The Simple Linear Regression model is Y = b0 + b1*X1 + u and the Multiple Linear Regression model with k variables is: Y = b0 + b1*X1 + b2*X2 + ... + bk*Xk + u Y is the dependent variable, the X1, X2, ..., Xk are the explanatory variables, b0 is the intercept, b1, b2, ..., bk are the slope coefficients, and u is the error term, Yhat represents the OLS fitted values, uhat represent the OLS residuals, b0_hat represents the OLS estimated intercept, and b1_hat, b2_hat,..., bk_hat, represent the OLS estimated slope coefficients. QUESTION 7 In the MLR model, the assumption of ‘linearity in parameters’ is violated if: one of the slope coefficients appears as a power (e.g. Y = b0 + b1*(X1^b2) + b3*X2 + u) the model includes the reciprocal of a variable (e.g. 1/X1) the model includes a variable squared (e.g. X1^2) the model includes a variable in its logarithmic form (i.e. log(X1) ) QUESTION 8 In the MLR model, the assumption of 'no perfect collinearity' is violated if: the model includes two variables that are not correlated the model includes both X1 and X1^2 (i.e. X1-squared) if two of the explanatory variables have a Pearson correlation equal to 0.98 if two of the explanatory variables have a Pearson correlation equal to -1 QUESTION 9 Suppose the estimated MLR model is, Yhat = 2 + 1.5*X1 + 0.5*X2 + 2*X3. Suppose that for an observation with X1=2, X2=-2, X3=5, we observe an actual value in the sample of Y=10. What is the residual, uhat, for this observation? negative 4 negative 14 positive 4 positive 14
The Simple Linear Regression model is Y = b0 + b1*X1 + u and the Multiple Linear Regression model with k variables is: Y = b0 + b1*X1 + b2*X2 + ... + bk*Xk + u Y is the dependent variable, the X1, X2, ..., Xk are the explanatory variables, b0 is the intercept, b1, b2, ..., bk are the slope coefficients, and u is the error term, Yhat represents the OLS fitted values, uhat represent the OLS residuals, b0_hat represents the OLS estimated intercept, and b1_hat, b2_hat,..., bk_hat, represent the OLS estimated slope coefficients. QUESTION 7 In the MLR model, the assumption of ‘linearity in parameters’ is violated if: one of the slope coefficients appears as a power (e.g. Y = b0 + b1*(X1^b2) + b3*X2 + u) the model includes the reciprocal of a variable (e.g. 1/X1) the model includes a variable squared (e.g. X1^2) the model includes a variable in its logarithmic form (i.e. log(X1) ) QUESTION 8 In the MLR model, the assumption of 'no perfect collinearity' is violated if: the model includes two variables that are not correlated the model includes both X1 and X1^2 (i.e. X1-squared) if two of the explanatory variables have a Pearson correlation equal to 0.98 if two of the explanatory variables have a Pearson correlation equal to -1 QUESTION 9 Suppose the estimated MLR model is, Yhat = 2 + 1.5*X1 + 0.5*X2 + 2*X3. Suppose that for an observation with X1=2, X2=-2, X3=5, we observe an actual value in the sample of Y=10. What is the residual, uhat, for this observation? negative 4 negative 14 positive 4 positive 14
MATLAB: An Introduction with Applications
6th Edition
ISBN:9781119256830
Author:Amos Gilat
Publisher:Amos Gilat
Chapter1: Starting With Matlab
Section: Chapter Questions
Problem 1P
Related questions
Question
The Simple Linear Regression model is
Y = b0 + b1*X1 + u
and the Multiple Linear Regression model with k variables is:
Y = b0 + b1*X1 + b2*X2 + ... + bk*Xk + u
Y is the dependent variable, the X1, X2, ..., Xk are the explanatory variables, b0 is the intercept, b1, b2, ..., bk are the slope coefficients, and u is the error term,
Yhat represents the OLS fitted values, uhat represent the OLS residuals, b0_hat represents the OLS estimated intercept, and b1_hat, b2_hat,..., bk_hat, represent the OLS estimated slope coefficients.
QUESTION 7
In the MLR model, the assumption of ‘linearity in parameters’ is violated if:
- one of the slope coefficients appears as a power (e.g. Y = b0 + b1*(X1^b2) + b3*X2 + u)
- the model includes the reciprocal of a variable (e.g. 1/X1)
- the model includes a variable squared (e.g. X1^2)
- the model includes a variable in its logarithmic form (i.e. log(X1) )
QUESTION 8
In the MLR model, the assumption of 'no perfect collinearity' is violated if:
- the model includes two variables that are not correlated
- the model includes both X1 and X1^2 (i.e. X1-squared)
- if two of the explanatory variables have a Pearson
correlation equal to 0.98 - if two of the explanatory variables have a Pearson correlation equal to -1
QUESTION 9
Suppose the estimated MLR model is, Yhat = 2 + 1.5*X1 + 0.5*X2 + 2*X3.
Suppose that for an observation with X1=2, X2=-2, X3=5, we observe an actual value in the sample of Y=10. What is the residual, uhat, for this observation?
- negative 4
- negative 14
- positive 4
- positive 14
Expert Solution

This question has been solved!
Explore an expertly crafted, step-by-step solution for a thorough understanding of key concepts.
Step by step
Solved in 3 steps with 3 images

Follow-up Questions
Read through expert solutions to related follow-up questions below.
Follow-up Question
So for question 7 and 8 are the answers options: 2 and 1?
Solution
Recommended textbooks for you
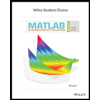
MATLAB: An Introduction with Applications
Statistics
ISBN:
9781119256830
Author:
Amos Gilat
Publisher:
John Wiley & Sons Inc
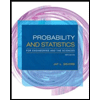
Probability and Statistics for Engineering and th…
Statistics
ISBN:
9781305251809
Author:
Jay L. Devore
Publisher:
Cengage Learning
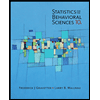
Statistics for The Behavioral Sciences (MindTap C…
Statistics
ISBN:
9781305504912
Author:
Frederick J Gravetter, Larry B. Wallnau
Publisher:
Cengage Learning
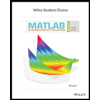
MATLAB: An Introduction with Applications
Statistics
ISBN:
9781119256830
Author:
Amos Gilat
Publisher:
John Wiley & Sons Inc
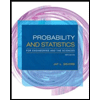
Probability and Statistics for Engineering and th…
Statistics
ISBN:
9781305251809
Author:
Jay L. Devore
Publisher:
Cengage Learning
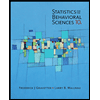
Statistics for The Behavioral Sciences (MindTap C…
Statistics
ISBN:
9781305504912
Author:
Frederick J Gravetter, Larry B. Wallnau
Publisher:
Cengage Learning
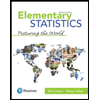
Elementary Statistics: Picturing the World (7th E…
Statistics
ISBN:
9780134683416
Author:
Ron Larson, Betsy Farber
Publisher:
PEARSON
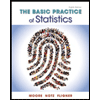
The Basic Practice of Statistics
Statistics
ISBN:
9781319042578
Author:
David S. Moore, William I. Notz, Michael A. Fligner
Publisher:
W. H. Freeman
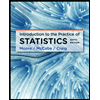
Introduction to the Practice of Statistics
Statistics
ISBN:
9781319013387
Author:
David S. Moore, George P. McCabe, Bruce A. Craig
Publisher:
W. H. Freeman