A study of obesity and metabolic syndrome used data collected from 15 students, and included systolic blood pressure (SBP), weight, and BMI. These data are presented in Table 2 (See data 3). Correlations for the three variables are shown in Figure 1. The very large and significant correlation between the variables weight and BMI suggests that including both of these variables in the model is inappropriate because of the high level of redundancy in the information provided by these variables. This makes logical sense since BMI is a function of weight. How to decide which of the variables to retain for constructing the regression model? Table 2 Data from 8 Random Sample of 15 Students Case NO SBP WEIGHT(lbs.) BMI metabolic syndrome 1 126 125 24.41 0 2 129 130 23.77 0 126 132 20.07 0 123 200 27.12 1 124 321 39.07 1 125 100 20.9 0 127 138 22.96 0 125 138 24.44 0 123 149 23.33 0 19 180 25.82 0 127 184 26.4 0 126 251 31.87 1 122 197 26.72 1 126 107 20.22 0 125 125 23.62 0 3 4 5 6 7 8 9 10 11 12 13 14 15 Correlations SBP WEIGHT (lbs.) SBP 1 Pearson Correlation Sig. (2-tailed) 15 WEIGHT(lbs.) -.090 964** N Pearson Correlation. Sig (2-tailed) N .749 .000 15 15 BMI Pearson Correlation -.042 1 Sig. (2-tailed) 883 .000 N 15 15 15 **. Correlation is significant at the 0.01 level (2-tailed). Figure 1 Comolations calaulated in SPSS -.090 749 15 1 15 BMI 964 -.042 .883 15
A study of obesity and metabolic syndrome used data collected from 15 students, and included systolic blood pressure (SBP), weight, and BMI. These data are presented in Table 2 (See data 3). Correlations for the three variables are shown in Figure 1. The very large and significant correlation between the variables weight and BMI suggests that including both of these variables in the model is inappropriate because of the high level of redundancy in the information provided by these variables. This makes logical sense since BMI is a function of weight. How to decide which of the variables to retain for constructing the regression model? Table 2 Data from 8 Random Sample of 15 Students Case NO SBP WEIGHT(lbs.) BMI metabolic syndrome 1 126 125 24.41 0 2 129 130 23.77 0 126 132 20.07 0 123 200 27.12 1 124 321 39.07 1 125 100 20.9 0 127 138 22.96 0 125 138 24.44 0 123 149 23.33 0 19 180 25.82 0 127 184 26.4 0 126 251 31.87 1 122 197 26.72 1 126 107 20.22 0 125 125 23.62 0 3 4 5 6 7 8 9 10 11 12 13 14 15 Correlations SBP WEIGHT (lbs.) SBP 1 Pearson Correlation Sig. (2-tailed) 15 WEIGHT(lbs.) -.090 964** N Pearson Correlation. Sig (2-tailed) N .749 .000 15 15 BMI Pearson Correlation -.042 1 Sig. (2-tailed) 883 .000 N 15 15 15 **. Correlation is significant at the 0.01 level (2-tailed). Figure 1 Comolations calaulated in SPSS -.090 749 15 1 15 BMI 964 -.042 .883 15
MATLAB: An Introduction with Applications
6th Edition
ISBN:9781119256830
Author:Amos Gilat
Publisher:Amos Gilat
Chapter1: Starting With Matlab
Section: Chapter Questions
Problem 1P
Related questions
Question

Transcribed Image Text:A study of obesity and metabolic syndrome used data collected from 15
students, and included systolic blood pressure (SBP), weight, and BMI. These
data are presented in Table 2 (See data 3). Correlations for the three
variables are shown in Figure 1. The very large and significant correlation
between the variables weight and BMI suggests that including both of these
variables in the model is inappropriate because of the high level of
redundancy in the information provided by these variables. This makes
logical sense since BMI is a function of weight. How to decide which of the
variables to retain for constructing the regression model?
Table 2 Data from 8 Random Sample of 15 Students
Case NO SBP
WEIGHT(lbs.) BMI
metabolic syndrome
1
126
125
24.41
0
2
129
130
23.77
0
3
126
132
20.07
0
4
123
200
27.12
1
5
124
321
39.07
1
6
125
100
20.9
0
127
138
22.96
0
125
138
24.44
0
123
149
23.33
0
19
180
25.82
0
127
184
26.4
0
126
251
31.87
1
122
197
26.72
1
126
107
20.22
0
125
125
23.62
0
7
8
9
10
11
12
13
14
15
Correlations
SBP
WEIGHT
(lbs.)
1
15
-.090
749
15
-.042
883
SBP
Pearson Correlation
Sig. (2-tailed)
WEIGHT(lbs.)
N
Pearson Correlation
Sig (2-tailed)
N
BMI
Pearson Correlation
Sig. (2-tailed)
.000
N
15
15
15
**. Correlation is significant at the 0.01 level (2-tailed).
Figure 1 Correlations calculated in SPSS
-.090
749
15
1
15
BMI
964
-.042
883
15
964
.000
15
1
Expert Solution

This question has been solved!
Explore an expertly crafted, step-by-step solution for a thorough understanding of key concepts.
Step by step
Solved in 2 steps

Recommended textbooks for you
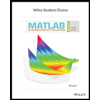
MATLAB: An Introduction with Applications
Statistics
ISBN:
9781119256830
Author:
Amos Gilat
Publisher:
John Wiley & Sons Inc
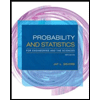
Probability and Statistics for Engineering and th…
Statistics
ISBN:
9781305251809
Author:
Jay L. Devore
Publisher:
Cengage Learning
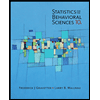
Statistics for The Behavioral Sciences (MindTap C…
Statistics
ISBN:
9781305504912
Author:
Frederick J Gravetter, Larry B. Wallnau
Publisher:
Cengage Learning
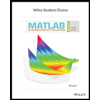
MATLAB: An Introduction with Applications
Statistics
ISBN:
9781119256830
Author:
Amos Gilat
Publisher:
John Wiley & Sons Inc
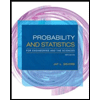
Probability and Statistics for Engineering and th…
Statistics
ISBN:
9781305251809
Author:
Jay L. Devore
Publisher:
Cengage Learning
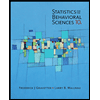
Statistics for The Behavioral Sciences (MindTap C…
Statistics
ISBN:
9781305504912
Author:
Frederick J Gravetter, Larry B. Wallnau
Publisher:
Cengage Learning
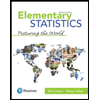
Elementary Statistics: Picturing the World (7th E…
Statistics
ISBN:
9780134683416
Author:
Ron Larson, Betsy Farber
Publisher:
PEARSON
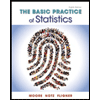
The Basic Practice of Statistics
Statistics
ISBN:
9781319042578
Author:
David S. Moore, William I. Notz, Michael A. Fligner
Publisher:
W. H. Freeman
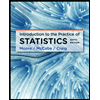
Introduction to the Practice of Statistics
Statistics
ISBN:
9781319013387
Author:
David S. Moore, George P. McCabe, Bruce A. Craig
Publisher:
W. H. Freeman