how do i expand this into 1000 words The methodology employed to identify differentially expressed genes (DEGs) in breast cancer using RNA-Seq data involves several systematic steps integrating data retrieval, analysis, normalization, DEG identification, and functional annotation. Initially, raw RNA-Seq data is retrieved from the NCBI GEO database, specifically from dataset GSE216238 (Nakshatri, 2023), which encompasses samples from both breast cancer and normal tissue. Subsequently, the raw data was imported into Excel for initial analysis, leveraging its widespread availability and user-friendly interface. Gene expression data for breast cancer analysis was obtained from the Gene Expression Omnibus (GEO) database. The GEO homepage (https://www.ncbi.nlm.nih.gov/geo/) was accessed, and the "Query & Browse" tab was selected. Advanced Search: Under "Search GEO DataSets," an advanced search was conducted (https://www.ncbi.nlm.nih.gov/gds/advanced). Keywords "breast" and "cancer" were searched in the "Subset description" or "Title" fields, and "high throughput sequencing" was specified as the platform technology type. Browsing: Using the "Repository Browser" option (https://www.ncbi.nlm.nih.gov/geo/summary/), datasets categorized under 'Expression profiling by high throughput sequencing' were explored. If you follow the browsing option above, then follow to 'Expression profiling by high throughput sequencing' that should bring just over 10,000 datasets. Then it would make sense to 'EXPORT' them in a CSV format. That will require three separate downloads due to limitations on the numbers you can export. Selecting from that 10,000 ling list is easier to do using a local EXCEL file rather than online. . To analyse differentially expressed genes (DEGs) in breast cancer using Excel, several straightforward steps were followed. First, gene expression data from breast cancer and normal samples were organized in Excel. Then, using formulas, the fold change in gene expression between cancer and normal samples was calculated. Genes with fold changes above a set threshold were identified as upregulated, while those below were deemed downregulated. In Excel, each gene's raw counts are written in separate columns for both cancer and normal tissue samples. Rows represent genes, while columns are for each sample. This setup allows direct comparison between cancer and normal samples. For each gene, the raw counts in the corresponding columns for cancer samples were compared to those in the columns for normal samples. Metrics such as fold change were calculated to quantify the differences in gene expression between the two groups. Statistical tests, such as the Wald test (Meeta Mistry, 2020) implemented in DESeq2, are utilized to ascertain genes exhibiting significant differences in expression levels. The predetermined significance thresholds used in the analysis typically include fold change, p-value, and false discovery rate (FDR). Fold change represents the minimum magnitude of change in gene expression considered biologically significant. For instance, a fold change greater than 2 (indicating a twofold or higher increase or decrease in expression) is commonly used to identify differentially expressed genes (DEGs). The p-value measures the statistical significance of the observed differences in gene expression between cancer and normal tissue samples. A commonly applied threshold is p < 0.05, indicating that the observed differences are unlikely to have occurred by chance alone. Additionally, the false discovery rate (FDR) adjusts the significance threshold to account for multiple comparisons, reducing the risk of false positives. Typically set at 0.05, an FDR threshold ensures that no more than 5% of identified DEGs are expected to be false positives. The outcomes of this analysis, inclusive of the identified DEGs, their respective fold changes, and statistical significance, are documented in a separate column within the Excel spreadsheet.
how do i expand this into 1000 words
The methodology employed to identify differentially expressed genes (DEGs) in breast cancer using RNA-Seq data involves several systematic steps integrating data retrieval, analysis, normalization, DEG identification, and functional annotation. Initially, raw RNA-Seq data is retrieved from the NCBI GEO database, specifically from dataset GSE216238 (Nakshatri, 2023), which encompasses samples from both breast cancer and normal tissue. Subsequently, the raw data was imported into Excel for initial analysis, leveraging its widespread availability and user-friendly interface. Gene expression data for breast cancer analysis was obtained from the Gene Expression Omnibus (GEO) database.
The GEO homepage (https://www.ncbi.nlm.nih.gov/geo/) was accessed, and the "Query & Browse" tab was selected. Advanced Search: Under "Search GEO DataSets," an advanced search was conducted (https://www.ncbi.nlm.nih.gov/gds/advanced). Keywords "breast" and "cancer" were searched in the "Subset description" or "Title" fields, and "high throughput sequencing" was specified as the platform technology type. Browsing: Using the "Repository Browser" option (https://www.ncbi.nlm.nih.gov/geo/summary/), datasets categorized under 'Expression profiling by high throughput sequencing' were explored. If you follow the browsing option above, then follow to 'Expression profiling by high throughput sequencing' that should bring just over 10,000 datasets. Then it would make sense to 'EXPORT' them in a CSV format. That will require three separate downloads due to limitations on the numbers you can export. Selecting from that 10,000 ling list is easier to do using a local EXCEL file rather than online.
.
To analyse differentially expressed genes (DEGs) in breast cancer using Excel, several straightforward steps were followed. First, gene expression data from breast cancer and normal samples were organized in Excel. Then, using formulas, the fold change in gene expression between cancer and normal samples was calculated. Genes with fold changes above a set threshold were identified as upregulated, while those below were deemed downregulated. In Excel, each gene's raw counts are written in separate columns for both cancer and normal tissue samples. Rows represent genes, while columns are for each sample. This setup allows direct comparison between cancer and normal samples. For each gene, the raw counts in the corresponding columns for cancer samples were compared to those in the columns for normal samples. Metrics such as fold change were calculated to quantify the differences in gene expression between the two groups. Statistical tests, such as the Wald test (Meeta Mistry, 2020) implemented in DESeq2, are utilized to ascertain genes exhibiting significant differences in expression levels.
The predetermined significance thresholds used in the analysis typically include fold change, p-value, and false discovery rate (FDR). Fold change represents the minimum magnitude of change in gene expression considered biologically significant. For instance, a fold change greater than 2 (indicating a twofold or higher increase or decrease in expression) is commonly used to identify differentially expressed genes (DEGs). The p-value measures the statistical significance of the observed differences in gene expression between cancer and normal tissue samples. A commonly applied threshold is p < 0.05, indicating that the observed differences are unlikely to have occurred by chance alone. Additionally, the false discovery rate (FDR) adjusts the significance threshold to account for multiple comparisons, reducing the risk of false positives. Typically set at 0.05, an FDR threshold ensures that no more than 5% of identified DEGs are expected to be false positives. The outcomes of this analysis, inclusive of the identified DEGs, their respective fold changes, and statistical significance, are documented in a separate column within the Excel spreadsheet.

Step by step
Solved in 6 steps

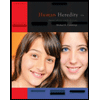
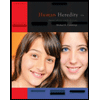