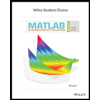
Concept explainers
8. Use the multiple regression output shown to answer the following questions.
The regression equation is:
Y = 9.77 0.775X1 + 0.062X2 - 0.221X3
Predictor | Coef | SE Coef | T | P |
Constant | 9.771 | 7.132 | 1.37 | 0.184 |
X1 | 0.7747 | 0.3201 | 2.42 | 0.025 |
X2 | 0.0642 | 0.1686 | 0.37 | 0.716 |
X3 | -0.2214 | 0.1730 | -1.28 | 0.214 |
S = 5.04975 R - Sq = 14.9% R - Sq (adj) = 2.8%
Analysis of Variance | |||||
Source | DF | SS | MS | F | P |
---|---|---|---|---|---|
Regression | 3 | 94.1 | 31.35 | 1.23 | 0.322 |
Residual Error | 21 | 535.5 | 25.5 | ||
Total | 24 | 629.6 |
(a) What is R2 for this model? Do we expect to increase, decrease, or remain the same if we eliminate the variable chosen in part (a)? What type of change in would indicate that removing the variable in part (a) was a GOOD idea? What type of change in would indicate that removing the variable in part (a) was a BAD idea?
b) What is the p-value for ANOVA for the original 3-predictor model?
Is the p-value most likely to increase, decrease, or remain the same if we eliminate the variable chosen in part (a)? What type of change in the p-value for ANOVA would indicate that removing the variable in part (a) was a GOOD idea? What type of change in the p-value for ANOVA would indicate that removing the variable in part (a) was a BAD idea?
c) What is the F-statistic from ANOVA for this model? Is the F-statistic most likely to increase, decrease, or remain the same if we eliminate the variable chosen in part (a)? What type of change in the F-statistic for ANOVA would indicate that removing the variable in part (a) was a good idea?

Trending nowThis is a popular solution!
Step by stepSolved in 2 steps with 2 images

- Listed below are systolic blood pressure measurements (in mm Hg) obtained from the same woman. Find the regression equation, letting the right arm blood pressure be the predictor (x) variable. Find the best predicted systolic blood pressure in the left arm given that the systolic blood pressure in the right arm is 90 mm Hg. Use a significance level of 0.05. Right Arm 103 102 96 76 76 Left Arm 174 167 149 148 148arrow_forwardThe money raised and spent (both in millions of dollars) by all congressional campaigns for 8 recent 2-year periods are shown in the table. The equation of the regression line is y = 0.942x +27.609. Find the standard error of estimate s, and interpret the result. 793.9 1042.3 957.7 1203.3 450.7 673.7 745.1 778.6 Money raised, x Money spent, y 734.8 1024.2 929.1 1160.6 448.6 697.9 735.7 751.2 Find the standard error of estimate s, and interpret the result. (Round to three decimal places as needed.) How can the standard error of estimate be interpreted? O A. The standard error of estimate of the money raised for a specific amount of money spent is about s, million dollars. O B. The standard error of estimate of the money spent for a specific amount of money raised is about s, million dollars.arrow_forwardListed below are systolic blood pressure measurements (in mm Hg) obtained from the same woman. Find the regression equation, letting the right arm blood pressure be the predictor (x) variable. Find the best predicted systolic blood pressure in the left arm given that the systolic blood pressure in the right arm is 90 mm Hg. Use a significance level of 0.05. Right Arm 101 100 92 75 75 O Left Arm 174 167 181 149 147 Click the icon to view the critical values of the Pearson correlation coefficient r The regression equation is y = + x. (Round to one decimal place as needed.) Given that the systolic blood pressure in the right arm is 90 mm Hg, the best predicted systolic blood pressure in the left arm is mm Hg. (Round to one decimal place as needed.)arrow_forward
- Listed below are systolic blood pressure measurements (in mm Hg) obtained from the same woman. Find the regression equation, letting the right arm blood pressure be the predictor (x) variable. Find the best predicted systolic blood pressure in the left arm given that the systolic blood pressure in the right arm is 80 mm Hg. Use a significance level of 0.05. Right Arm 100 99 93 77 77 Q Left Arm 174 168 148 148 146 Click the icon to view the critical values of the Pearson correlation coefficient r The regression equation is ŷ=+x. (Round to one decimal place as needed.) mm Hg. Given that the systolic blood pressure in the right arm is 80 mm Hg, the best predicted systolic blood pressure in the left arm is (Round to one decimal place as needed.) Data table Critical Values of the Pearson Correlation Coefficient r α = 0.05 α = 0.01 0.950 0.990 0.959 0.878 0.811 0.917 0.754 0.875 0.707 0.834 0.666 0.798 0.632 0.765 0.602 0.735 0.576 0.708 0.553 0.684 0.532 0.661 0.514 0.641 0.497 0.623 0.482…arrow_forwardThe following data shows memory scores collected from adults of different ages. Age (X) Memory Score (Y) 25 10 32 10 39 9 48 9 56 7 Use the data to find the regression equation for predicting memory scores from age. The regression equation is: Ŷ = 4.33X + 0.11 Ŷ = -0.11X + 4.33 Ŷ = -0.11X + 13.26 Ŷ = -0.09X + 5.4 Ŷ = -0.09X + 12.6 Use the regression equation you found in question 6 to find the predicted memory scores for the following age: 28 For the calculations, leave two places after the decimal point and do not round: Use the regression equation you found in question 6 to find the predicted memory scores for the following age: 43 For the calculations, leave two places after the decimal point and do not round: Use the regression equation you found in question 6 to find the predicted memory scores for the following age: 50 For the calculations, leave two places after the decimal point and do not round:arrow_forwardThe following table gives the data for the average temperature and the snow accumulation in several small towns for a single month. Determine the equation of the regression line, ŷ = bo + b₁x. Round the slope and y-intercept to the nearest thousandth. Then determine if the regression equation is appropriate for making predictions at the 0.05 level of significance. Critical Values of the Pearson Correlation Coefficient Average Temperature (°F) Average Temperatures and Snow Accumulations 42 31 24 45 38 18 33 21 25 9 12 27 7 15 22 30 13 20 37 Snow Accumulation (in.) 8arrow_forward
- An automobile rental company wants to predict the yearly maintenance expense (Y) for an automobile using the number of miles driven during the year () and the age of the car (, in years) at the beginning of the year. The company has gathered the data on 10 automobiles and run a regression analysis with the results shown below:. Summary measures Multiple R 0.9689 R-Square 0.9387 Adj R-Square 0.9212 StErr of Estimate 72.218 Regression coefficients Coefficient Std Err t-value p-value Constant 33.796 48.181 0.7014 0.5057 Miles Driven 0.0549 0.0191 2.8666 0.0241 Age of car 21.467 20.573 1.0434 0.3314 Use the information above to estimate the annual maintenance expense for a 10 years old car with 60,000 miles.arrow_forwardGiven the correlation coefficient 0.794 and the linear regression equation ŷ = 16.4 +1.58x, compute the coefficient of determination. p² Round to 4 decimal places. =arrow_forwardUse the shoe print lengths and heights shown below to find the regression equation, letting shoe print lengths be the predictor (x) variable. Then find the best predicted height of a male who has a shoe print length of 28.5 cm. Would the result be helpful to police crime scene investigators in trying to describe the male? Use a significance level of α=0.05. Shoe Print (cm) 29.1 29.1 31.8 31.9 27.5 Foot Length (cm) 25.7 25.4 27.9 26.7 25.1 Height (cm) 175.4 177.8 185.2 175.4 173.2 The best predicted height is enter your response here cm. (Round to two decimal places as needed.) Would the result be helpful? A. No, because the description would be the same regardless of shoe print length. B. Yes, because the description would be based on an actual shoe print length. C. Yes, because the correlation is strong, so the predicted…arrow_forward
- MATLAB: An Introduction with ApplicationsStatisticsISBN:9781119256830Author:Amos GilatPublisher:John Wiley & Sons IncProbability and Statistics for Engineering and th...StatisticsISBN:9781305251809Author:Jay L. DevorePublisher:Cengage LearningStatistics for The Behavioral Sciences (MindTap C...StatisticsISBN:9781305504912Author:Frederick J Gravetter, Larry B. WallnauPublisher:Cengage Learning
- Elementary Statistics: Picturing the World (7th E...StatisticsISBN:9780134683416Author:Ron Larson, Betsy FarberPublisher:PEARSONThe Basic Practice of StatisticsStatisticsISBN:9781319042578Author:David S. Moore, William I. Notz, Michael A. FlignerPublisher:W. H. FreemanIntroduction to the Practice of StatisticsStatisticsISBN:9781319013387Author:David S. Moore, George P. McCabe, Bruce A. CraigPublisher:W. H. Freeman
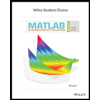
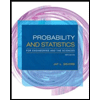
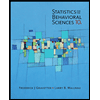
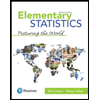
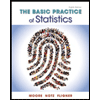
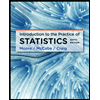