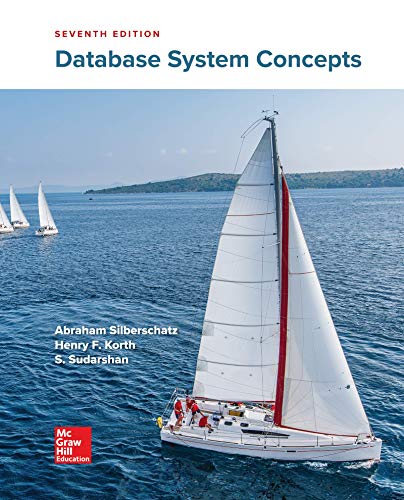
4-Clique Problem
The clique problem is to find cliques in a graph.
A clique is a set of vertices that are all adjacent - connected - to each other.
A 4-clique is a set of 4 vertices that are all connected to each other.
So in this example of the 4-Clique Problem, we have a 7-vertex graph. A brute-force
https://en.wikipedia.org/wiki/Clique_problem
You should read the Wikipedia page for the Clique Problem (and then read wider if need be) if you need to understand more about it.
Note that the Clique Problem is NP-Complete and therefore when the graph size is large a deterministic search is impractical. That makes it an ideal candidate for an evolutionary search.
For this assignment you must suppose that you have been tasked to implement the 4-clique problem as an evolutionary algorithm for any graph with any number of vertices (an n-vertex graph). The algorithm succeeds if it finds a 4-clique.
To achieve this task you would need to:
- develop an understanding of the problem and the nature of the search space
- figure out how to represent solutions and calculate fitness
- figure out ways to perform techniques such as mutation, crossover and termination
...and so the questions below are designed to take you through some of those processes.
You are expected to fill in any gaps in your knowledge of combinatorial mathematics through independent study.
1. Provide an algebraic expression, in terms of n, for the size of the phenotypic search space (the number of possible combinations of 4 vertices), for an n-vertex graph. You may assume that n ≥ 4.
2. Explain how a valid genotype can be represented as a partial permutation of integers, providing an example for the case n=7.
3. If we choose to represent each genotype as a partial permutation, then provide an algebraic expression, in terms of n, for the size of the genetic search space (the number of possible valid genotypes), in terms of n. You may again assume that n ≥ 4.
4. By comparing the answers to questions 1 and 3 above, briefly comment on how this reflects the mapping of genotypes to phenotypes.
5. Suppose a candidate solution p, where p is a phenotype consisting of 4 vertices. Suppose that minimum fitness occurs when no pair of vertices in p are connected, and maximum fitness occurs when all pairs of vertices in p are connected. Explain using pseudocode how to calculate the fitness F of p.
6. Suppose we decided to use swap mutation. Explain why that would be either a good or bad idea.
7. Give two reasons why a termination criterion that only halts when a valid solution is found is insufficient.
8. Suggest an additional termination criterion to fix the problem in question 7 above.

Trending nowThis is a popular solution!
Step by stepSolved in 6 steps with 3 images

- Consider the following edge-weighted graph G with 9 vertices and 16 edges: 90 Q6.1 Kruskal 40 50 80 130 110 70 S 150 60 30 10 120 140 20 100 List the weights of the MST edges in the order that Kruskal's algorithm adds them the MST. Your answer should be a sequence of 8 integers, with one space between each integer, 14arrow_forwardUse of Matching for Edge ColouringEdges in a matching can share the same colour since matching in a graph is defined as the collection of non-adjacent edges. As a result, we can create an algorithm based on this idea using the below stages. 1. Input: G = (V, E)2. Output: Minimal edge coloring of G3. color ← 04. while G = ∅$5. find maximal matching M of G6. color all M vertices with color7. G ← G − M8. color ← color + 1implementation of Pythonarrow_forward1. Input: An undirected weighted graph G = (V, E, w)2. Output: An MST T = (V, E) of G3. T ← Ø4. Sort edges of G in non-increasing order and place them in a queue Q.5. repeat6. Remove the first edge (u, v) from Q and add it to T if it does not form a cyclewith the edges edge that are already included in T .7. until there are n − 1 edges in T .We can use union-find data structure to find whether the two endpoints of theselected edge are in the same set (the current MST fragment). It can be shown thatthis algorithm is correct and has a time complexity of O(m log n) [1].Give Python Implementation of algoarrow_forward
- True or false helparrow_forwardKruskal's Algorithm: Kruskal's algorithm is a minimum-spanning-tree algorithm which finds an edge of the least possible weight that connects any two trees in the forest. This algorithm is a Greedy Algorithm. The steps to find a MST using this algorithm are as follows: Sort all the edges in non-decreasing order of their weight. Pick the smallest edge. Check if it forms a cycle with the spanning tree formed so far. If cycle is not formed, include this edge. Else, discard it. Repeat step2 until there are (V-1) edges in the spanning tree. Example with 10 vertices and 20 weighted edges:arrow_forwardCliques The Clique problem is finding the subsets of vertices all adjacent to each other. The maximum clique is the largest complete subgraph with every vertex connected by an edge. Cliques are studied in social networks, bioinformatics, and computational chemistry. Describe the brute force algorithm to find the maximum clique. What algorithm complexity class is the brute force solution to the clique problem? What time complexity is the brute force algorithm to find the maximum clique? How does the graph of a clique differ from the graph of a social network influencer? List the number of algorithm steps for graphs with the following vertex count: 10,100,1000arrow_forward
- We recollect that Kruskal's Algorithm is used to find the minimum spanning tree in a weighted graph. Given a weighted undirected graph G = (V , E, W), with n vertices/nodes, the algorithm will first sort the edges in E according to their weights. It will then select (n-1) edges with smallest weights that do not form a cycle. (A cycle in a graph is a path along the edges of a graph that starts at a node and ends at the same node after visiting at least one other node and not traversing any of the edges more than once.) Use Kruskal's Algorithm to nd the weight of the minimum spanning tree for the following graph.arrow_forwardShow what the final distance and previous array values will be after running the shortest path algorithm from class on the following graph, starting at vertex 2: 1 6 5 4 4 4 2 7 0 5 5 6 2 2 3 13 6arrow_forward11. For the given graph below, use the depth-first search algorithm to visit the vertices and write the sequence of the visit. Start from the vertex "A". At any given vertex, you will visit first the unexplored vertex that has least alphabetical order. с G E F Answer the sequence of visiting the vertices: -_arrow_forward
- Database System ConceptsComputer ScienceISBN:9780078022159Author:Abraham Silberschatz Professor, Henry F. Korth, S. SudarshanPublisher:McGraw-Hill EducationStarting Out with Python (4th Edition)Computer ScienceISBN:9780134444321Author:Tony GaddisPublisher:PEARSONDigital Fundamentals (11th Edition)Computer ScienceISBN:9780132737968Author:Thomas L. FloydPublisher:PEARSON
- C How to Program (8th Edition)Computer ScienceISBN:9780133976892Author:Paul J. Deitel, Harvey DeitelPublisher:PEARSONDatabase Systems: Design, Implementation, & Manag...Computer ScienceISBN:9781337627900Author:Carlos Coronel, Steven MorrisPublisher:Cengage LearningProgrammable Logic ControllersComputer ScienceISBN:9780073373843Author:Frank D. PetruzellaPublisher:McGraw-Hill Education
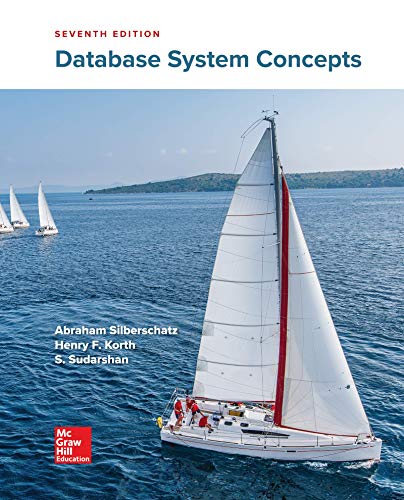
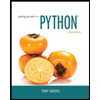
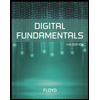
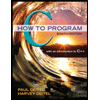
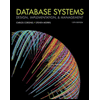
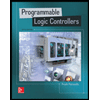