <- read.csv("modeldata.csv") > x1 <- modeldata$x1 > x2 <- modeldata$x2 > x3 <- modeldata$x3 > x4 <- modeldata$x4 > y <- modeldata$y > model <- lm(y~x1+x2+x3+x4)Question: What this particular plot shows about the model being analysed?
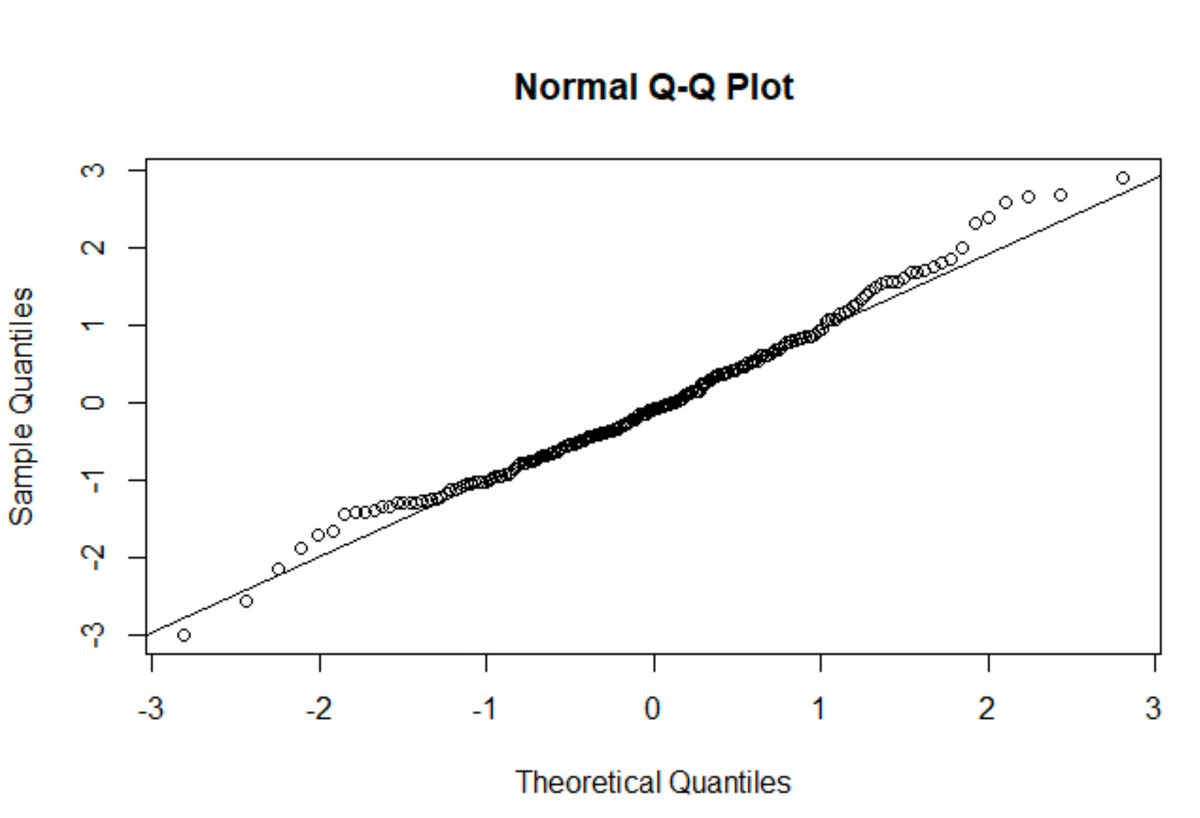
Transcribed Image Text:Sample Quantiles
-3
-2 -1
0 1
-3
2
3
0.00
0000
-2
-1
0
Theoretical Quantiles
Normal Q-Q Plot
00
1
2
3
00
0
0
о
Expert Solution

This question has been solved!
Explore an expertly crafted, step-by-step solution for a thorough understanding of key concepts.
Step by step
Solved in 3 steps

Recommended textbooks for you
Algebra & Trigonometry with Analytic Geometry
Algebra
ISBN:
9781133382119
Author:
Swokowski
Publisher:
Cengage
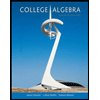
College Algebra
Algebra
ISBN:
9781305115545
Author:
James Stewart, Lothar Redlin, Saleem Watson
Publisher:
Cengage Learning
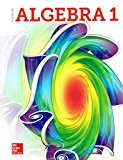
Glencoe Algebra 1, Student Edition, 9780079039897…
Algebra
ISBN:
9780079039897
Author:
Carter
Publisher:
McGraw Hill
Algebra & Trigonometry with Analytic Geometry
Algebra
ISBN:
9781133382119
Author:
Swokowski
Publisher:
Cengage
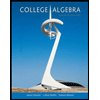
College Algebra
Algebra
ISBN:
9781305115545
Author:
James Stewart, Lothar Redlin, Saleem Watson
Publisher:
Cengage Learning
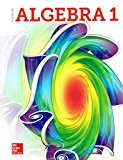
Glencoe Algebra 1, Student Edition, 9780079039897…
Algebra
ISBN:
9780079039897
Author:
Carter
Publisher:
McGraw Hill