The administrator of a school board in a large county was analyzing the average mathematics test scores in the schools under her control. She noticed that there were dramatic differences in scores among the schools. In an attempt to improve the scores of all the schools, she isolated factors that could account for the differences and were easily obtained from HR. Accordingly, she took a random sample of 40 schools across the county and for each determined: Test Score, Y: the mean test score last year at the school sampled Math Dgr, X1: the percentage of teachers in each school who have at least one university degree in math Age, X2: the mean age of the math teachers at the school sampled Income, X3: the mean annual income of the math teachers at the school sampled Data: Test Score Math Dgr Age Income 73.9 77 52 44.4 59.4 48 32 49 64.6 33 50 52.6 59.8 25 43 39.6 58.8 25 40 40.7 58.7 39 33 38 68.5 71 37 25.1 54.7 24 48 46.5 61.7 25 47 38.4 81.6 49 50 44.6 83.7 56 59 54.8 62.5 46 40 38.5 68.2 75 41 44.8 51.1 25 51 39.9 44 46 38 55.6 65.7 68 45 44.1 69.6 27 49 47.5 54.5 23 31 40 56.5 48 38 40.2 44.8 41 38 28.3 72.6 79 39 44.8 65.3 76 43 34.5 64.9 31 39 28 69.8 63 49 51.4 75.3 72 49 55.2 57 30 23 24.6 52.8 62 34 29.7 71.3 75 36 32.1 55.2 43 35 32.6 62.6 64 50 37.4 58.2 44 45 42.9 71.8 46 29 34.4 69.9 35 39 45.6 70.1 63 34 52.9 66.1 56 36 22.1 71.7 57 59 55.1 68.8 41 40 33.7 45 27 40 19.8 61.9 37 44 48.2 56 36 56 55.9 The least squares regression equation using the percentage of teachers with a math degree to predict mean test score is given by: predicted Math Score = 50.65 + 0.2634(MathDgr%) Estimate a school’s predicted mean test score given that 30% of the math teachers have at least one university math degree. (Be sure to use the correct units as they are recorded in the dataset for inputting 30%).
The administrator of a school board in a large county was analyzing the average mathematics test scores in the schools under her control. She noticed that there were dramatic differences in scores among the schools. In an attempt to improve the scores of all the schools, she isolated factors that could account for the differences and were easily obtained from HR. Accordingly, she took a random sample of 40 schools across the county and for each determined:
Test Score, Y: the mean test score last year at the school sampled
Math Dgr, X1: the percentage of teachers in each school who have at least one university
degree in math
Age, X2: the mean age of the math teachers at the school sampled
Income, X3: the mean annual income of the math teachers at the school sampled
Data:
Test Score | Math Dgr | Age | Income |
73.9 | 77 | 52 | 44.4 |
59.4 | 48 | 32 | 49 |
64.6 | 33 | 50 | 52.6 |
59.8 | 25 | 43 | 39.6 |
58.8 | 25 | 40 | 40.7 |
58.7 | 39 | 33 | 38 |
68.5 | 71 | 37 | 25.1 |
54.7 | 24 | 48 | 46.5 |
61.7 | 25 | 47 | 38.4 |
81.6 | 49 | 50 | 44.6 |
83.7 | 56 | 59 | 54.8 |
62.5 | 46 | 40 | 38.5 |
68.2 | 75 | 41 | 44.8 |
51.1 | 25 | 51 | 39.9 |
44 | 46 | 38 | 55.6 |
65.7 | 68 | 45 | 44.1 |
69.6 | 27 | 49 | 47.5 |
54.5 | 23 | 31 | 40 |
56.5 | 48 | 38 | 40.2 |
44.8 | 41 | 38 | 28.3 |
72.6 | 79 | 39 | 44.8 |
65.3 | 76 | 43 | 34.5 |
64.9 | 31 | 39 | 28 |
69.8 | 63 | 49 | 51.4 |
75.3 | 72 | 49 | 55.2 |
57 | 30 | 23 | 24.6 |
52.8 | 62 | 34 | 29.7 |
71.3 | 75 | 36 | 32.1 |
55.2 | 43 | 35 | 32.6 |
62.6 | 64 | 50 | 37.4 |
58.2 | 44 | 45 | 42.9 |
71.8 | 46 | 29 | 34.4 |
69.9 | 35 | 39 | 45.6 |
70.1 | 63 | 34 | 52.9 |
66.1 | 56 | 36 | 22.1 |
71.7 | 57 | 59 | 55.1 |
68.8 | 41 | 40 | 33.7 |
45 | 27 | 40 | 19.8 |
61.9 | 37 | 44 | 48.2 |
56 | 36 | 56 | 55.9 |
The least squares regression equation using the percentage of teachers with a math degree to predict mean test score is given by:
predicted Math Score = 50.65 + 0.2634(MathDgr%)
Estimate a school’s predicted mean test score given that 30% of the math teachers have at least one university math degree. (Be sure to use the correct units as they are recorded in the dataset for inputting 30%).

Step by step
Solved in 2 steps

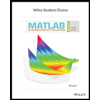
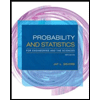
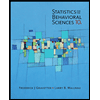
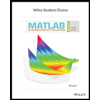
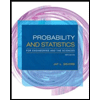
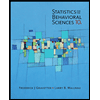
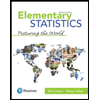
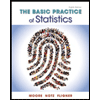
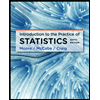