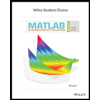
Consider the fitted values from a simple linear regression model with intercept: yˆ = 7 + 4x. Assume that the total number of observations is n = 20. In addition, the explained sum of squares is SSE = 10 and the residual sum of squares is SSR = 30. Under the classical Gauss-Markov assumptions, a) What is the value of the R2 ?

Step by stepSolved in 2 steps

Consider the fitted values from a simple linear regression model with intercept: yˆ = 7 + 4x. Assume that the total number of observations is n = 20. In addition, the explained sum of squares is SSE = 10 and the residual sum of squares is SSR = 30. Under the classical Gauss-Markov assumptions,
a) What is the value of the R^2 ?
b) What is the value of the adjusted R^2 ?
c) What is the standard error of the regression?
d) What is the standard error of the estimated slope coefficient?
e) What is the value of the F test?
Consider the fitted values from a simple linear regression model with intercept: yˆ = 7 + 4x. Assume that the total number of observations is n = 20. In addition, the explained sum of squares is SSE = 10 and the residual sum of squares is SSR = 30. Under the classical Gauss-Markov assumptions
R^2 = 0.75,
What is the value of the adjusted R2?
Consider the fitted values from a simple linear regression model with intercept: yˆ = 7 + 4x. Assume that the total number of observations is n = 20. In addition, the explained sum of squares is SSE = 10 and the residual sum of squares is SSR = 30. Under the classical Gauss-Markov assumptions,
a) What is the value of the R^2 ?
b) What is the value of the adjusted R^2 ?
c) What is the standard error of the regression?
d) What is the standard error of the estimated slope coefficient?
e) What is the value of the F test?
Consider the fitted values from a simple linear regression model with intercept: yˆ = 7 + 4x. Assume that the total number of observations is n = 20. In addition, the explained sum of squares is SSE = 10 and the residual sum of squares is SSR = 30. Under the classical Gauss-Markov assumptions
R^2 = 0.75,
What is the value of the adjusted R2?
- The null hypothesis being tested in the least-squares regression output for B is B1 = B1,0=1. True Falsearrow_forwardThe y-interept bo of a least-squares regression line has a useful interpretation only if the x-values are either all positive or all negative. Determine if the statement is true or false. Why? If the statement is false, rewrite as a true statement.arrow_forwardSuppose we have a multiple regression model with 2 predictors and an intercept. (Without any interaction or higher order terms, we have only the 2 predictors in the model and the intercept.) We have only n= 6 observations (so it would be rather silly to fit this model to this data, but let's pretend it is reasonable). We find the values of the first 5 residuals are: 2.6, 2.3, 2.5, -1.5, -1.4 What is the value of MSRes for this multiple regression model?arrow_forward
- Consider the following population model for household consumption: cons = a + b1 * inc+ b2 * educ+ b3 * hhsize + u where cons is consumption, inc is income, educ is the education level of household head, hhsize is the size of a household. Suppose a researcher estimates the model and gets the predicted value, cons_hat, and then runs a regression of cons_hat on educ, inc, and hhsize. Which of the following choice is correct and please explain why. A) be certain that R^2 = 1 B) be certain that R^2 = 0 C) be certain that R^2 is less than 1 but greater than 0. D) not be certainarrow_forwardShow calculations or explanation for each question. a) Which of the following techniques is used to predict the value of one variable on thebasis of other variables?a. Correlation analysisb. Coefficient of correlationc. Covarianced. Regression analysis b) In the least squares regression line, y^=3-2x the predicted value of y equals:a. 1.0 when x = −1.0b. 2.0 when x = 1.0c. 2.0 when x = −1.0d. 1.0 when x = 1.0 c) In the simple linear regression model, the y-intercept represents the:a. change in y per unit change in x.b. change in x per unit change in y.c. value of y when x = 0.d. value of x when y = 0.arrow_forwardA prospective MBA student would like to examine the factors that impact starting salary upon graduation and decides to develop a model that uses program per-year tuition as a predictor of starting salary. Data were collected for 37 full-time MBA programs offered at private universities. The least squares equation was found Y; = -13258.594 + 2.422X;, where X; is the program per-year tuition and Y; is the predicted mean starting salary. To perform a residual analysis for these data, the following results are obtained. of regression have been seriously violated. Residual index plot QQ Plot of Residuals Residuals Residuals 20000- 20000 0. -20000 -20000 a) To evaluate whether the assumption of linearity has been violated, which of the following graph shou be examined? A. Predicted Values vs. Residuals B. Residual index plot C. QQ plot of residuals D. Residuals vs. Progrm Per-Year Tuition ($) b) To evaluate whether the assumption of normality has been violated, which of the following graph…arrow_forward
- )A county real estate appraiser wants to develop a statistical model to predict the appraised value of 3) houses in a section of the county called East Meadow. One of the many variables thought to be an important predictor of appraised value is the number of rooms in the house. Consequently, the appraiser decided to fit the simple linear regression model: E(u) = Bo + Bix, where y = appraised value of the house (in thousands of dollars) and x = number of rooms. Using data collected for a sample of n = 73 houses in Fast Meadow, the following results were obtained: y = 73.80 + 19.72x What are the properties of the least squares line, y = 73.80 + 19.72x? A) Average error of prediction is 0, and SSE is minimum. B) It will always be a statistically useful predictor of y. C) It is normal, mean 0, constant variance, and independent. D) All 73 of the sample y-values fall on the line.arrow_forwardThis data table contians the listed prices and weights of the diamonds in 48 rings offered for sale in The Singapore Times. The prices are in Singapore dollars, with the weights in crats. Estimate the linear regression using weight as the explanatory variable and price as the response variable using the following two methods: a) Create a scatterplot with trendline (be sure to show the equation and R-square on the chart.) b) Use the Data Analysis Toolpak to do the work for you.arrow_forwardA year-long fitness center study sought to determine if there is a relationship between the amount of muscle mass gained y(kilograms) and the weekly time spent working out under the guidance of a trainer x(minutes). The resulting least-squares regression line for the study is y=2.04 + 0.12x A) predictions using this equation will be fairly good since about 95% of the variation in muscle mass can be explained by the linear relationship with time spent working out. B)Predictions using this equation will be faily good since about 90.25% of the variation in muscle mass can be explained by the linear relationship with time spent working out C)Predictions using this equation will be fairly poor since only about 95% of the variation in muscle mass can be explained by the linear relationship with time spent working out D) Predictions using this equation will be fairly poor since only about 90.25% of the variation in muscle mass can be explained by the linear relationship with time spent…arrow_forward
- MATLAB: An Introduction with ApplicationsStatisticsISBN:9781119256830Author:Amos GilatPublisher:John Wiley & Sons IncProbability and Statistics for Engineering and th...StatisticsISBN:9781305251809Author:Jay L. DevorePublisher:Cengage LearningStatistics for The Behavioral Sciences (MindTap C...StatisticsISBN:9781305504912Author:Frederick J Gravetter, Larry B. WallnauPublisher:Cengage Learning
- Elementary Statistics: Picturing the World (7th E...StatisticsISBN:9780134683416Author:Ron Larson, Betsy FarberPublisher:PEARSONThe Basic Practice of StatisticsStatisticsISBN:9781319042578Author:David S. Moore, William I. Notz, Michael A. FlignerPublisher:W. H. FreemanIntroduction to the Practice of StatisticsStatisticsISBN:9781319013387Author:David S. Moore, George P. McCabe, Bruce A. CraigPublisher:W. H. Freeman
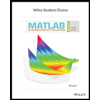
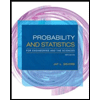
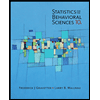
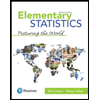
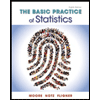
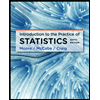