3 K 5 8 K -4.2 -2.5 0 1.7 d(1.7, 2.5) 11.7 (-2.5) |= 4.2 - = - d(3, 8) 13 81-5 Fig. 2. Distance on R x, y = R. Figure 2 illustrates the notation. In the plane and in “ordi- nary" three-dimensional space the situation is similar. In functional analysis we shall study more general "spaces" and "functions" defined on them. We arrive at a sufficiently general and flexible concept of a "space" as follows. We replace the set of real numbers underlying R by an abstract set X (set of elements whose nature is left unspecified) and introduce on X a "distance function" which has only a few of the most fundamental properties of the distance function on R. But what do we mean by "most fundamental"? This question is far from being trivial. In fact, the choice and formula- tion of axioms in a definition always needs experience, familiarity with practical problems and a clear idea of the goal to be reached. In the present case, a development of over sixty years has led to the following concept which is basic and very useful in functional analysis and its applications. 1.1-1 Definition (Metric space, metric). A metric space is a pair (X, d), where X is a set and d is a metric on X (or distance function on X), that is, a function defined² on XXX such that for all x, y, z= X we have: d is real-valued, finite and nonnegative. (M1) (M2) d(x, y)=0 if and only if x = y. (M3) d(x, y) = d(y, x) (Symmetry). (M4) d(x, y)d(x, z)+d(z, y) (Triangle inequality). ■ Problem 4: Metric Tensor Spaces and Graph-Theoretic Embeddings Background: A metric tensor space is a generalization of metric spaces where distances are defined via tensors. Functional analysis often deals with spaces equipped with various tensor norms. Tasks: a. Graph Representation of Tensor Norms: Define a graph T where vertices represent tensors in a tensor product space XY, and edges represent adjacency based on tensor norms (e.g., two tensors u and v are connected if ||uv|| ≤ € for a given tensor norm). Discuss how different tensor norms (projective, injective, Hilbert-Schmidt, etc.) affect the graph's structure. - b. Embedding Tensor Spaces into Metric Graphs: Develop an embedding of a tensor product space XY into a graph T such that the graph metric approximates the tensor norm. Prove the existence of such embeddings for specific tensor norms and discuss their accuracy. c. Graph-Theoretic Duality and Tensor Duals: Investigate how duality in tensor spaces (e.g., dual tensor norms) can be captured through dual graphs. Specifically, construct dual graphs T* corresponding to T and analyze how dual tensor operations are represented. d. Applications to Operator Spaces: Apply the graph constructions from parts (a)-(c) to operator spaces, particularly in representing bounded linear operators between Banach spaces. Analyze how operator space properties, such as completely bounded maps, are reflected in the corresponding graph structures.
3 K 5 8 K -4.2 -2.5 0 1.7 d(1.7, 2.5) 11.7 (-2.5) |= 4.2 - = - d(3, 8) 13 81-5 Fig. 2. Distance on R x, y = R. Figure 2 illustrates the notation. In the plane and in “ordi- nary" three-dimensional space the situation is similar. In functional analysis we shall study more general "spaces" and "functions" defined on them. We arrive at a sufficiently general and flexible concept of a "space" as follows. We replace the set of real numbers underlying R by an abstract set X (set of elements whose nature is left unspecified) and introduce on X a "distance function" which has only a few of the most fundamental properties of the distance function on R. But what do we mean by "most fundamental"? This question is far from being trivial. In fact, the choice and formula- tion of axioms in a definition always needs experience, familiarity with practical problems and a clear idea of the goal to be reached. In the present case, a development of over sixty years has led to the following concept which is basic and very useful in functional analysis and its applications. 1.1-1 Definition (Metric space, metric). A metric space is a pair (X, d), where X is a set and d is a metric on X (or distance function on X), that is, a function defined² on XXX such that for all x, y, z= X we have: d is real-valued, finite and nonnegative. (M1) (M2) d(x, y)=0 if and only if x = y. (M3) d(x, y) = d(y, x) (Symmetry). (M4) d(x, y)d(x, z)+d(z, y) (Triangle inequality). ■ Problem 4: Metric Tensor Spaces and Graph-Theoretic Embeddings Background: A metric tensor space is a generalization of metric spaces where distances are defined via tensors. Functional analysis often deals with spaces equipped with various tensor norms. Tasks: a. Graph Representation of Tensor Norms: Define a graph T where vertices represent tensors in a tensor product space XY, and edges represent adjacency based on tensor norms (e.g., two tensors u and v are connected if ||uv|| ≤ € for a given tensor norm). Discuss how different tensor norms (projective, injective, Hilbert-Schmidt, etc.) affect the graph's structure. - b. Embedding Tensor Spaces into Metric Graphs: Develop an embedding of a tensor product space XY into a graph T such that the graph metric approximates the tensor norm. Prove the existence of such embeddings for specific tensor norms and discuss their accuracy. c. Graph-Theoretic Duality and Tensor Duals: Investigate how duality in tensor spaces (e.g., dual tensor norms) can be captured through dual graphs. Specifically, construct dual graphs T* corresponding to T and analyze how dual tensor operations are represented. d. Applications to Operator Spaces: Apply the graph constructions from parts (a)-(c) to operator spaces, particularly in representing bounded linear operators between Banach spaces. Analyze how operator space properties, such as completely bounded maps, are reflected in the corresponding graph structures.
Advanced Engineering Mathematics
10th Edition
ISBN:9780470458365
Author:Erwin Kreyszig
Publisher:Erwin Kreyszig
Chapter2: Second-order Linear Odes
Section: Chapter Questions
Problem 1RQ
Question
100%
Make sure to answer by hand, make all graphs and give steps how you constructed these, DO NOT SOLVE USING AI
USE : https://drive.google.com/file/d/1a2B3cDeFgHiJkLmNoPqRsTuVwXyZz0/view?usp=sharing
For the reference, and the book kreyszig can be used,

Transcribed Image Text:3
K 5
8
K -4.2
-2.5
0
1.7
d(1.7, 2.5) 11.7 (-2.5) |= 4.2
-
=
-
d(3, 8) 13 81-5
Fig. 2. Distance on R
x, y = R. Figure 2 illustrates the notation. In the plane and in “ordi-
nary" three-dimensional space the situation is similar.
In functional analysis we shall study more general "spaces" and
"functions" defined on them. We arrive at a sufficiently general and
flexible concept of a "space" as follows. We replace the set of real
numbers underlying R by an abstract set X (set of elements whose
nature is left unspecified) and introduce on X a "distance function"
which has only a few of the most fundamental properties of the
distance function on R. But what do we mean by "most fundamental"?
This question is far from being trivial. In fact, the choice and formula-
tion of axioms in a definition always needs experience, familiarity with
practical problems and a clear idea of the goal to be reached. In the
present case, a development of over sixty years has led to the following
concept which is basic and very useful in functional analysis and its
applications.
1.1-1 Definition (Metric space, metric). A metric space is a pair
(X, d), where X is a set and d is a metric on X (or distance function on
X), that is, a function defined² on XXX such that for all x, y, z= X we
have:
d is real-valued, finite and nonnegative.
(M1)
(M2)
d(x, y)=0 if and only if
x = y.
(M3)
d(x, y) = d(y, x)
(Symmetry).
(M4)
d(x, y)d(x, z)+d(z, y)
(Triangle inequality). ■

Transcribed Image Text:Problem 4: Metric Tensor Spaces and Graph-Theoretic Embeddings
Background: A metric tensor space is a generalization of metric spaces where distances are defined
via tensors. Functional analysis often deals with spaces equipped with various tensor norms.
Tasks:
a. Graph Representation of Tensor Norms: Define a graph T where vertices represent tensors in a
tensor product space XY, and edges represent adjacency based on tensor norms (e.g., two
tensors u and v are connected if ||uv|| ≤ € for a given tensor norm). Discuss how different
tensor norms (projective, injective, Hilbert-Schmidt, etc.) affect the graph's structure.
-
b. Embedding Tensor Spaces into Metric Graphs: Develop an embedding of a tensor product space
XY into a graph T such that the graph metric approximates the tensor norm. Prove the
existence of such embeddings for specific tensor norms and discuss their accuracy.
c. Graph-Theoretic Duality and Tensor Duals: Investigate how duality in tensor spaces (e.g., dual
tensor norms) can be captured through dual graphs. Specifically, construct dual graphs T*
corresponding to T and analyze how dual tensor operations are represented.
d. Applications to Operator Spaces: Apply the graph constructions from parts (a)-(c) to operator
spaces, particularly in representing bounded linear operators between Banach spaces. Analyze how
operator space properties, such as completely bounded maps, are reflected in the corresponding
graph structures.
Expert Solution

This question has been solved!
Explore an expertly crafted, step-by-step solution for a thorough understanding of key concepts.
Step by step
Solved in 2 steps with 8 images

Recommended textbooks for you
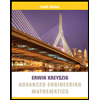
Advanced Engineering Mathematics
Advanced Math
ISBN:
9780470458365
Author:
Erwin Kreyszig
Publisher:
Wiley, John & Sons, Incorporated
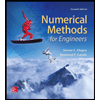
Numerical Methods for Engineers
Advanced Math
ISBN:
9780073397924
Author:
Steven C. Chapra Dr., Raymond P. Canale
Publisher:
McGraw-Hill Education
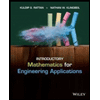
Introductory Mathematics for Engineering Applicat…
Advanced Math
ISBN:
9781118141809
Author:
Nathan Klingbeil
Publisher:
WILEY
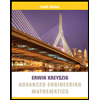
Advanced Engineering Mathematics
Advanced Math
ISBN:
9780470458365
Author:
Erwin Kreyszig
Publisher:
Wiley, John & Sons, Incorporated
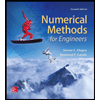
Numerical Methods for Engineers
Advanced Math
ISBN:
9780073397924
Author:
Steven C. Chapra Dr., Raymond P. Canale
Publisher:
McGraw-Hill Education
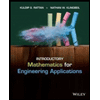
Introductory Mathematics for Engineering Applicat…
Advanced Math
ISBN:
9781118141809
Author:
Nathan Klingbeil
Publisher:
WILEY
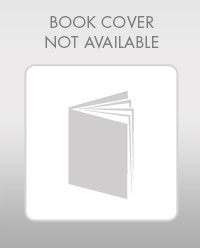
Mathematics For Machine Technology
Advanced Math
ISBN:
9781337798310
Author:
Peterson, John.
Publisher:
Cengage Learning,
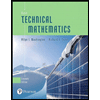
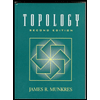