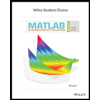
17. When the heights (in inches) and shoe lengths (also in inches) were measured for a large random sample of individuals, it was found that r = 0.89, and a regression equation was constructed in order to further explore the relationship between shoe length and height, with height being the response variable. From this information, what can we conclude?
1. Approximately 89% of the variability in height can be explained by the regression equation.
2. The regression equation relating shoe length to height might have a negative intercept.
3. Because the value of r is less than 1, we should characterize this relationship as being weak.
4.The
5. The regression equation relating shoe length to height must have a slope equal to 0.89.

Trending nowThis is a popular solution!
Step by stepSolved in 2 steps

- What is the null hypothesis to test the significance of the slope in a regression equation? Multiple Choice Ho:B 20 Ho: Bs0 O Ho: B = 0 Ho: B 0arrow_forwardThe table below gives the age and bone density for five randomly selected women. Using this data, consider the equation of the regression line, yˆ=b0+b1x, for predicting a woman's bone density based on her age. Keep in mind, the correlation coefficient may or may not be statistically significant for the data given. Remember, in practice, it would not be appropriate to use the regression line to make a prediction if the correlation coefficient is not statistically significant. Age Bone Density34 35745 34148 33160 32965 325 Step 2 of 6: Find the estimated y-intercept. Round your answer to three decimal places.arrow_forwardA regression analysis was performed to predict weight (y, in kg) using height (x, in cm) among 150 children. The coefficient of determination was . Which of the following is a valid interpretation? a. For each 1-cm increase in height, weight tends to increase by about 0.32 kg b. There is no association between weight and height c. Height accounts for about 32% of the total variability in weight d. The correlation between weight and height is about 0.32arrow_forward
- 1.arrow_forward9. Find the equation of the regression line for the given data. Then construct a scatter plot of the data and draw the regression line. (Each pair of variables has a significant correlation.) Then use the regression equation to predict the value of y for each of the given x-values, if meaningful. The caloric content and the sodium content (in milligrams) for 6 beef hot dogs are shown in the table below. Calories, x Sodium, y 160 130 330 120 70 190 (a) x = 170 calories (c) x = 150 calories 180 (b) x = 80 calories 420 470 360 250 530 (d) x = 210 calories Find the regression equation. x+( (Round to three decimal places as needed.) y = Choose the correct graph below. OA. О В. OC. OD. 560- 560 560 560- 200 G 0IN T> 200 200 Calories Calories Calories Calories (a) Predict the value of y for x = 170. Choose the correct answer below. O A. 411.632 O B. 543.752 O C. 455.672 O D. not meaningful (b) Predict the value of y for x = 80. Choose the correct answer below. O A. 411.632 О В. 257.492 O C.…arrow_forwardTrue or false? If the statement is false, use one or two sentences to justify your answer.arrow_forward
- The following data shows memory scores collected from adults of different ages. Age (X) Memory Score (Y) 25 10 32 10 39 9 48 9 56 7 Use the data to find the regression equation for predicting memory scores from age. The regression equation is: Ŷ = 4.33X + 0.11 Ŷ = -0.11X + 4.33 Ŷ = -0.11X + 13.26 Ŷ = -0.09X + 5.4 Ŷ = -0.09X + 12.6 Use the regression equation you found in question 6 to find the predicted memory scores for the following age: 28 For the calculations, leave two places after the decimal point and do not round: Use the regression equation you found in question 6 to find the predicted memory scores for the following age: 43 For the calculations, leave two places after the decimal point and do not round: Use the regression equation you found in question 6 to find the predicted memory scores for the following age: 50 For the calculations, leave two places after the decimal point and do not round:arrow_forwardThe data show the number of viewers for television stars with certain salaries. Find the regression equation, letting salary be the independent (x) variable. Find the best predicted number of viewers for a television star with a salary of $6 million. Is the result close to the actual number of viewers, 8.9 million? Use a significance level of 0.05. Salary (millions of $) Viewers (millions) Click the icon to view the critical values of the Pearson correlation coefficient r. 98 3.5 3 7 13 12 13 10 2 6.8 6.3 10.2 8.5 4.4 1.8 2.7 What is the regression equation? y=+x (Round to three decimal places as needed.) What is the best predicted number of viewers for a television star with a salary of $6 million? The best predicted number of viewers for a television star with a salary of $6 million is million. (Round to one decimal place as needed.) Is the result close to the actual number of viewers, 8.9 million? O A. The result is very close to the actual number of viewers of 8.9 million. O B. The…arrow_forward17. When the heights (in inches) and shoe lengths (also in inches) were measured for a large random sample of individuals, it was found that r = 0.89, and a regression equation was constructed in order to further explore the relationship between shoe length and height, with height being the response variable. From this information, what can we conclude? 1. The regression equation relating shoe length to height must have a positive intercept. 2. Approximately 89% of the variability in height can be explained by the regression equation. 3. Because the value of r is less than 1, we should characterize this relationship as being weak. 4. The regression equation relating shoe length to height must have a slope equal to 0.89. 5. The correlation coefficient should have no units.arrow_forward
- The table below gives the number of hours spent unsupervised each day as well as the overall grade averages for seven randomly selected middle school students. Using this data, consider the equation of the regression line, y = bo + bjx, for predicting the overall grade average for a middle school student based on the number of hours spent unsupervised each day. Keep in mind, the correlation coefficient may or may not be statistically significant for the data given. Remember, in practice, it would not be appropriate to use the regression line to make a prediction if the correlation coefficient is not statistically significant. Hours Unsupervised 0.5 1.5 2.5 3 4 4.5 6 Overall Grades 89 86 81 79 72 67 62 Table Copy Data Step 1 of 6: Find the estimated slope. Round your answer to three decimal places.arrow_forwarda ift = The table below gives the age and bone density for five randomly selected women. Using this data, consider the equation of the regression line, bo + b₁x, for predicting a woman's bone density based on her age. Keep in mind, the correlation coefficient may or may not be statistically significant for the data given. Remember, In practice, it would not be appropriate to use the regression line to make a prediction if the correlation coefficient is not statistically significant. tab ** Answer How to enter your answer (opens in new window) Step 2 of 6: Find the estimated y-Intercept. Round your answer to three decimal places. esc 1945 ! 1 q alt a Z ebook x360 ng from any angle. @ 2 W S →>> X # 3 e d C $ 4 C r f Age Bone Density 359 % 5 V t g 36 51 63 66 70 357 328 314 310 Oll A 6 b y hp h & 7 O n u j * 8 N O i m ( 9 k O 100- 4 Tables ctrl { [ Keypad Keyboard Shortcuts Previous step answers Submit Answer D + = 11 : 90 ; ? Copy Data O SEAR Dec 2 Table ] USE YOUR SMARTPHONE FOR…arrow_forwardIn this section, we introduced a descriptive measure of the utility of the regression equation for making predictions. a. Identify the term and symbol for that descriptive measure. b. Provide an interpretation.arrow_forward
- MATLAB: An Introduction with ApplicationsStatisticsISBN:9781119256830Author:Amos GilatPublisher:John Wiley & Sons IncProbability and Statistics for Engineering and th...StatisticsISBN:9781305251809Author:Jay L. DevorePublisher:Cengage LearningStatistics for The Behavioral Sciences (MindTap C...StatisticsISBN:9781305504912Author:Frederick J Gravetter, Larry B. WallnauPublisher:Cengage Learning
- Elementary Statistics: Picturing the World (7th E...StatisticsISBN:9780134683416Author:Ron Larson, Betsy FarberPublisher:PEARSONThe Basic Practice of StatisticsStatisticsISBN:9781319042578Author:David S. Moore, William I. Notz, Michael A. FlignerPublisher:W. H. FreemanIntroduction to the Practice of StatisticsStatisticsISBN:9781319013387Author:David S. Moore, George P. McCabe, Bruce A. CraigPublisher:W. H. Freeman
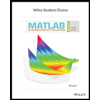
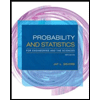
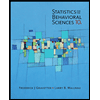
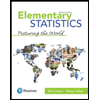
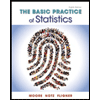
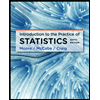