1. JMP output appears below for simple linear regression with data from the price, y (in $1000), of n = 28 Seattle home prices. The explanatory variable is the total number of square feet in the home. VOResponse Price ($000) v Regression Plot 600 500 400 300 200 1000 1500 2000 2500 3000 3500 DDistributions Square Feet Square Feet Summary of Fit RSquare RSquare Adj Root Mean Square Error Mean of Response Observations (or Sum Wgts) 0.560503 0.543599 356.8214 28 v Analysis of Variance Sum of 1000 1500 2000 2500 3000 3500 Source DF Squares Mean Square F Ratio Model 249200,64 249201 33.1585 Prob > F Summary Statistics Error 26 C. Total 27 <.0001* Mean 1923.1071 653,11574 123.42727 Std Dev - Parameter Estimates Std Err Mean Term Estimate Std Error t Ratio Prob>lt| Upper 95% Mean Lower 95% Mean 1669.8553 2176.359 1.43 0.1653 Intercept Square Feet 0.1470966 0.025545 73.938964 51.78554 5.76 <.0001" 28 Price ($000) 2. For the same houses from Question 1, a multiple regression model is now used to predict the price y (in $1000) of the n = 28 Seatle home prices based on two more explanatory variables in addition to square feet. The explanatory variables are then X1 = square feet ; Price/Square Feet; Bathrooms (Number of bathrooms). B1, B2 and B3 are the corresponding parameters in the model. For all the testing problem hereby, set significance level a = 0.05. Response Price ($000) Summary of Fit RSquare RSquare Adj Root Mean Square Error Mean of Response Observations (or Sum Wgts) 0.9534 0.947575 29.38132 Response Price ($000) Summary of Fit 356.8214 28 RSquare RSquare Adj Root Mean Square Error Mean of Response Observations (or Sum Wgts) 0.131114 0.097695 v Analysis of Variance 121.8935 Sum of 356.8214 Source DF Squares Mean Square F Ratio 28 Model 423883.82 141295 163.6752 Analysis of Variance Error 24 20718.29 863 Prob > F C. Total 27 444602.11 <.0001* Sum of Source DF Squares Mean Square F Ratio v Parameter Estimates Model 58293.36 58293.4 3.9234 Term Estimate Std Error t Ratio Prob>|t| 14858.0 Prob > F 0.0583 Error 26 386308.75 C. Total Intercept Square Feet 0.1895693 0.011048 Price/Sq Ft -371.4508 45.67288 -8.13 <.0001* 27 444602.11 17.16 <.0001* v Parameter Estimates 12.51 <.0001* -0.33 0.7411 1961.0355 156.728 Bathrooms -3.798639 11.36416 Term Estimate Std Error t Ratio Prob>|t| Intercept Price/Sq Ft 1089.7999 550.1965 v Effect Tests 149.87283 106.9894 1.40 0.1731 1.98 0.0583 Sum of Nparm F Ratio Prob > F Effect Tests Source DF Squares Square Feet Price/Sq Ft Bathrooms 254169.70 294.4294 <.0001* Sum of 135151.41 156.5590 <.0001* Source Nparm DF Squares F Ratio Prob > F 1 96.45 0.1117 0.7411 Price/Sq Ft 58293.360 3.9234 0.0583
Correlation
Correlation defines a relationship between two independent variables. It tells the degree to which variables move in relation to each other. When two sets of data are related to each other, there is a correlation between them.
Linear Correlation
A correlation is used to determine the relationships between numerical and categorical variables. In other words, it is an indicator of how things are connected to one another. The correlation analysis is the study of how variables are related.
Regression Analysis
Regression analysis is a statistical method in which it estimates the relationship between a dependent variable and one or more independent variable. In simple terms dependent variable is called as outcome variable and independent variable is called as predictors. Regression analysis is one of the methods to find the trends in data. The independent variable used in Regression analysis is named Predictor variable. It offers data of an associated dependent variable regarding a particular outcome.
Site images for background.
In terms of variables Xi and parameters Bi, write the null and alternative hypotheses for testing whether, after including Price/Square Feet(x2) in the model already, the further incorporation of the other 2 explanatory variable (x1,x3) adds any useful information for expliaining price y. Also give the value of the F statistic and its degrees of freedom (df).



Trending now
This is a popular solution!
Step by step
Solved in 2 steps with 3 images

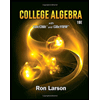
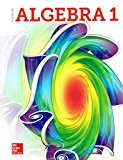
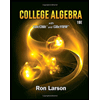
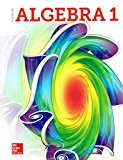
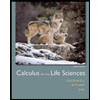
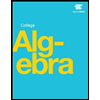
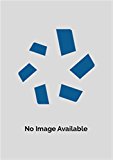