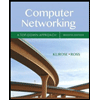
Computer Networking: A Top-Down Approach (7th Edition)
7th Edition
ISBN: 9780133594140
Author: James Kurose, Keith Ross
Publisher: PEARSON
expand_more
expand_more
format_list_bulleted
Question

Transcribed Image Text:We consider a neural network with 100 neurons in the input layer and 10 neurons in
the hidden layer and these two layers are fully connected between, how many weight
parameters do we have between these two layers?
Expert Solution

This question has been solved!
Explore an expertly crafted, step-by-step solution for a thorough understanding of key concepts.
This is a popular solution
Trending nowThis is a popular solution!
Step by stepSolved in 2 steps

Knowledge Booster
Similar questions
- The cost function of a general neural network is defined as J(ŷ,y) = m // [4 (9(0), y(1) The loss function L(ŷ), y() is defined by the logistic loss function Ly, y) = [ylogy) + (1-y)log (1 - ¹)] Please list the stochastic gradient descent update rule, batch gradient descent update rule, and mini-batch gradient descent update rule. Explain the main difference of these three update rules.arrow_forwardConsider the neural network shown below. The network consists of 2 input values (x1, x2) with their associated weights (0.3, 0.4, 0.3) and (0.2, 0.7, 0.1), 3 nodes in the hidden layer (h1, h2, h3) with associated weights (0.3, 0.5, 0.2) for target output. Assume all neurons have the same bias b = 0.2, and the same sigmoid activation function. If we input (x1, x2) = [1, 2], what will be the network’s output?arrow_forwardGiven the flowing neural network, each circle represents a neuron whose thresholdvalue is recorded inside the circle. The lines connecting the circles represent connectionsbetween neurons. Two connected neurons associate the same weight which is recordednext to the line connecting the neurons. What is the output when both inputs are 1s?arrow_forward
- dont post existing answer dont answer without knowledgearrow_forwardConsider the following neural network which takes two binary-valued inputs and outputs. Which logical function does it approximately compute? +1 ... -12 +8 X1 he(x) +8 X2arrow_forwardSuppose we are fitting a neural network with three hidden layers to a training set. It is found that the cross validation error Jcv(0) is much larger than the training error Jtrain (0). Should we increase the number of hidden layers?arrow_forward
- Give an example of how a high number of layers in a neural network might cause a problem.Discuss overfitting and how to prevent it in the following paragraphs.arrow_forwardPlease show the steps to solve as wellarrow_forwardQ1. Consider the neural network in Figure 25.13. Let bias values be fixed at 0, and let the weight matrices between the input and hidden, and hidden and output layers, respectively, be: W = (w1, w2, w3) = (1, 1, –1) W = (w, w½, wý)™ = (0.5, 1, 2)" Assume that the hidden layer uses ReLU, whereas the output layer uses sigmoid activation. Assume SSE error. Answer the following questions, when the input is x = 4 and the true response is y = 0: z1 wi w2 22 ws 23 Figure 25.13. Neural network for Q1. (a) Use forward propagation to compute the predicted output. (b) What is the loss or error value? (c) Compute the net gradient vector 8º for the output layer. (d) Compute the net gradient vector &ª for the hidden layer.arrow_forward
- Question 16 Joe designed two neural networks: NN1 and NN2. Both networks have a softmax output layer with three classes and a cross-entropy loss. He feeds an input from class 1 to each network. The output of NN1 is 0.41, 0.37, and 0.22 for classes 1, 2, and 3, respectively. The output of NN2 is 0.74, 0.11, and 0.15 for classes 1, 2, and 3, respectively. Calculate the loss of each network and report it for NN1 and NN2 Based on these findings, (NN1 or NN2) is a better design.arrow_forwardPlease provide an illustration of how a neural network that has an excessive number of layers could result in an issue.Overfitting will be discussed, along with ways to prevent it, in the following paragraphs.arrow_forwardShow the output for each node during the forward pass. The output of C is (round to 3 decimals): The output of D is (round to 3 decimals):arrow_forward
arrow_back_ios
SEE MORE QUESTIONS
arrow_forward_ios
Recommended textbooks for you
- Computer Networking: A Top-Down Approach (7th Edi...Computer EngineeringISBN:9780133594140Author:James Kurose, Keith RossPublisher:PEARSONComputer Organization and Design MIPS Edition, Fi...Computer EngineeringISBN:9780124077263Author:David A. Patterson, John L. HennessyPublisher:Elsevier ScienceNetwork+ Guide to Networks (MindTap Course List)Computer EngineeringISBN:9781337569330Author:Jill West, Tamara Dean, Jean AndrewsPublisher:Cengage Learning
- Concepts of Database ManagementComputer EngineeringISBN:9781337093422Author:Joy L. Starks, Philip J. Pratt, Mary Z. LastPublisher:Cengage LearningPrelude to ProgrammingComputer EngineeringISBN:9780133750423Author:VENIT, StewartPublisher:Pearson EducationSc Business Data Communications and Networking, T...Computer EngineeringISBN:9781119368830Author:FITZGERALDPublisher:WILEY
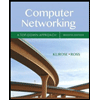
Computer Networking: A Top-Down Approach (7th Edi...
Computer Engineering
ISBN:9780133594140
Author:James Kurose, Keith Ross
Publisher:PEARSON
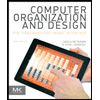
Computer Organization and Design MIPS Edition, Fi...
Computer Engineering
ISBN:9780124077263
Author:David A. Patterson, John L. Hennessy
Publisher:Elsevier Science
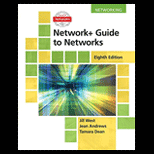
Network+ Guide to Networks (MindTap Course List)
Computer Engineering
ISBN:9781337569330
Author:Jill West, Tamara Dean, Jean Andrews
Publisher:Cengage Learning
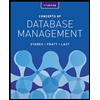
Concepts of Database Management
Computer Engineering
ISBN:9781337093422
Author:Joy L. Starks, Philip J. Pratt, Mary Z. Last
Publisher:Cengage Learning
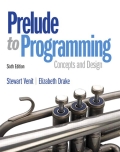
Prelude to Programming
Computer Engineering
ISBN:9780133750423
Author:VENIT, Stewart
Publisher:Pearson Education
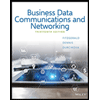
Sc Business Data Communications and Networking, T...
Computer Engineering
ISBN:9781119368830
Author:FITZGERALD
Publisher:WILEY