Explain of each detail of this code : import numpy as np import pandas as pd from sklearn.cluster import KMeans from sklearn.neighbors import KNeighborsClassifier from sklearn.model_selection import train_test_split from sklearn.preprocessing import normalize from sklearn.metrics import confusion_matrix from sklearn.metrics import accuracy_score from sklearn.metrics import precision_score from sklearn.metrics import recall_score from sklearn.metrics import f1_score import time tic=time.time() full_data=pd.read_csv("../input/creditcard.csv") full_data=full_data.sample(frac=1)#randomize the whole dataset full_features=full_data.drop(["Time","Class"],axis=1) full_labels=pd.DataFrame(full_data[["Class"]]) full_features_array=full_features.values full_labels_array=full_labels.values train_features,test_features,train_labels,test_labels=train_test_split(full_features_array,full_labels_array,train_size=0.90) train_features=normalize(train_features) test_features=normalize(test_features) #k_means_classification --> k_means_clustering, confsion_matrix, reassigning kmeans=KMeans(n_clusters=2,random_state=0,algorithm="elkan",max_iter=10000,n_jobs=-1) kmeans.fit(train_features) kmeans_predicted_train_labels=kmeans.predict(train_features) #confusion matrix # tn fp # fn tp print("tn --> true negatives") print("fp --> false positives") print("fn --> false negatives") print("tp --> true positives") tn,fp,fn,tp=confusion_matrix(train_labels,kmeans_predicted_train_labels).ravel() reassignflag=False if tn+tp",kmeans_accuracy_score) print("Precison -->",kmeans_precison_score) print("Recall -->",kmeans_recall_score) print("F1 -->",kmeans_f1_score) #k_nearest_neighbours_classification knn=KNeighborsClassifier(n_neighbors=5,algorithm="kd_tree",n_jobs=-1) knn.fit(train_features,train_labels.ravel()) knn_predicted_test_labels=knn.predict(test_features) #calculating confusion matrix for knn tn,fp,fn,tp=confusion_matrix(test_labels,knn_predicted_test_labels).ravel() #scoring knn knn_accuracy_score=accuracy_score(test_labels,knn_predicted_test_labels) knn_precison_score=precision_score(test_labels,knn_predicted_test_labels) knn_recall_score=recall_score(test_labels,knn_predicted_test_labels) knn_f1_score=f1_score(test_labels,knn_predicted_test_labels) #printing print("") print("K-Nearest Neighbours") print("Confusion Matrix") print("tn =",tn,"fp =",fp) print("fn =",fn,"tp =",tp) print("Scores") print("Accuracy -->",knn_accuracy_score) print("Precison -->",knn_precison_score) print("Recall -->",knn_recall_score) print("F1 -->",knn_f1_score) #time elapsed toc=time.time() elapsedtime=toc-tic print("") print("Time Taken : "+str(elapsedtime)+"seconds")
Explain of each detail of this code :
import numpy as np
import pandas as pd
from sklearn.cluster import KMeans
from sklearn.neighbors import KNeighborsClassifier
from sklearn.model_selection import train_test_split
from sklearn.preprocessing import normalize
from sklearn.metrics import confusion_matrix
from sklearn.metrics import accuracy_score
from sklearn.metrics import precision_score
from sklearn.metrics import recall_score
from sklearn.metrics import f1_score
import time
tic=time.time()
full_data=pd.read_csv("../input/creditcard.csv")
full_data=full_data.sample(frac=1)#randomize the whole dataset
full_features=full_data.drop(["Time","Class"],axis=1)
full_labels=pd.DataFrame(full_data[["Class"]])
full_features_array=full_features.values
full_labels_array=full_labels.values
train_features,test_features,train_labels,test_labels=train_test_split(full_features_array,full_labels_array,train_size=0.90)
train_features=normalize(train_features)
test_features=normalize(test_features)
#k_means_classification --> k_means_clustering, confsion_matrix, reassigning
kmeans=KMeans(n_clusters=2,random_state=0,
kmeans.fit(train_features)
kmeans_predicted_train_labels=kmeans.predict(train_features)
#confusion matrix
# tn fp
# fn tp
print("tn --> true negatives")
print("fp --> false positives")
print("fn --> false negatives")
print("tp --> true positives")
tn,fp,fn,tp=confusion_matrix(train_labels,kmeans_predicted_train_labels).ravel()
reassignflag=False
if tn+tp<fn+fp:
# clustering is opposite of original classification
reassignflag=True
kmeans_predicted_test_labels=kmeans.predict(test_features)
if reassignflag:
kmeans_predicted_test_labels=1-kmeans_predicted_test_labels
#calculating confusion matrix for kmeans
tn,fp,fn,tp=confusion_matrix(test_labels,kmeans_predicted_test_labels).ravel()
#scoring kmeans
kmeans_accuracy_score=accuracy_score(test_labels,kmeans_predicted_test_labels)
kmeans_precison_score=precision_score(test_labels,kmeans_predicted_test_labels)
kmeans_recall_score=recall_score(test_labels,kmeans_predicted_test_labels)
kmeans_f1_score=f1_score(test_labels,kmeans_predicted_test_labels)
#printing
print("")
print("K-Means")
print("Confusion Matrix")
print("tn =",tn,"fp =",fp)
print("fn =",fn,"tp =",tp)
print("Scores")
print("Accuracy -->",kmeans_accuracy_score)
print("Precison -->",kmeans_precison_score)
print("Recall -->",kmeans_recall_score)
print("F1 -->",kmeans_f1_score)
#k_nearest_neighbours_classification
knn=KNeighborsClassifier(n_neighbors=5,algorithm="kd_tree",n_jobs=-1)
knn.fit(train_features,train_labels.ravel())
knn_predicted_test_labels=knn.predict(test_features)
#calculating confusion matrix for knn
tn,fp,fn,tp=confusion_matrix(test_labels,knn_predicted_test_labels).ravel()
#scoring knn
knn_accuracy_score=accuracy_score(test_labels,knn_predicted_test_labels)
knn_precison_score=precision_score(test_labels,knn_predicted_test_labels)
knn_recall_score=recall_score(test_labels,knn_predicted_test_labels)
knn_f1_score=f1_score(test_labels,knn_predicted_test_labels)
#printing
print("")
print("K-Nearest Neighbours")
print("Confusion Matrix")
print("tn =",tn,"fp =",fp)
print("fn =",fn,"tp =",tp)
print("Scores")
print("Accuracy -->",knn_accuracy_score)
print("Precison -->",knn_precison_score)
print("Recall -->",knn_recall_score)
print("F1 -->",knn_f1_score)
#time elapsed
toc=time.time()
elapsedtime=toc-tic
print("")
print("Time Taken : "+str(elapsedtime)+"seconds")

Trending now
This is a popular solution!
Step by step
Solved in 10 steps

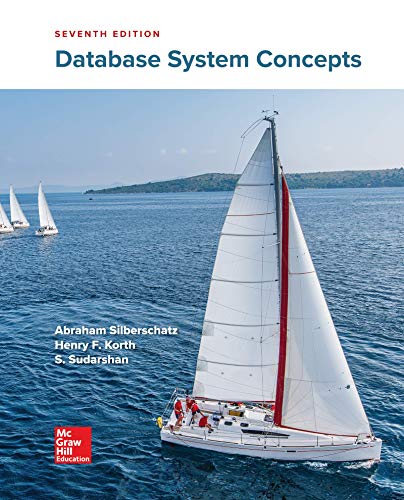
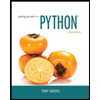
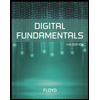
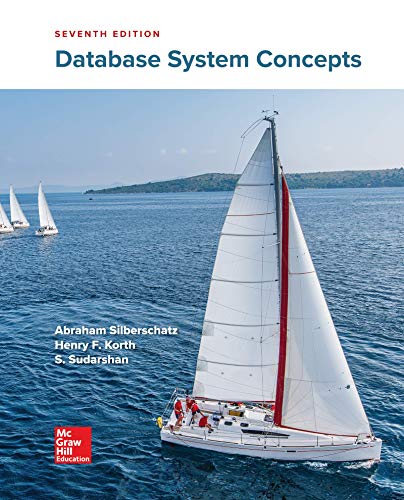
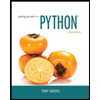
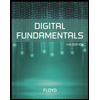
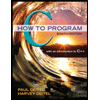
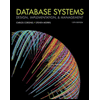
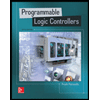