astfoodStats Assignment Description For this assignment, name your R file fastfoodStats.R For all questions you should load tidyverse, openintro, and lm.beta. You should not need to use any other libraries. suppressPackageStartupMessages(library(tidyverse)) suppressPackageStartupMessages(library(openintro)) suppressPackageStartupMessages(library(lm.beta)) The actual data set is called fastfood. Continue to use %>% for the pipe. CodeGrade does not support the new pipe. Round all float/dbl values to two decimal places. All statistics should be run with variables in the order I state E.g., "Run a regression predicting mileage from mpg, make, and type" would be: lm(mileage ~ mpg + make + type...) To access the fastfood data, run the following: fastfood <- openintro::fastfood Create a correlation matrix for the relations between calories, total_fat, sugar, and calcium for all items at Sonic, Subway, and Taco Bell, omitting missing values with na.omit(). Assign the matrix to Q1. The output should look like this: calories total_fat sugar calcium calories 1.00 0.81 0.45 0.61 total_fat 0.81 1.00 0.10 0.24 sugar 0.45 0.10 1.00 0.67 calcium 0.61 0.24 0.67 1.00
astfoodStats Assignment Description
For this assignment, name your R file fastfoodStats.R
- For all questions you should load tidyverse, openintro, and lm.beta. You should not need to use any other libraries.
suppressPackageStartupMessages(library(tidyverse))
suppressPackageStartupMessages(library(openintro))
suppressPackageStartupMessages(library(lm.beta))
The actual data set is called fastfood.
- Continue to use %>% for the pipe. CodeGrade does not support the new pipe.
- Round all float/dbl values to two decimal places.
- All statistics should be run with variables in the order I state
- E.g., "Run a regression predicting mileage from mpg, make, and type" would be:
lm(mileage ~ mpg + make + type...)
To access the fastfood data, run the following:
fastfood <- openintro::fastfood |
- Create a correlation matrix for the relations between calories, total_fat, sugar, and calcium for all items at Sonic, Subway, and Taco Bell, omitting missing values with na.omit().
- Assign the matrix to Q1. The output should look like this:
calories total_fat sugar calcium calories 1.00 0.81 0.45 0.61 total_fat 0.81 1.00 0.10 0.24 sugar 0.45 0.10 1.00 0.67 calcium 0.61 0.24 0.67 1.00 |

Introduction
Co-relational matrix:
A correlation matrix is a matrix that depicts the linear relation of a number of variables. Pearson's correlation, which quantifies the sequential association between two variables, is displayed by the submissions inside the mixture. Pearson's correlations can vary from -1 to 1, with -1 representing a perfect negative linear relationship, 1 representing a perfect positive linear relationship, and 0 representing no linear relationship. In exploratory data analysis, the correlation matrix is frequently used to identify and visualize relationships between variables.
Step by step
Solved in 3 steps

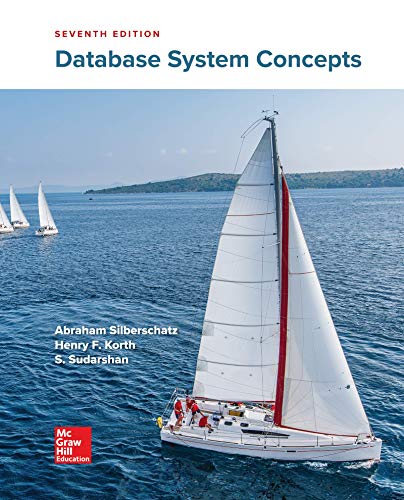
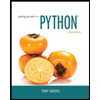
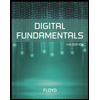
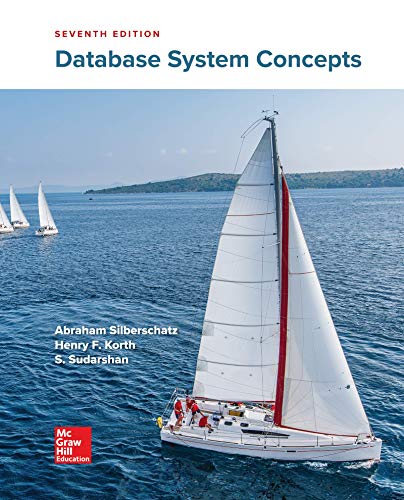
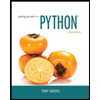
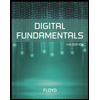
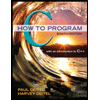
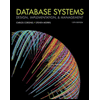
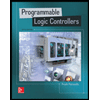