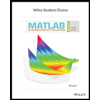
5.25. Representative data on x = carbonation depth (in millimeters) and y = strength (in megapascals) for a sample of concrete core specimens taken from a particular building were read from a plot in the article “The Carbonation of Concrete Structures in the Tropical Environment of Singapore” (Magazine of Concrete Research [1996]: 293-300):
Depth, x 8.0 20.0 20.0 30.0 35.0 40.0 50.0 55.0 65.0
Strength, y 22.8 17.1 21.1 16.1 13.4 12.4 11.4 9.7 6.8
a. Construct a scatterplot. Does the relationship between carbonation depth and strength appear to be linear?
Yes, the relationship between carbonation depth and strength appears to be linear however it is a negative linear relation.
b. Find the equation of the of the least-squares line.
c. What would you predict for strength when carbonation depth is 25 mm?
d. Explain why it would not be reasonable to use the least-squares line to predict strength when carbonation depth is 100 mm.

Trending nowThis is a popular solution!
Step by stepSolved in 4 steps with 2 images

- The accompanying data on x = head circumference z score (a comparison score with peers of the same age - a positive score suggests a larger size than for peers) at age 6 to 14 months and y = volume of cerebral grey matter (in ml) at age 2 to 5 years were read from a graph in an article. Cerebral GreyMatter (ml) 2-5 yr Head Circumfer-ence z Scores at6-14 Months 680 -0.74 690 1.8 700 -0.29 720 0.26 740 0.31 740 2.1 750 1.7 750 2.6 760 1.7 780 1.7 790 2.6 810 2.7 815 3.4 820 2.8 825 0.91 835 2.95 840 2.9 845 2.8 (a) What is the value of the correlation coefficient? (Give the answer to two decimal places.)r = (b) Find the equation of the least-squares line. (Give the answer to two decimal places.) = (c) Predict the volume of cerebral grey matter for a child whose head circumference z score at age 12 months was 1.9. (Give the answer to two decimal places.)arrow_forward15.3 #6 The authors of the article "Age, Spacing and Growth Rate of Tamarix as an Indication of Lake Boundary Fluctuations at Sebkhet Kelbia, Tunisia"† used a simple linear regression model to describe the relationship between y = vigor (average width in centimeters of the last two annual rings) and x = stem density (stems/m2). The estimated model was based on the following data. Also given are the standardized residuals. x 4 5 6 9 14 15 15 19 21 22 y 0.75 1.20 0.55 0.60 0.65 0.55 0.00 0.35 0.45 0.40 Std resid −0.28 1.92 −0.90 −0.28 0.54 0.24 −2.05 −0.12 0.60 0.52 (a) What assumptions are required for the simple linear regression model to be appropriate? (Select all that apply.) A) The random errors associated with different observations are dependent on one another. B) The distribution of e at any given x is not normal. C) The distribution of e at any given x is normal. D) The distribution of e at any particular x value has mean value 0. E) The random…arrow_forwardLaetisaric acid is a compound that holds promise for control of fungus diseases in crop plants. The accompanying data show the results of growing the fungus Pythium (y) in various concentrations of laetisaric acid (x). Laetisaric acid concentration (uG/mL) Fungus growth (mm) 0. 33.3 31.0 29.8 27.8 6. 28.0 6. 29.0 10 25.5 10 23.8 20 18.3 20 15.5 30 11.7 30 10.0 Mean 11.500 23.642 Standard deviation 10.884 7.8471 T =-0.98754 %3D a. State the linear regression equation, and with a 0.01 level of significance, predict the amount (in mm) of fungus growth when 25 uG/mL laetisaric acid is applied. Assume the pairs of data follow a bivariate normal distribution and that the scatterplot shows no evidence of a non-linear relationship in the data. b. Determine the percentage of the variation in fungus growth that is explained by the linear relationship between laetisaric acid concentration and fungus growth. Attack Eilarrow_forward
- 1. Model 1: OLS, using observations 1-706 Dependent variable: RST Coefficient Std. Error t-ratio p-value const 3586.38 38.9124 92.17 <0.0001 *** TOTWRK −0.150746 0.0167403 −9.005 <0.0001 *** Mean dependent var 3266.356 S.D. dependent var 444.4134 Sum squared resid 1.25e+08 S.E. of regression 421.1357 R-squared 0.103287 Adjusted R-squared 0.102014 F(1, 704) 81.08987 P-value(F) 1.99e-1810538.19 Log-likelihood −5267.096 Akaike criterion 10538.19 Schwarz criterion 10547.31 Hannan-Quinn 10541.71 RSTi =3586.38−0.150746 x TOTWRKi , R2=0.103287,SER=421.1357 (38.9124) (0.0167403) Question? could you please help with this question below. 3) By observing the GRETL output in Part (1) above, provide a detailed explanation of the coefficient of determination. Based on your analysis, is this a good model? Why or why not?arrow_forwardneed with bell shape curve pleasearrow_forwardAccess the significsnce of each of the variables in the attached table. For age, write out all of the steps for hypothesis testing. For the other variables, report a p-value and conclusion. Write out the interpretation of the hazards ratios for age and sex in words. What is the estimated hazard ratio for a 10-year increase in age?arrow_forward
- Aa Febru The body mass index (BMI) of a person is defined to be the person's body mass divided by the square of the person's height. The article "Influences of Parameter Uncertainties within the ICRP 66 Respiratory Tract Model: Particle Deposition" (W. Bolch, E. Farfan, et al., Health Physics, 2001:378-394) states that body mass index (in kg/m2) in men aged 25-34 is lognormally distributed with parameters u = 3.215 and o = 0.157. a.Find the mean and standard deviation BMI for men aged 25-34. b.Find the standard deviation of BMI for men aged 25-34. c.Find the median BMI for men aged 25-34. d.What proportion of men aged 25-34 have a BMI less than 20? e.Find the 80th percentile of BMI for men agėd 25 -34. 04... Rext 田arrow_forwardMist (airborne droplets or aerosols) is generated when metal- removing fluids are used in machining operations to cool and lubricate the tool and workpiece. Mist generation is a concern to OSHA, which has recently lowered substantially the workplace standard. The article "Variables Affecting Mist Generaton from Metal Removal Fluids" (Lubrication Engr., 2002: 10-17) gave the accompanying data on x = fluid-flow velocity for a 5% soluble oil (cm/sec) and y = the extent of mist droplets having diameters smaller than 10 μm (mg/m³): x y 89 177 189 354 362 442 965 .40 .60 .48 .66 .61 .69 .99 a. The investigators performed a simple linear regres- sion analysis to relate the two variables. Does a scat- terplot of the data support this strategy? b. What proportion of observed variation in mist can be attributed to the simple linear regression relationship between velocity and mist? c. The investigators were particularly interested in the impact on mist of increasing velocity from 100 to 1000 (a…arrow_forwardAn engineer measures the peak current (in microamps) when a solution containing an amount of nickel (in parts per 10°) is added to a buffer. The experiment was repeated for eleven different values of nickel solutions. A scatterplot showing the data is given below: Peak Current in Eleven Buffers with Added Nickel Solutio 60 120 140 100 Amount of nickel (pp million) Suppose a regression line was added to the plot above. If an additional measurement had been taken with the nickel of 62 parts per 10 and a peak current of 0.38 microamps, adding this observation would: O A. increase the intercept, decrease the slope. OB. increase the intercept, increase the slope. C. decrease the intercept, increase the slope. OD. decrease the intercept, decrease the slope. E. not affect the regression line.arrow_forward
- MATLAB: An Introduction with ApplicationsStatisticsISBN:9781119256830Author:Amos GilatPublisher:John Wiley & Sons IncProbability and Statistics for Engineering and th...StatisticsISBN:9781305251809Author:Jay L. DevorePublisher:Cengage LearningStatistics for The Behavioral Sciences (MindTap C...StatisticsISBN:9781305504912Author:Frederick J Gravetter, Larry B. WallnauPublisher:Cengage Learning
- Elementary Statistics: Picturing the World (7th E...StatisticsISBN:9780134683416Author:Ron Larson, Betsy FarberPublisher:PEARSONThe Basic Practice of StatisticsStatisticsISBN:9781319042578Author:David S. Moore, William I. Notz, Michael A. FlignerPublisher:W. H. FreemanIntroduction to the Practice of StatisticsStatisticsISBN:9781319013387Author:David S. Moore, George P. McCabe, Bruce A. CraigPublisher:W. H. Freeman
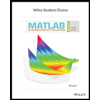
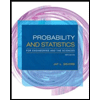
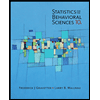
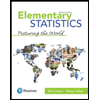
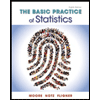
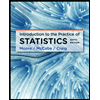