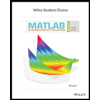
Concept explainers
Demand Estimation for The San Francisco Bread Company
Consider the hypothetical example of The San Francisco Bread Company, a San Francisco-based chain of bakery/cafes. San Francisco Bread Company has initiated an empirical estimation of customer traffic at 30 regional locations to help the firm formulate pricing and promotional plans for the coming year. Annual operating data for the 30 outlets appear in the attached Table 1.
The following regression equation was fit to these data:
Qi = b0 + b1Pi + b2Pxi + b3Adi + b4Ii + uit.
Where: Q is the number of meals served,
P is the average price per meal (customer ticket amount, in dollars),
Px is the average price charged by competitors (in dollars),
Ad is the local advertising budget for each outlet (in dollars),
I is the average income per household in each outlet’s service area,
ui is a residual (or disturbance) term.
The subscript indicates the regional market (i = 1,…, 30) from which the observation was taken. Least squares estimation of the regression equation on the basis of the 30 data cross sectional observations resulted in the estimated regression coefficients and other statistics as shown in Table 2.
F. Conduct a F-test for the set of coefficients in the equation to determine if they are significant at the 95 and 99 percent levels.
(See Below for Data)
Table 1 - San Francisco Bread Company (30 Markets)
Market Demand Price Competitor Advertising Income
Market (Q) (P) Price(Px) (Ad) (I)
1 596,611 7.62 6.52 200,259 54,880
2 596,453 7.29 5.01 204,559 51,755
3 599,201 6.66 5.96 206,647 52,955
4 572,258 8.01 5.30 207,025 54,391
5 558,142 7.53 6.16 207,422 48,491
6 627,973 6.51 7.56 216,224 51,219
7 593,024 6.20 7.15 217,954 48,685
8 565,004 7.28 6.97 220,139 47,219
9 596,254 5.96 5.52 220,215 49,755
10 652,880 6.42 6.27 220,728 54,932
11 596,784 5.94 5.66 226,603 48,092
12 657,468 6.47 7.68 228,620 54,929
13 519,886 6.99 5.10 230,241 46,057
14 612,941 7.72 5.38 232,777 55,239
15 621,707 6.46 6.20 237,300 53,976
16 597,215 7.31 7.43 238,756 49,576
17 617,427 7.36 5.28 241,957 55,454
18 572,320 6.19 6.12 251,317 48,480
19 602,400 7.95 6.38 254,393 53,249
20 575,004 6.34 5.67 255,699 49,696
21 667,581 5.54 7.08 262,270 52,600
22 569,880 7.89 5.10 275,588 50,472
23 644,684 6.76 7.22 277,667 53,409
24 605,468 6.39 5.21 277,816 52,660
25 599,213 6.42 6.00 279,031 50,464
26 610,735 6.82 6.97 279,934 49,525
27 603,830 7.10 5.30 287,921 49,489
28 617,803 7.77 6.96 289,358 49,375
29 529,009 8.07 5.76 294,787 48,254
30 573,211 6.91 5.96 296,246 46,017
Mean 598,412 6.93 6.16 244,649 51,044
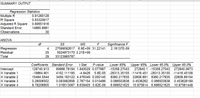
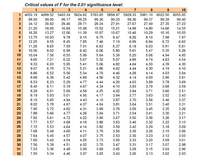

Trending nowThis is a popular solution!
Step by stepSolved in 2 steps

- This is only one questionarrow_forwardConsumers are often interested in the fuel efficiency of the vehicles they choose to buy, so much so that they will research the various models they consider buying. Fuel efficiency can depend on a variety of variables. In this analysis, there are 73 automobiles that are popular with consumers. A regression analysis has been performed; the dependent variable is CityMPG (EPA miles per gallon in city driving), and independent variables are Length (vehicle length in inches), Width (vehicle width in inches), Weight (vehicle weight in pounds), and ManTran (1 if manual shift transmission, 0 otherwise). The level of significance is 0.05. Use the following MegaStat output to answer questions about this regression analysis. a. State the regression equation. b. How would CityMPG be affected if the width of a vehicle increased by an inch? c. Estimate the CityMPG for a vehicle with a length of 190 inches, a width of 75 inches, a weight of 4100 pounds, and a manual. Round your answer to the nearest…arrow_forwardThe manager of the Bayville police department motor pool wants to develop a forecast model for annual maintenance on police cars, based on mileage in the past year and age of the cars. The following data have been collected for eight different cars: a. Using Excel, develop a multiple regression equation for these data. b. What is the coefficient of determination for this regression equation? c. Forecast the annual maintenance cost for a police car that is 5 years old and will be driven 10,000 miles in 1 year.arrow_forward
- Hiroshi Sato, an owner of a sushi restaurant in San Francisco, has been following an aggressive marketing campaign to thwart the effect of rising unemployment rates on business. He used monthly data on sales ($1,000s), advertising costs ($), and the unemployment rate (%) fromJanuary 2008 to May 2009 to estimate the following sample regression equation: Sales(t) = 17.51 +0.05 Advertising Costs(t-1) – 0.70 Unemployment Rate t-1 Requirement: a. Hiroshi had budgeted $620 toward advertising costs in May 2009. Make a forecast in June2009, if the unemployment rate in May 2009 was 9.1%b. What will be the forecast if he raises his advertisement budget to $700?c. Reevaluate the above forecast if the unemployment rate in May 2009 was 9.5%. Please, if possible, can you do the answer in Excel/Spreadsheetarrow_forwardUse the Financial database from “Excel Databases.xls” on Blackboard. Use Total Revenues, Total Assets, Return on Equity, Earnings Per Share, Average Yield, and Dividends Per Share to predict the average P/E ratio for a company. Use Excel to develop the multiple linear regression model. Assume a 5% level of significance. Which independent variable is the strongest predictor of the average P/E ratio of a company? A. Total Revenues B. Average Yield C. Earnings Per Share D.Return on Equity E. Total Assets F.Dividends Per Share Company Type Total Revenues Total Assets Return on Equity Earnings per Share Average Yield Dividends per Share Average P/E Ratio AFLAC 6 7251 29454 17.1 2.08 0.9 0.22 11.5 Albertson's 4 14690 5219 21.4 2.08 1.6 0.63 19 Allstate 6 20106 80918 20.1 3.56 1 0.36 10.6 Amerada Hess 7 8340 7935 0.2 0.08 1.1 0.6 698.3 American General 6 3362 80620 7.1 2.19 3 1.4 21.2 American Stores 4 19139 8536 12.2 1.01 1.4 0.34 23.5 Amoco 7 36287…arrow_forwardAccording to an article, one may be able to predict an individual's level of support for ecology based on demographic and ideological characteristics. The multiple regression model proposed by the authors was the following. y = 3.60-.01x₁+.01.₂-.07x3+.12x4+.02xs-.04x6-01-.04.xg-.02.xg+c The variables are defined as follows. y = ecology score (higher values indicate a greater concern for ecology) X₁ = age times 10 x₂ = income (in thousands of dollars) x3 = gender (1 = male, 0 = female) X4 = race (1 = white, 0 = nonwhite) X5 = education (in years) x6 = ideology (4 = conservative, 3 = right of center, 2 = middle of the road, 1 = left of center, and 0 = liberal) X7 = social class (4 = upper, 3 = upper middle, 2 = middle, 1 = lower middle, 0 = lower) xg = postmaterialist (1 if postmaterialist, 0 otherwise) x9 = materialist (1 if materialist, 0 otherwise) (a) Suppose you knew a person with the following characteristics: a 30 year old, white female with a college degree (20 years of…arrow_forward
- Gasoline Mileage. An engineer wants to determine factors that affect the EPA miles per gallon rating of new automobiles available for sale in the United States. a. Suggest five predictor variables that might be useful in predicting the miles per gallon rating. b. Write the multiple linear regression model for the conditional mean of the response variable, miles per gallon.arrow_forwardWe have data from 209 publicly traded companies (circa 2010) indicating sales and compensation information at the firm-level. We are interested in predicting a company's sales based on the CEO's salary. The variable sales; represents firm i's annual sales in millions of dollars. The variable salary; represents the salary of a firm i's CEO in thousands of dollars. We use least-squares to estimate the linear regression sales; = a + ßsalary; + ei and get the following regression results: . regress sales salary Source Model Residual Total sales salary cons SS 337920405 2.3180e+10 2.3518e+10 df 1 207 208 Coef. Std. Err. .9287785 .5346574 5733.917 1002.477 MS 337920405 111980203 113066454 Number of obs F (1, 207) Prob > F R-squared t P>|t| = Adj R-squared = Root MSE 1.74 0.084 5.72 0.000 = = -.1252934 3757.543 = 209 3.02 0.0838 0.0144 0.0096 10582 [95% Conf. Interval] 1.98285 7710.291 This output tells us the regression line equation is sales = 5,733.917 +0.9287785 salary. Interpret the…arrow_forward
- MATLAB: An Introduction with ApplicationsStatisticsISBN:9781119256830Author:Amos GilatPublisher:John Wiley & Sons IncProbability and Statistics for Engineering and th...StatisticsISBN:9781305251809Author:Jay L. DevorePublisher:Cengage LearningStatistics for The Behavioral Sciences (MindTap C...StatisticsISBN:9781305504912Author:Frederick J Gravetter, Larry B. WallnauPublisher:Cengage Learning
- Elementary Statistics: Picturing the World (7th E...StatisticsISBN:9780134683416Author:Ron Larson, Betsy FarberPublisher:PEARSONThe Basic Practice of StatisticsStatisticsISBN:9781319042578Author:David S. Moore, William I. Notz, Michael A. FlignerPublisher:W. H. FreemanIntroduction to the Practice of StatisticsStatisticsISBN:9781319013387Author:David S. Moore, George P. McCabe, Bruce A. CraigPublisher:W. H. Freeman
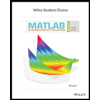
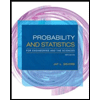
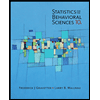
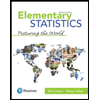
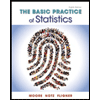
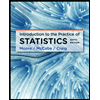