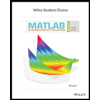
In order to determine whether or not the sales of a company (y in millions of dollars) is related to advertising expenditures (x1 in millions of dollars) and the number of salespeople (x2), data were gathered for 10 (n) years. Part of the regression results are shown below.
|
Coefficients |
Standard Error |
Intercept |
7.0174 |
1.8972 |
x1 |
8.6233 |
2.3968 |
x2 |
0.0858 |
0.1845 |
ANOVA |
|
|
|
|
|
df |
SS |
MS |
F |
Regression |
|
321.11 |
|
|
Residual (Error) |
|
63.39 |
|
|
A. Use the above results and write the multiple regression equation that can be used to predict sales and interpret the statical meaning of the estimated slope coefficient b1(for advertising expenditure). |
B. Estimate the sales volume for an advertising expenditure of 3.5 million dollars and 45 salespeople. Give your answer in dollars. Please show all the relevant calculations. |
C. At α = 0.05, test to determine through an F-test if the fitted equation developed in Part A represents a significant relationship between the independent variables and the dependent variable. Please show all the relevant calculations. |
D. At α = .05, test to see through a t-test if β1 is significantly different from zero. Please show all the relevant calculations. |
E. Determine the multiple coefficient of determination (R^2). Please show all the relevant calculations. |
F. Compute the adjusted coefficient of determination (Adj R^2). Please show all the relevant calculations. |

Trending nowThis is a popular solution!
Step by stepSolved in 4 steps with 3 images

- Researchers are interested in predicting the height of a child based on the heights of their mother and father. Data were collected, which included height of the child (height ), height of the mother ( mothersheight), and height of the father (fathersheight ). The initial analysis used the heights of the parents to predict the height of the child (all units are inches). The results of the analysis, a multiple regression, are presented below. . regress height mothersheight fathersheight Source Model Residual Total height mothersheight fathersheight _cons SS df 208.008457 314.295372 37 2 104.004228 8.49446952 MS 522.303829 39 13.3924059 Coef. Std. Err. .6579529 .1474763 .2003584 .1382237 9.804327 12.39987 t P>|t| 4.46 0.000 C 0.156 0.79 0.434 Number of obs = F( 2, 37) = Prob > F R-squared Adj R-squared Root MSE = .3591375 -.0797093 -15.32021 = 40 12.24 0.0001 0.3983 0.3657 2.9145 [95% Conf. Interval] .9567683 .4804261 34.92886 What is the predicted height for a child born to a mother…arrow_forwardUsing the data, run a regression where you control for “Promotion,” and test the effect of “Wins” on “Attendance”. What should be the attendance if the number of wins is 10? Wins Promotion Attendance 4 29500 36300 6 55700 40100 6 71300 41200 8 87000 53000 6 75000 44000 7 72000 45600 5 55300 39000 7 81600 47500arrow_forwardListed below are foot lengths (mm) and heights (mm) of males. Find the regression equation, letting foot length be the predictor (x) variable. Find the best predicted height of a male with a foot length of 273.3 mm. How does the result compare to the actual height of 1776 mm? Foot Length 281.9 278.3 253.2 258.7 278.7 257.8 274.2 262.2 Height 1784.8 1771.0 1675.6 1645.9 1858.7 1710.1 1789.2 1737.4 the regression equation is y=enter your response here+enter your response herex. (Round the y-intercept to the nearest integer as needed. Round the slope to two decimal places as needed.) The best predicted height of a male with a foot length of 273.3 mm is enter your response here mm. (Round to the nearest integer as needed.)arrow_forward
- Listed below are foot lengths (mm) and heights (mm) of males. Find the regression equation, letting foot length be the predictor (x) variable. Find the best predicted height of a male with a foot length of 272.7 mm. How does the result compare to the actual height of 1776 mm? Foot Length 282.3 277.8 252.8 258.7 279.0 258.4 274.1 261.7 Height 1785.0 1771.0 1675.7 1645.7 1859.3 1710.2 1789.2 1737.0 The regression equation is ŷ = + (x. (Round the y-intercept to the nearest integer as needed. Round the slope to two decimal places as needed.) The best predicted height of a male with a foot length of 272.7 mm is (Round to the nearest integer as needed.) How does the result compare to the actual height of 1776 mm? O A. The result is close to the actual height of 1776 mm. O B. The result is exactly the same as the actual height of 1776 mm. O C. The result is very different from the actual height of 1776 mm. O D. The result does not make sense given the context of the data. C mm.arrow_forwardA statistical program is recommended. The owner of Showtime Movie Theaters, Inc., would like to predict weekly gross revenue as a function of advertising expenditures. Historical data for a sample of eight weeks follow. Weekly Television Gross Newspaper Advertising Advertising ($1,000s) ($1,000s) Revenue ($1,000s) 96 5.0 1.5 90 2.0 2.0 95 4.0 1.5 92 2.5 2.5 95 3.0 3.3 94 3.5 2.3 94 2.5 4.2 94 3.0 2.5 1 (a) Develop an estimated regression equation with the amount of television advertising as the independent variable. (Round your numerical values to two decimal places. Let x₁ represent the amount of television advertising in $1,000s and y represent the weekly gross revenue in $1,000s.) y = 88.64 + 1.60x1 X (b) Develop an estimated regression equation with both television advertising and newspaper advertising as the independent variables. (Round your numerical values to two decimal places. Let x₁ represent the amount of television advertising in $1,000s, x₂ represent the amount of…arrow_forwardYou believe that the price of Zoom Videoconferencing stock and the price of American Airlines stock will move in opposite directions. In order to test this relationship, we do a simple regression with the following variables:A - dependent variable : month end price of American Airlines stockZ - independent variable: month end price of Zoom Videoconferencing stock Data from April 2019 through December 2020 (21 observations) is availableBased on the data, we compute the following:Var (Z) = 20927.702Cov (A,Z) = -899.153E(A) = 20.790E(Z) = 187.530Std Error of Estimate = 6.088TSS = 1476.830 Consider the equation At = b0 + b1 Zt + εtBased on the numbers given above, complete the following table Variable Estimate Std error t-statistic Slope b1 .00941 Constant b0 2.2088 R-square N/A N/A F statistic N/A N/A Are the coefficients (slope and/or constant) significant at the .05 level?arrow_forward
- Using the data, run a regression where you control for “Promotion,” and test the effect of “Wins” on “Attendance”. Does “Promotion” have a significant effect on “Attendance”? Wins Promotion Attendance 4 29500 36300 6 55700 40100 6 71300 41200 8 87000 53000 6 75000 44000 7 72000 45600 5 55300 39000 7 81600 47500arrow_forwardIn linear regression analysis, the coefficient for the x-variable when the y-variable is regressed on the x-variable can be thought of as: Note: more than one answer may be correct Group of answer choices How much the value of the predicted y-variable will change when the x-variable changes by one unit. In the simple (two variable) linear regression model, the coefficient can be thought as the slope coefficient that measures the responsiveness of y to changes in x. The estimated coefficient will change if the sample containing x and y changes. The sample coefficient is an estimate of the population coefficient Before interpreting the coefficient for the x-variable, we should test whether the coefficient is statistically significant.arrow_forwardThe accompanying data are the number of wins and the earned run averages (mean number of earned runs allowed per nine innings pitched) for eight baseball pitchers in a recent season. Find the equation of the regression line. Then construct a scatter plot of the data and draw the regression line. Then use the regression equation to predict the value of y for each of the given x-values, if meaningful. If the x-value is not meaningful to predict the value of y, explain why not. (a) x = 5 wins Click the icon to view the table of numbers of wins and earned run average. (b) x= 10 wins (c) x=21 wins (d) x= 15 wins The equation of the regression line is y = x+ | (Round to two decimal places as needed.) !!arrow_forward
- An automobile rental company wants to predict the yearly maintenance expense (Y) for an automobile using the number of miles driven during the year () and the age of the car (, in years) at the beginning of the year. The company has gathered the data on 10 automobiles and run a regression analysis with the results shown below:. Summary measures Multiple R 0.9689 R-Square 0.9387 Adj R-Square 0.9212 StErr of Estimate 72.218 Regression coefficients Coefficient Std Err t-value p-value Constant 33.796 48.181 0.7014 0.5057 Miles Driven 0.0549 0.0191 2.8666 0.0241 Age of car 21.467 20.573 1.0434 0.3314 Use the information above to estimate the annual maintenance expense for a 10 years old car with 60,000 miles.arrow_forwardIs it reasonable to interpret the y-intercept of the regression line? Why or why not? One home that is 1,750 square feet is listed at $590,000. Is this home's price above or below average for a home of this size? Use residuals and show your work to answer. footaget) ## (Intercept) 21.14411 136.31449 0.155 0.87954 ## footage 0.28388 0.08516 3.333 0.00667 ** ## Residual standard error: 71.64 on 11 degrees of freedom ## Multiple R-squared: 0.5025, Adjusted R-squared: 0.4573 ## F-statistic: 11.11 on 1 and 11 DF, p-value: 0.006671arrow_forwardResearchers are interested in predicting the height of a child based on the heights of their mother and father. Data were collected, which included height of the child (height ), height of the mother ( mothersheight ), and height of the father ( fathersheight ). The initial analysis used the heights of the parents to predict the height of the child (all units are inches). The results of the analysis, a multiple regression, are presented below. . regress height mothersheight fathersheight Source Model Residual Total height mothersheight fathersheight _cons SS df 208.008457 314.295372 37 522.303829 2 104.004228 8.49446952 MS 39 13.3924059 Coef. Std. Err. .6579529 .1474763 .2003584 .1382237 9.804327 12.39987 Calculate the test statistic that is labeled "C" in the output. t P>|t| 4.46 0.000 C 0.156 0.79 0.434 Number of obs = F( 2, Prob > F 37) = R-squared Adj R-squared = Root MSE 40 12.24 0.0001 = 0.3983 0.3657 2.9145 .3591375 -.0797093 -15.32021 = [95% Conf. Intervall .9567683 .4804261…arrow_forward
- MATLAB: An Introduction with ApplicationsStatisticsISBN:9781119256830Author:Amos GilatPublisher:John Wiley & Sons IncProbability and Statistics for Engineering and th...StatisticsISBN:9781305251809Author:Jay L. DevorePublisher:Cengage LearningStatistics for The Behavioral Sciences (MindTap C...StatisticsISBN:9781305504912Author:Frederick J Gravetter, Larry B. WallnauPublisher:Cengage Learning
- Elementary Statistics: Picturing the World (7th E...StatisticsISBN:9780134683416Author:Ron Larson, Betsy FarberPublisher:PEARSONThe Basic Practice of StatisticsStatisticsISBN:9781319042578Author:David S. Moore, William I. Notz, Michael A. FlignerPublisher:W. H. FreemanIntroduction to the Practice of StatisticsStatisticsISBN:9781319013387Author:David S. Moore, George P. McCabe, Bruce A. CraigPublisher:W. H. Freeman
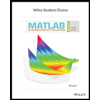
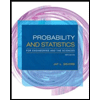
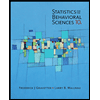
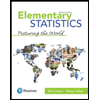
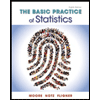
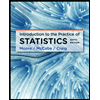