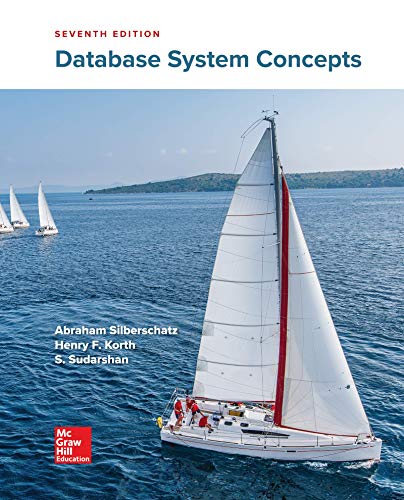
def edge_highlight(self, image):
"""
Returns a new image with the edges highlighted
# Check output
>>> img_proc = PremiumImageProcessing()
>>> img = img_read_helper('img/test_image_32x32.png')
>>> img_edge = img_proc.edge_highlight(img)
>>> img_exp = img_read_helper('img/exp/test_image_32x32_edge.png')
>>> img_exp.pixels == img_edge.pixels # Check edge_highlight output
True
>>> img_save_helper('img/out/test_image_32x32_edge.png', img_edge)
"""
# YOUR CODE GOES HERE #
kernel = [[-1, -2, -1],
[0, 0, 0],
[1, 2, 1]
]
pixels = image.pixels
new_pixels = []
for y in range(len(pixels)):
new_row = []
for x in range(len(pixels[y])):
neighbors = []
for dy in range(-1, 2):
for dx in range(-1, 2):
nx = x + dx
ny = y + dy
if 0 <= nx < len(pixels[y]) and 0 <= ny < len(pixels):
neighbors.append(pixels[ny][nx])
new_r = sum([neighbors[i][j][0] * kernel[i][j] for i in range(3) for j in range(3)])
new_g = sum([neighbors[i][j][1] * kernel[i][j] for i in range(3) for j in range(3)])
new_b = sum([neighbors[i][j][2] * kernel[i][j] for i in range(3) for j in range(3)])
new_r = min(255, max(0, new_r))
new_g = min(255, max(0, new_g))
new_b = min(255, max(0, new_b))
new_row.append([new_r, new_g, new_b])
new_pixels.append(new_row)
return RGBImage(new_pixels)
A method that highlights the edges in an image.
To highlight edges, we will want to ignore all color data. Therefore, your first step should be to convert all pixels into single values.
For the following RGB Image
[
[[215, 209, 223], [108, 185, 135], [ 54, 135, 36]],
[[184, 20, 245], [ 32, 52, 249], [243, 155, 96]],
[[108, 66, 116], [ 65, 200, 34], [ 2, 213, 238]]
]
This would become (use floor division when calculating average)
[
[215, 142, 75],
[149, 111, 164],
[ 96, 99, 151]
]
The next step is to apply something called a kernel. It is a type of 2D array that acts like a mask, being applied to every pixel. It looks like this
[
[-1, -1, -1],
[-1, 8, -1],
[-1, -1, -1]
]
To calculate the output for the pixel at row=0, col=0, you multiply all of the mask’s values with all of the pixel values, then add them together.
Since the mask is centered at the top left, you can ignore the weights outside of the image. The colored values are the relevant weights that we will use for this single operation.
[
[-1, -1, -1],
[-1, 8, -1],
[-1, -1, -1]
]
Then, centered at 0,0 we multiply each weight by the relevant value
[
[215 * 8, 142 * -1, …],
[149 * -1, 111 * -1, …],
[ …, …, …]
]
masked_value = 215*8 + 142*-1 + 149*-1 + 111*-1 = 1318 -> 255
Note that the masked_value needs to be between 0 and 255 in your code.
Next, we will show the calculation for the value at row=1, col=1. This will use the full mask.
[
[215 * -1, 142 * -1, 75 * -1],
[149 * -1, 111 * 8, 164 * -1],
[ 96 * -1, 99 * -1, 151 * -1]
]
masked_value = 215*-1 + 142*-1 + 75*-1 + 149*-1 + 111*8 + 164*-1 + 96*-1 + 99*-1 + 151*-1 = -203 -> 0
This is what the two calculations we have done would look like in the output matrix. Note that it is certainly possible to have values between 0 and 255 in the output, but that these examples did not yield that.
[
[255, …, …],
[ …, 0, …],
[ …, …, …]
]
Finally, you will want to convert this into an RGBImage object. Simply use the single intensity value for all 3 channels.
[
[[255,255,255], …, …],
[ …, [ 0, 0, 0], …],
[ …, …, …]
]
This process of applying a mask to all of the pixels in an image is called convolution, and is the technique that Convolutional Neural Networks use.
no imports

Step by stepSolved in 2 steps

- body { font-family: Georgia, serif; font-size: 100%; line-height: 175%; margin: 0 15% 0; background-color:rgb(210,220,157); background-image: url("/Users/332bo/Desktop/Week_9_Lab/images/bullseye.png"); /* Rounded Shape image */ /* background-repeat:round space; */opacity: 0.5; background-size: contain; } header { margin-top: 0; padding: 3em 1em 2em 1em; text-align: center; border-radius:4px; background-color:hsl(0, 14%, 95%); background-image: url("/Users/332bo/Desktop/Week_9_Lab/images/purpledot.png"); background-repeat: repeat-x; } a { text-decoration: none; color:rgb(153,51,153); } a:visited { color: hsl(300, 13%, 51%); } a:hover { background-color: #fff; } a:focus { background-color: #fff; } a:active{color:#ff00ff;} h1 { font: bold 1.5em Georgia, serif; text-shadow: 0.1em 0.1em 0.2em gray; color: rgb(153,51,153);} h2 { font-size: 1em; text-transform: uppercase; letter-spacing: 0.5em; text-align: center; color:rgb(204,102,0); }dt { font-weight: bold; } strong { font-style: italic; }…arrow_forwardYou can also download the image file from https://www.dropbox.com/scl/fi/ztmuke5mlonrb3e0jdpuj/Santa.jpg?rlkey=lfdzstv649h141jolqdh2kagv&dl=0(1) Write a code to change all red color pixels into blue.(2) Write a code to change only the right half of the red hat into blue, using two ifarrow_forwardJS-> // selecting required elementconst element = document.querySelector(".pagination ul");let totalPages = 10;let page = 2; //calling function with passing parameters and adding inside element which is ul tagelement.innerHTML = createPagination(totalPages, page);function createPagination(totalPages, page){ let liTag = ''; let active; let beforePage = page - 1; let afterPage = page + 1; if(page > 1){ //show the next button if the page value is greater than 1 liTag += <liclass=btn prevonclick=createPagination(totalPages, ${page - 1})><span><iclass=fas fa-angle-left><i>Prev<span><li>; } // how many pages or li show before the current li if (page == totalPages) { beforePage = beforePage - 2; } else if (page == totalPages - 1) { beforePage = beforePage - 1; } // how many pages or li show after the current li if (page == 1) { afterPage = afterPage + 2; } else if (page == 2) { afterPage = afterPage + 1; } for (var…arrow_forward
- The arcpy.mapping module can do all of the following except: O Create a new mxd from scratch Save a copy of a mxd Update text elements on a map layout Repair data sourcesarrow_forwardWhat function is required after you have made changes to the pixels[] array. updatePixels() loadPixels() accessPixels() createPixels()arrow_forwardEXPERIMENT: Watching the Image Loader using e global flags to enable a debugging feature Write all steps of experiement.arrow_forward
- Create empty images for Ix, Iy, Ixx, Iyy, and Ixy (all the same dimensions as your original image) Loop through pixels of image and fill in values for Ix, Iy, Ixx, Iyy, and Ixy (ignore the edges when you set up the range of your loops) Create empty image to hold "cornerness" values Loop through pixels of image Access the a neighborhood surrounding each pixel (start with a 3x3, you can expand it later if you'd like) Sum up all the Ixx, Iyy, and Ixy values in this neighborhood Compute the determinant of the M matrix Compute the trace of the M matrix Compute the "cornerness" value for the center pixel - store in the "cornerness" image find max cornerness value in imageset threshold = 0.01(max C value) loop through pixels. if(C at pixel) > threshold: cv2.circle(image(X,Y),size(b,g,r) - 1) Do may not make use of any existing functions/code that extract features. Use opencv and python. Also don't use sobel.arrow_forwardHow do i add a transfer page code to the line of code below? <ul class="nav nav-pills mb-3" id="pills-tab" role="tablist"> <li class="nav-item" role="presentation"> <button class="nav-link active" id="pills-home-tab" data-bs-toggle="pill" data-bs-target="#pills-home" type="button" role="tab" aria-controls="pills-home" aria-selected="true">Account to Account</button> </li> <li class="nav-item" role="presentation"> <button class="nav-link" id="pills-profile-tab" data-bs-toggle="pill" data-bs-target="#pills-profile" type="button" role="tab" aria-controls="pills-profile" aria-selected="false">External Transfer</button> </li> <li class="nav-item" role="presentation"> <button class="nav-link" id="pills-contact-tab" data-bs-toggle="pill" data-bs-target="#pills-contact" type="button" role="tab" aria-controls="pills-contact" aria-selected="false">Recurring Transfer</button>arrow_forward.arrow_forward
- Find the body:def get_line(board: str, dir: str, col_or_row: int) -> str: """Return the characters that make up the specified row, column, or diagonal from the given tic-tac-toe game board. >>> get_line(4, across, 2) XX >>> get_line(9, down, 3) OOO >>> get_line(4, down_diagonal) OO """arrow_forwardPlease help me create a jframe with clickable image icons (with words at the bottom of the icons) and a search bar at the top. Please include the logo at the top. Please use java and comment the code. Images are provided belowarrow_forwardAdjust the parameter. Image size is unsupported. Each image dimension must utilize 40–2600 pixels.arrow_forward
- Database System ConceptsComputer ScienceISBN:9780078022159Author:Abraham Silberschatz Professor, Henry F. Korth, S. SudarshanPublisher:McGraw-Hill EducationStarting Out with Python (4th Edition)Computer ScienceISBN:9780134444321Author:Tony GaddisPublisher:PEARSONDigital Fundamentals (11th Edition)Computer ScienceISBN:9780132737968Author:Thomas L. FloydPublisher:PEARSON
- C How to Program (8th Edition)Computer ScienceISBN:9780133976892Author:Paul J. Deitel, Harvey DeitelPublisher:PEARSONDatabase Systems: Design, Implementation, & Manag...Computer ScienceISBN:9781337627900Author:Carlos Coronel, Steven MorrisPublisher:Cengage LearningProgrammable Logic ControllersComputer ScienceISBN:9780073373843Author:Frank D. PetruzellaPublisher:McGraw-Hill Education
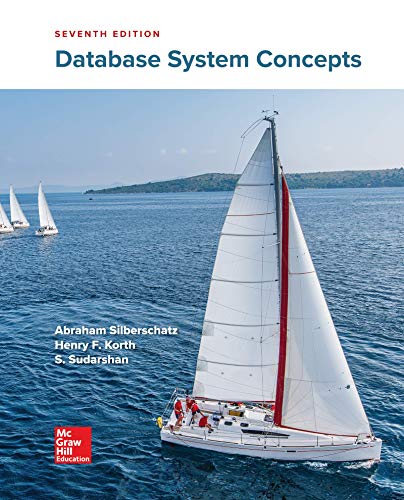
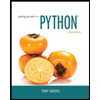
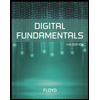
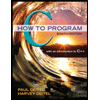
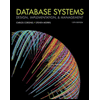
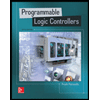