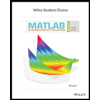
Concept explainers
A Simple Linear Regression (SLR) was performed where the monthly Revenue ("Rev", the y-variable) was regressed on the monthly Advertising Expenditures ("Expend", the x-variable). Excel was used to construct the 98% Confidence
ANOVA
df | SS | MS | F | Significance F | |
Regression | 1 | 492.528125 | 492.528125 | 10.65525634 | 0.046980871 |
Residual | 3 | 138.671875 | 46.22395833 | ||
Total | 4 | 631.2 |
Coefficients | Standard Error | t Stat | P-value | Lower 95% | Upper 95% | Lower 98.0% | Upper 98.0% | |
Intercept | 23.1328125 | 5.324310936 | 4.344752359 | 0.022510469 | 6.188478833 | 40.07714617 | -1.043301388 | 47.30892639 |
Expend | 3.1015625 | 0.950164031 | 3.264239014 | 0.046980871 | 0.077716489 | 6.125408511 | -1.212850033 | 7.415975033 |
a. Enter the value of the Left-Hand Endpoint (LHEP) of the 98% Confidence Interval (CI) estimate of beta subscript 1. Round off your answer to the fourth decimal place.
The LHEP of the 98% CI for beta subscript 1, rounded off as instructed, is: Blank 1. Fill in the blank, read surrounding text.
b. Enter the value of the Right-Hand Endpoint (RHEP) of the 98% Confidence Interval (CI) estimate of beta subscript 1. Round off your answer to the fourth decimal place.
The RHEP of the 98% CI for beta subscript 1, rounded off as instructed, is: Blank 2. Fill in the blank, read surrounding text.
c. Select the number of the following statement that gives the correct interpretation of the CI in this problem:
1: the CI is a NEGATIVE CI, and therefore REV and EXPEND are NOT significantly Linearly related.
2: The CI is a POSITIVE CI, and therefore REV and EXPEND are NOT significantly Linearly related.
3: The CI is a MIXED CI, and therefore REV and EXPEND are NOT significantly Linearly related.
4: The CI is a NEGATIVE CI, and therefore REV and EXPEND ARE significantly Negatively Linearly related.
5: The CI is a POSITIVE CI, and therefore REV and EXPEND ARE significantly Positively Linearly related.
Answer to part (c):

Step by stepSolved in 2 steps

- What mean physical health score would you expect in a group of 28-year-old women with a graduate degree?arrow_forwardListed below are altitudes (thousands of feet) and outside air temperatures (°F) recorded during a flight. Find the (a) explained variation, (b) unexplained variation, and (c) indicated prediction interval. There is sufficient evidence to support a claim of a linear correlation, so it is reasonable to use the regression equation when making predictions. For the prediction interval, use a 95% confidence level with the altitude of 6327 ft (or 6.327 thousand feet). Altitude 8 15 22 28 31 33 Temperature 56 39 24 - 28 - 41 - 60 a. Find the explained variation. (Round to two decimal places as needed.)arrow_forwardThe accompanying table lists overhead widths (cm) of seals measured from photographs and the weights (kg) of the seals. Find the (a) explained variation, (b) unexplained variation, and (c) prediction interval for an overhead width of 8.9 cm using a 99% confidence level. There is sufficient evidence to support a claim of a linear correlation, so it is reasonable to use the regression equation when making predictions. Click the icon to view the seal data. a. The explained variation is (Round to the nearest integer as needed.) b. The unexplained variation is. (Round to the nearest integer as needed.) c. The 99% prediction interval for an overhead width of 8.9 cm is kgarrow_forwardListed below are altitudes (thousands of feet) and outside air temperatures (°F) recorded during a flight. Find the (a) explained variation, (b) unexplained variation, and (c) indicated prediction interval. There is sufficient evidence to support a claim of a linear correlation, so it is reasonable to use the regression equation when making predictions. For the prediction interval, use a 95% confidence level with the altitude of 6327 ft (or 6.327 thousand feet). Altitude Temperature a. Find the explained variation. (Round to two decimal places as needed.) 2 55 8 40 13 25 20 - 3 28 - 26 31 - 41 34 - 53arrow_forwardA Bivariate Regression was conducted to evaluate the predictive relationship between total years of schooling and annual income. The results of the regression model were F(1,88) = 4.1, p < .05. What can be concluded about these results? Group of answer choices total years of schooling is a significant predictor of annual income. total years of schooling is not a significant predictor of annual income.arrow_forward6arrow_forwardLet's study the relationship between brand, camera resolution, and internal storage capacity on the price of smartphones. Use α = .05 to perform a regression analysis of the Smartphones01CS dataset, and then answer the following questions. When you copy and paste output from MegaStat to answer a question, remember to choose to "Keep Formatting" to paste the text. a. Did you find any evidence of multicollinearity and variance inflation among the predictors. Explain your answer using a VIF analysis. b. Copy and paste the normal probability plot for your analysis. Is there any evidence that the errors are not normally distributed? Explain. c. Copy and paste the Residuals vs. Predicted Y-values. Does the pattern support the null hypothesis of constant variance for the errors? Explain. d. Study the residuals analysis. Which observations, if any, have unusual residuals? e. Study the residuals analysis. Calculate the leverage statistic. Which observations, if any, are high leverage…arrow_forwardA Simple Linear Regression (SLR) was performed where the monthly Revenue ("Rev", the y-variable) was regressed on the monthly Advertising Expenditures ("Expend", the x-variable). The Excel-generated Regression output is provided below: ANOVA df SS MS F Significance F Regression 1 492.528125 492.528125 10.65525634 0.046980871 Residual 3 138.671875 46.22395833 Total 4 631.2 Coefficients Standard Error t Stat P-value Lower 95% Upper 95% Intercept 23.1328125 5.324310936 4.344752359 0.022510469 6.188478833 40.07714617 Expend 3.1015625 0.950164031 3.264239014 0.046980871 0.077716489 6.125408511 a. From the Excel-generated Regression output above, give the value of b0, the estimated y-intercept. Round off your answer to the fourth decimal place. b0 =____. b. From the Excel-generated Regression output above, give the value of b1 , the estimated slope. Round off your answer to the fourth decimal place. b1 = _________arrow_forwardarrow_back_iosarrow_forward_ios
- MATLAB: An Introduction with ApplicationsStatisticsISBN:9781119256830Author:Amos GilatPublisher:John Wiley & Sons IncProbability and Statistics for Engineering and th...StatisticsISBN:9781305251809Author:Jay L. DevorePublisher:Cengage LearningStatistics for The Behavioral Sciences (MindTap C...StatisticsISBN:9781305504912Author:Frederick J Gravetter, Larry B. WallnauPublisher:Cengage Learning
- Elementary Statistics: Picturing the World (7th E...StatisticsISBN:9780134683416Author:Ron Larson, Betsy FarberPublisher:PEARSONThe Basic Practice of StatisticsStatisticsISBN:9781319042578Author:David S. Moore, William I. Notz, Michael A. FlignerPublisher:W. H. FreemanIntroduction to the Practice of StatisticsStatisticsISBN:9781319013387Author:David S. Moore, George P. McCabe, Bruce A. CraigPublisher:W. H. Freeman
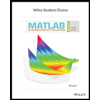
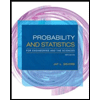
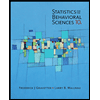
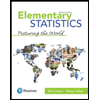
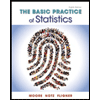
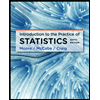