In this assignment you will implement linear regression model and evaluate their performance on the California house price data set. (housing.csv) Apply the codes and save them in a seperate .py or .ipynb file DO NOT PUT THE CODE IN YOUR REPORT DOCUMENT, only present your output metrics as well as requested graphs and personal comments in the report. Name the report and code files with surname_studentID_section. You will submit a report and a .py/.ipynb file. Only use the data set version provided with the assignment do not download other versions or use the ready made version in google colab. In the assignment you will do the following: - apply linear regression on each individual numerical feature (drop features : 'ocean_proximity' ‘longitude', 'latitude') - output the coefficients and your self implemented error measures: sum of squared error SSE, mean squared error MSE, use split percentage cross validation with 30% test size and shuffle as True refer to documentations during implementation. In dataset, - report the feature of best performance on each error measure. - plot a graph of each error measure with respect to the feature indicators. - Is there a feature of most importance? state that in your report. Tip: for all work drop 'total_bedrooms' feature as it causes divergence in models. Tip: for inputing individual features to the fit function use: np.array(X_train).reshape(-1,1) which was used in Week3, DO NOT FORGET TO APPLY STANDARD SCALER ON THE FEATURES. # apply linear regression on all numerical feature at once "multivariate linear regression" output coefficients and error measures.
In this assignment you will implement linear regression model and evaluate their performance on the California house price data set. (housing.csv) Apply the codes and save them in a seperate .py or .ipynb file DO NOT PUT THE CODE IN YOUR REPORT DOCUMENT, only present your output metrics as well as requested graphs and personal comments in the report. Name the report and code files with surname_studentID_section. You will submit a report and a .py/.ipynb file. Only use the data set version provided with the assignment do not download other versions or use the ready made version in google colab. In the assignment you will do the following: - apply linear regression on each individual numerical feature (drop features : 'ocean_proximity' ‘longitude', 'latitude') - output the coefficients and your self implemented error measures: sum of squared error SSE, mean squared error MSE, use split percentage cross validation with 30% test size and shuffle as True refer to documentations during implementation. In dataset, - report the feature of best performance on each error measure. - plot a graph of each error measure with respect to the feature indicators. - Is there a feature of most importance? state that in your report. Tip: for all work drop 'total_bedrooms' feature as it causes divergence in models. Tip: for inputing individual features to the fit function use: np.array(X_train).reshape(-1,1) which was used in Week3, DO NOT FORGET TO APPLY STANDARD SCALER ON THE FEATURES. # apply linear regression on all numerical feature at once "multivariate linear regression" output coefficients and error measures.

Trending now
This is a popular solution!
Step by step
Solved in 4 steps with 4 images

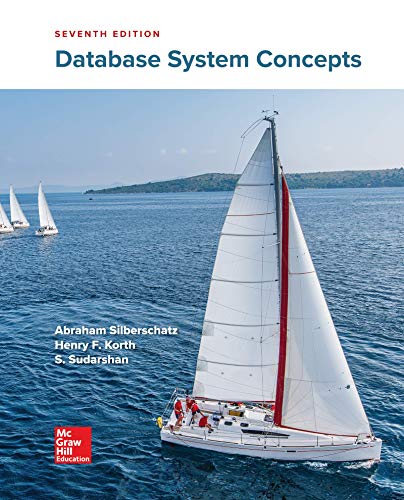
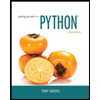
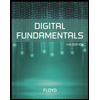
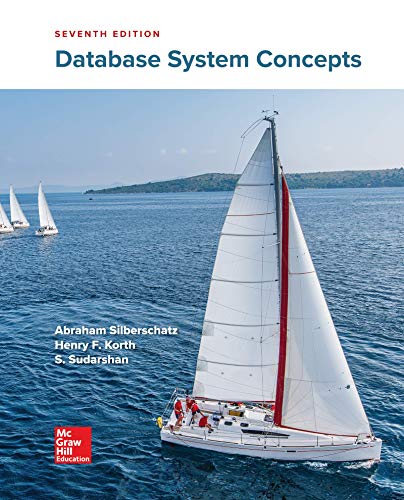
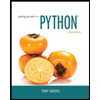
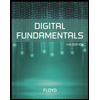
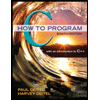
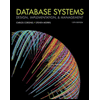
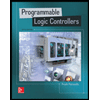