Manually train a hypothesis function h(x) = g(ỗ¹x) based on the following training instances using stochastic gradient ascent rule. The initial values of parameters are 0o = 0.1,0₁ = 0.1, 0₂ = 0.1. The learning rate a is 0.1. Please update each parameter at least five times. X1 0 0 1 1 X2 0 1 0 1 y 1 1 0 0
Manually train a hypothesis function h(x) = g(ỗ¹x) based on the following training instances using stochastic gradient ascent rule. The initial values of parameters are 0o = 0.1,0₁ = 0.1, 0₂ = 0.1. The learning rate a is 0.1. Please update each parameter at least five times. X1 0 0 1 1 X2 0 1 0 1 y 1 1 0 0
Database System Concepts
7th Edition
ISBN:9780078022159
Author:Abraham Silberschatz Professor, Henry F. Korth, S. Sudarshan
Publisher:Abraham Silberschatz Professor, Henry F. Korth, S. Sudarshan
Chapter1: Introduction
Section: Chapter Questions
Problem 1PE
Related questions
Question
![**Training a Hypothesis Function Using Stochastic Gradient Ascent**
To manually train a hypothesis function \( h_{\vec{\theta}}(\vec{x}) = g(\vec{\theta}^T \vec{x}) \), we will apply the stochastic gradient ascent rule with the given dataset. The starting values for the parameters are:
- \(\theta_0 = 0.1\)
- \(\theta_1 = 0.1\)
- \(\theta_2 = 0.1\)
The learning rate \(\alpha\) is set at 0.1. It is necessary to update each parameter at least five times.
**Training Instances:**
\[
\begin{array}{|c|c|c|}
\hline
x_1 & x_2 & y \\
\hline
0 & 0 & 1 \\
0 & 1 & 1 \\
1 & 0 & 0 \\
1 & 1 & 0 \\
\hline
\end{array}
\]
This table represents the input features \(x_1\), \(x_2\), and the target output \(y\) for training the model. Conduct the training by iterating over these instances while adjusting the parameters using the specified learning rate.](/v2/_next/image?url=https%3A%2F%2Fcontent.bartleby.com%2Fqna-images%2Fquestion%2Fb6d57dbf-1951-461b-ba86-1f9bc3e1f7b9%2F1758508e-e043-439e-8f7b-b6b58de92f67%2Ffo8ma7_processed.jpeg&w=3840&q=75)
Transcribed Image Text:**Training a Hypothesis Function Using Stochastic Gradient Ascent**
To manually train a hypothesis function \( h_{\vec{\theta}}(\vec{x}) = g(\vec{\theta}^T \vec{x}) \), we will apply the stochastic gradient ascent rule with the given dataset. The starting values for the parameters are:
- \(\theta_0 = 0.1\)
- \(\theta_1 = 0.1\)
- \(\theta_2 = 0.1\)
The learning rate \(\alpha\) is set at 0.1. It is necessary to update each parameter at least five times.
**Training Instances:**
\[
\begin{array}{|c|c|c|}
\hline
x_1 & x_2 & y \\
\hline
0 & 0 & 1 \\
0 & 1 & 1 \\
1 & 0 & 0 \\
1 & 1 & 0 \\
\hline
\end{array}
\]
This table represents the input features \(x_1\), \(x_2\), and the target output \(y\) for training the model. Conduct the training by iterating over these instances while adjusting the parameters using the specified learning rate.
Expert Solution

Step 1
: Solution ::
step: 1
Gradient Descent algorithm:-
#1. Import All Librariesimport numpy as npimport pandas as pdfrom sklearn.linear_model import Linear Regressionimport math
#2. Train Model Using Sklearndef
predict_using_sklean():df = pd.read_csv("test.csv")r =
Linear Regression()r.fit(df[['x1','x2']],df.y)print(r.intercept_)return r.coef_,
r.intercept_predict_using_sklean()
Step by step
Solved in 2 steps

Knowledge Booster
Learn more about
Need a deep-dive on the concept behind this application? Look no further. Learn more about this topic, computer-science and related others by exploring similar questions and additional content below.Recommended textbooks for you
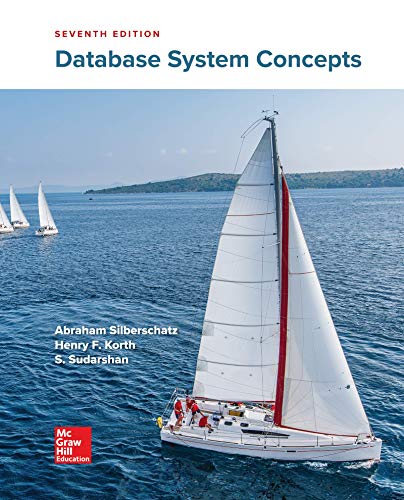
Database System Concepts
Computer Science
ISBN:
9780078022159
Author:
Abraham Silberschatz Professor, Henry F. Korth, S. Sudarshan
Publisher:
McGraw-Hill Education
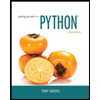
Starting Out with Python (4th Edition)
Computer Science
ISBN:
9780134444321
Author:
Tony Gaddis
Publisher:
PEARSON
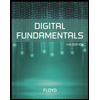
Digital Fundamentals (11th Edition)
Computer Science
ISBN:
9780132737968
Author:
Thomas L. Floyd
Publisher:
PEARSON
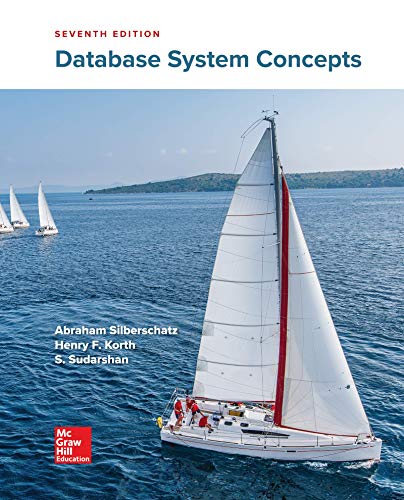
Database System Concepts
Computer Science
ISBN:
9780078022159
Author:
Abraham Silberschatz Professor, Henry F. Korth, S. Sudarshan
Publisher:
McGraw-Hill Education
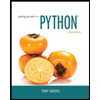
Starting Out with Python (4th Edition)
Computer Science
ISBN:
9780134444321
Author:
Tony Gaddis
Publisher:
PEARSON
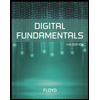
Digital Fundamentals (11th Edition)
Computer Science
ISBN:
9780132737968
Author:
Thomas L. Floyd
Publisher:
PEARSON
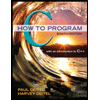
C How to Program (8th Edition)
Computer Science
ISBN:
9780133976892
Author:
Paul J. Deitel, Harvey Deitel
Publisher:
PEARSON
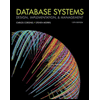
Database Systems: Design, Implementation, & Manag…
Computer Science
ISBN:
9781337627900
Author:
Carlos Coronel, Steven Morris
Publisher:
Cengage Learning
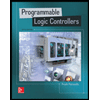
Programmable Logic Controllers
Computer Science
ISBN:
9780073373843
Author:
Frank D. Petruzella
Publisher:
McGraw-Hill Education