###Run this cell. Do not change the code in this cell from nltk.tokenize import sent_tokenize, word_tokenize from nltk.corpus import gutenberg def get_rawtext(filename='carroll-alice.txt'): text=gutenberg.raw(filename) return text def get_text(filename='carroll-alice.txt'): text=gutenberg.raw(filename) sentences=sent_tokenize(text) tokenized= [word_tokenize(sent.lower()) for sent in sentences] normalised=[["Nth" if (token.endswith( ("nd","st","th")) and token [:-2].isdigit()) else token for token in sent] for sent in tokenized] normalised=[["NUM" if token.isdigit() else token for token in sent] for sent in normalised] filtered=[[word for word in sent if word.isalpha()] for sent in normalised] return filtered
###Run this cell. Do not change the code in this cell from nltk.tokenize import sent_tokenize, word_tokenize from nltk.corpus import gutenberg def get_rawtext(filename='carroll-alice.txt'): text=gutenberg.raw(filename) return text def get_text(filename='carroll-alice.txt'): text=gutenberg.raw(filename) sentences=sent_tokenize(text) tokenized= [word_tokenize(sent.lower()) for sent in sentences] normalised=[["Nth" if (token.endswith( ("nd","st","th")) and token [:-2].isdigit()) else token for token in sent] for sent in tokenized] normalised=[["NUM" if token.isdigit() else token for token in sent] for sent in normalised] filtered=[[word for word in sent if word.isalpha()] for sent in normalised] return filtered
C++ Programming: From Problem Analysis to Program Design
8th Edition
ISBN:9781337102087
Author:D. S. Malik
Publisher:D. S. Malik
Chapter12: Points, Classes, Virtual Functions And Abstract Classes
Section: Chapter Questions
Problem 17SA
Related questions
Question
![- Question 2
This question is about word-cooccurences, collocations and distributional similarity.
Throughout this question, reference will be made to the sample of English stored in text1 (Lewis Carroll's Alice in Wonderland) - a sample of
which is output below.
###Run this cell.
Do not change the code in this cell
from nltk.tokenize import sent_tokenize, word_tokenize
from nltk.corpus import gutenberg
def get_rawtext(filename='carroll-alice.txt'):
text=gutenberg.raw(filename)
return text
def get_text(filename='carroll-alice.txt'):
text=gutenberg.raw(filename)
sentences=sent_tokenize(text)
tokenized= [word_tokenize (sent.lower()) for sent in sentences]
normalised= [["Nth" if (token.endswith( ("nd","st","th")) and token [:-2].isdigit()) else token for token in sent] for sent in tokenized]
normalised=[["NUM" if token.isdigit () else token for token in sent] for sent in normalised]
filtered= [[word for word in sent if word.isalpha(] for sent in normalised]
return filtered
text1=get_text()
text1[:10]
a) Explain what each step in the get_text() function does,](/v2/_next/image?url=https%3A%2F%2Fcontent.bartleby.com%2Fqna-images%2Fquestion%2F3fc6c909-dbd9-42e3-aded-512269cafd79%2Fa746275b-3484-4ab1-af6b-96a6e4567790%2Fld4yhko_processed.png&w=3840&q=75)
Transcribed Image Text:- Question 2
This question is about word-cooccurences, collocations and distributional similarity.
Throughout this question, reference will be made to the sample of English stored in text1 (Lewis Carroll's Alice in Wonderland) - a sample of
which is output below.
###Run this cell.
Do not change the code in this cell
from nltk.tokenize import sent_tokenize, word_tokenize
from nltk.corpus import gutenberg
def get_rawtext(filename='carroll-alice.txt'):
text=gutenberg.raw(filename)
return text
def get_text(filename='carroll-alice.txt'):
text=gutenberg.raw(filename)
sentences=sent_tokenize(text)
tokenized= [word_tokenize (sent.lower()) for sent in sentences]
normalised= [["Nth" if (token.endswith( ("nd","st","th")) and token [:-2].isdigit()) else token for token in sent] for sent in tokenized]
normalised=[["NUM" if token.isdigit () else token for token in sent] for sent in normalised]
filtered= [[word for word in sent if word.isalpha(] for sent in normalised]
return filtered
text1=get_text()
text1[:10]
a) Explain what each step in the get_text() function does,
Expert Solution

This question has been solved!
Explore an expertly crafted, step-by-step solution for a thorough understanding of key concepts.
Step by step
Solved in 2 steps

Knowledge Booster
Learn more about
Need a deep-dive on the concept behind this application? Look no further. Learn more about this topic, computer-science and related others by exploring similar questions and additional content below.Recommended textbooks for you
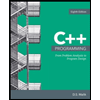
C++ Programming: From Problem Analysis to Program…
Computer Science
ISBN:
9781337102087
Author:
D. S. Malik
Publisher:
Cengage Learning
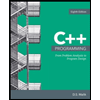
C++ Programming: From Problem Analysis to Program…
Computer Science
ISBN:
9781337102087
Author:
D. S. Malik
Publisher:
Cengage Learning