IDS-2023-A3-Final Project
pdf
keyboard_arrow_up
School
Swinburne University of Technology *
*We aren’t endorsed by this school
Course
10001
Subject
Computer Science
Date
Apr 3, 2024
Type
Pages
13
Uploaded by MinisterOkapiPerson1098
1 Swinburne University of Technology Department of Computing Technologies COS60008 Introduction to Data Science Semester Sept
2023 – Final Project Due: 23:59 Sunday
10 December
2023 Introduction This is an individual assignment and worth 40% of your final grade. You will be allocated a unique randomly generated dataset designed to help you demonstrate your individual skills in a data science process. Specifically, the steps of Data (3) Preparation, (4) Exploration, (5) Modelling, and (6) Communication. The initial tasks will be familiar to you from
previous assignments and classwork. Later tasks involve modelling and then prediction using your model. You will
communicate the outcomes of your work in notebook output and a short video.
READ ALL OF THIS DOCUMENT BEFORE YOU START! J
NOTE: We strongly
recommend that you read the entire Final Project details (this document) before
you start the work. If you read through this document first, you will be ready to make good choices. Be prepared! Academic Integrity The submitted work must be your own work, and any parts that are not created by yourself must be properly referenced. Plagiarism is treated very seriously at Swinburne. It includes submitting the code and/or text copied from other students, the Internet or other resources without proper reference. Allowing others to copy your work is also plagiarism. Please note that you should always create your own work even if you have very similar ideas with other students. Plagiarism detection software will be used to check your submissions. Severe penalties (e.g., zero mark) will be applied in cases of plagiarism. For further information, please refer to the relevant section in the Unit Outline under the menu “Syllabus” in Canvas and the Academic Integrity information at https://www.swinburne.edu.au/current-
students/manage-course/exams-results-assessment/plagiarism-academic-integrity/
. Moderation: Your work will be analysed to see if it is similar to other students. This analysis may occur multiple times, and after your initial mark has been given to you during the semester. Your results may be moderated at any point in the semester to include later analysis results.
2 Domain, Questions & Data Details The data domain is completely artificial and generated uniquely for each student, based on real-world principles and relationships. You will prepare, explore and model this data. We consider (Step 1: Problem Formulation) agricultural data that has (hypothetically) been collected for a number of location and crop types recorded over a period of years. Although the number of data points is not large, you have been asked to investigate the relationship between various attributes and outcomes to see if there are general relationships that could be modelled, and then used to improve or predict future crop choices and outcomes. Some of the specific questions that you need to investigate, and some that you may choose to investigate, are presented in later tasks. As you have been provided (Step 2: Acquisition) with a unique set of data files just for you, you do not need to capture the data yourself. You will have to deal with some typical issues of preparing historical data. The data files were emailed to you as three (3) separate .csv files within a single zip file. You will need to prepare (Step 3: Preparation) this data into a single “data_clean.csv” file and follow the requirements stated later in Task 1. When you complete Task 1, your clean output data should have the features described in Table 1. Table 1. The required clean data output columns details for each attribute. Attribute Name Role Type Description Units / Format Year Feature Ordinal (Quantity) The calendar year of the data Integer Location Feature Nominal (Categorical) String value Year Irrigation Feature Nominal (Categorical) Was the field irrigated? Y/N Crop Type Feature Nominal (Categorical) What type of plant (crop) was used in the location , i.e. “Wheat”, “Soy” etc String Avg Min Temp *C Feature Continuous Daily minimum temperature averaged over the growing season Celsius Yr Rain mm Feature Continuous Average rainfall for each year as recorded at the location mm Heat Wave Feature Nominal (Categorical) Was there a heat wave (a critical number of consecutive days above a standard temperature) in the growing season? Y/N Dry Spell Feature Nominal (Categorical) Was there a dry spell (a critical number of consecutive days with no-rain) in the growing season? Y/N Cold Wave Feature Nominal (Categorical) Was there a cold wave (a critical number of consecutive days below a standard temperature) in the growing season? Y/N Wet Spell Feature Nominal (Categorical) Was there a wet spell (a critical number of consecutive days with rain) in the growing season? Y/N Crop Damage Feature/Target Nominal (Categorical) Was there crop damage this season? This could be due to pests or seasonal variations. (1) Y/N Yield Feature/Target Continuous ( Floating point value of the average yield for the crop per unit size of the field. Unknown (2) Observer Feature Ordinal Person who recorded the String value String (1)
The exact type of damage and the reason for crop damage is not recorded. It might be due to external factors (disease, bugs, fires), but might correlate to temperature or rain data. Excessive hot or cold might damage crops. Extended wet or dry periods during the growing season might stunt growth. When there is “Crop Damage” this is likely reflected in the yield being lower than historical trends. (2)
The exact units of the “Yield” have not been recorded by the client but the investigation will continue without it. It might be “bushels per acre” or a similar production metric.
3 Table 2. The unique data files provided in the zip file to each student. These need to be processed to create the clean data. File Name Data Details data_1_env.csv year, location, temp (*F), rain (inches), heat wave, dry spell, cold wave, wet spell data_2_env.csv year, location, temp (*C), rain (mm), heat wave, dry spell, cold wave, wet spell data_3_crop.csv year, location, irrigation, crop type, crop damage, yield, observer Data Notes: •
The reason for the two “env” files is that older temperature and rainfall data was recorded in degrees Fahrenheit and inches. This is stored in data_1_env.csv and will need its values converted so that if can be combined with later values from data_2_env.csv which already has the wanted degrees Celsius and millimetre (mm) units. •
The crop observations were stored in a separate file (data_3_crop.csv) by staff members each year (“observer”). This data will need to be matched with the combined environment data (data_1_env.csv and data_2_env.csv files). There should be a unique crop observation entry for each valid year and location in the “env” data, but check if this is the case. •
It is known that some values are missing, but it is believed this only occurred accidentally when some entries were partially duplicated. You should try to confirm this, and if it has occurred, deal with missing data appropriately. •
It is possible that some of the continuous values are outside of the sensible expected range. You need to confirm if this has occurred or not and deal with it appropriately if needed. What is “sensible” has not been indicated so you will need to make and record appropriate assumptions for this. General Requirements This section contains the general requirements which must be met by your submitted assignment work. Marks will be deducted if you fail to meet any of the following general requirements. •
Use Python 3 & Notebook: You must complete code tasks using the Jupyter Notebook format with a Python 3 kernel. •
Use a single notebook file per tasks: Tasks 1 to 4 must each have a SINGLE notebook file with the exact required filename. See each task for details. •
Include the header section in all notebook files: At the start of each notebook file, include a header section as a markdown cell. See each task for details.
•
Use cells for sub-tasks:
Create appropriate notebook cells for sub-tasks within each task. •
Don’t have a single cell with too much code that combines different sub-tasks. •
Don’t have a single cell for every single line of python code.
•
It is your job to communicate effectively. •
Code Comments:
You must include code-level comments in your notebook file to explain the key
parts of your code.
•
If you do not have code comments that support your code answer, your mark will be reduced even if the code is correct. (Note that this is for KEY parts of your work, not every part of it.)
•
It is valuable to make your code comments unique so that your work is not like other students when assessed. Put things in your own words!
•
You do NOT have to explain every single line of code or things that are very easy for another programmer to understand.
•
Graphs are Clear and Labelled:
All significant plots or graphs, such as those used to answer questions in Task 2, should have appropriate titles, axis labels and legends. •
Follow Tasks Instructions: You must follow the instructions exactly as given in each task and complete them.
•
Submit Correctly:
You must follow the details specified in the tasks and submit the files requests. Make sure your files are named exactly as specified. (This also helps with marking scripts when used.)
Your preview ends here
Eager to read complete document? Join bartleby learn and gain access to the full version
- Access to all documents
- Unlimited textbook solutions
- 24/7 expert homework help
4 Task 1 – Data Loading & Preparation (20%) In this task you need to load your unique three data files, and create a clean and merged version of the data ready for later task use. You will upload your code to achieve this as a notebook file and HTML version saved from your Jupyter-lab session. You will also upload your final cleaned data to Canvas. Note: Details of the provided data files were presented in the section “Domain, Questions & Data Details” earlier in this document. You will need to consider the details there to complete this task. Exact steps needed are not given here. Use this task to demonstrate that you know what to do and what is appropriate. Create a Jupyter notebook file (“
task_1.ipynb
”) for this task. Include a markdown
header section (not python comments) in the file with the following details, with your name and other student details. Note that it includes the “Task 1” as a level 2 heading. Update this to suit each task. Task 1 Checklist:
•
Create
the appropriate notebook code file with the exact filename of “task_1.ipynb”. •
Include the required header
section and update with your specific student details. •
Load
the three source data files you have been provided. •
Correctly clean
the data, convert
as needed, and merge
into a single useful collection. •
Use markdown headings
(required) to organise the major steps of what you do so that they explain what you are doing. (i.e. “## Converting temperature values from F to C”). •
You might want to look for … duplicate rows, missing values, out of range values, inconsistent string values (labels), etc. ? •
You may want to use plots
as well as summary and descriptive
methods to identify issues. You can (should) include these in your code, but only include them if they make sense or give you an answer about how to clean, prepare or merge the data. (Leave detailed exploration for Task 2.) •
Use appropriate python code tools to identify what to fix. (Don’t perform this work in other non-python tools. You are to show off your python data science skills.) •
Include code comments
to state what you are doing and why
it is useful. •
Save
the clean data to file, in the same location
as the notebook file (no sub-folders or absolute paths), in csv format, with a header
row, and named exactly clean_data.csv
. •
Upload
the required files to Canvas for this task. General •
Do include markdown headings to explain what you are doing (as a data science task or goal). •
Do include useful comments that would help another data scientist understand your code does. •
Do NOT include irrelevant code or comments in your code or html file. Clean up your code before you submit to remove code or comments that are not needed or do not support the task requirements. •
Do NOT include irrelevant plots that have no purpose. If you have plots but don’t have a comment to explain why they are included, or the reason is poor, there will be a deduction. •
Do NOT use Excel or other similar tools to identify and then fix data issues. Show that you can do this using the python code tools only (even if you first get a hint using other tools to inspect that data first). Submit to “Assignment 3 - Task 1” in Canvas the following files: •
Notebook file: “task_1.ipynb”
•
HTML version of notebook session: “task_1.html”
•
Cleaned csv file: “clean_data.csv”
5 Tip: The provided csv files have a header row. Make sure you read this in correctly and use the information. Tip: Using the pandas “unique()” method can help with string values to identify what is present and compare that to what you expect should actually be there. For strings, you can then use the remap function to replace and make all strings/categorical values consistent. Tip: Use the DataFrame describe() method to get a quick understanding of values present in a column/feature. You can use this on individual files before merging and compare. Also, DataFrame.groupby() is very handy – use it. Tip: Use “preliminary” scatter plots and histograms to get a quick top-level understanding of the distribution of values, clusters and outlier values. You do not need to label everything as you would in a report, but it is a good habit to be in. The groupby() method is also useful here as well to select particular data features to inspect.
6 Task 2 – Exploration (30%) We are pretending that a number of questions have been collected from the client by asking them about what they want to know about from the historical information collected, and how they want to use the data in the future. All the questions are listed in the next section of this document. You should attempt to answer these questions. Some questions are relatively easy and “straight-forward” and most students will be able to do all of these. Other questions you may not be able to answer with your current skills and knowledge (and that’s okay). Some questions may be impossible to answer with the data available, or the answer may be “no” when asked. You do not need to answer all questions. (In real situations, questions cannot always be answered, but you should ideally be able to explain why they cannot be answered, or at least not yet.). There are 30 marks for this task. •
Answer all the basic “Baseline” questions first which will contribute approximately 20 marks for this task (~20 questions), with marks depending on the quality of your answers. •
Then, select a number of additional questions, for the remaining ~10 marks, that you are able to answer. Marks will be based on the number and complexity of questions and answers. For example, 10 simpler questions at ~1 mark each, or 5 intermediate questions at ~2 marks each, or a complex question worth more with other simpler questions. •
Your choice of appropriate questions is part of your mark for this task. Create a Jupyter notebook file (“
task_2.ipynb
”) for this task. Include the appropriate markdown
header section cell with similar details to Task 1 but updated for this task. Make sure it includes the text “Task 2 - Exploration” as a level 2 heading. You should document your analysis steps and answers to the questions in the notebook file. (There is no separate report file for this assignment.) It is up to you to clearly present each question you are answering and then your answer. For example (suggestion), the image below shows using a markdown cell and a level 3 heading to state one of the questions, then in a python cell with a code comment to explain (if needed) what will be done and then code to (try to) get the answer. Clearly state your answer so a reader can easily identify it (as part of code output is preferred). The order you use to attempt or present the questions is up to you. In general, use different cells for different questions (unless they are closely related.) You can have a question in the file without an answer. Just make it clear you have not answered it yet. Task 2 Checklist:
•
Create
the appropriate notebook code file with the exact filename of “
task_2.ipynb
”. •
Include the required header
section and update with your specific student details. •
Load
the clean data file you created in Task 1. (Don’t re-do the step.) •
Answer
questions: Clearly state each question (heading?) and then your attempt/answer. •
The order is up to you and the exact format is up to you. •
Answers must be in the notebook file. •
You do not need to answer every question. •
Tip: Tables (with results) are sometimes more useful then plots. •
Save
the notebook file and a HTML version of the work. •
Upload
the two required files to Canvas for this task. General •
Do include useful comments that would help another data scientist understand your code does. •
Do NOT include irrelevant code or comments in your code or html file. Clean up your code before you submit to remove code or comments that are not needed or do not support the task requirements. •
Do NOT include irrelevant plots that have no purpose. If you do there will be a deduction. •
Do NOT use Excel or other similar tools to identify answers to the questions. Show that you can do this using the python code tools only (even if you first get a hint using other tools to inspect that data first). Submit to “Assignment 3-Task 2” in Canvas the following files: •
Notebook file + HTML version, task_2.ipynb
and task_2.html
Your preview ends here
Eager to read complete document? Join bartleby learn and gain access to the full version
- Access to all documents
- Unlimited textbook solutions
- 24/7 expert homework help
7 Task 2 Questions Baseline Properties & Count Questions (Answer All. ~20 marks, ~1 mark each): •
Determine the following basic details about the data. (Presented as a table would be ideal, but not required): o
What is the “Year” range (max, min) of the data collected? o
Are there any missing years in the data? If so, which? o
What are the “Locations” (values) in the data? o
What are the “Crop Types” (values) in the data? o
What is the range (min, max) of the temperature values? o
What is the range (min, max) of the rainfall values? •
Have all locations been used every year of data collection? •
Do locations always have the same “Irrigated” value or does it change over the years? •
What is the total number of valid data entries (rows of data) you are expecting based on years of data collected and number of site locations? o
Is this the number you actually have? If not, why? •
Explore the “Crop” data: o
What is the total occurrence (count used) of each crop type in the sample? (Table?) o
What is the break-down (count used) of “crop type” at each location? (Plot? Table?) o
What is the total yield for each crop type over the entire sample? (Yield total by type?) o
Has there been any changes in crop type usage over time? (Trends? Uniform? Plot?) •
Staff members making obversions o
Who was the longest serving staff member(s) making observations? §
How many years and at what location? o
Did any staff member join again after they first performed the role? General Relationships (~2-5 marks each): •
Is there a relationship between temperature and yield? (Consider “in general” is acceptable.) o
Is there a different between the irrigated and non-irrigated crops? o
Note: The assumption is “yes there would be – not too cold and not too hot” and that “irrigated” locations would do better than non-irrigated locations. o
Tip: A plot of the yield (y-axis) compared to the temperature (x-axis) is a good start. •
Is there a relationship between rainfall and yield? (Consider “in general” is acceptable.) o
Is there a different between the irrigated and non-irrigated crops? o
Note: The assumption is “yes there would be – not too wet and not too dry” and that “irrigated” are not as affected by the rainfall as non-irrigated location would be. o
Tip: A plot of the yield (y-axis) compared to the rainfall (x-axis) is a good start. •
Is there a difference in yield expectations for specific crop types? o
Compare both the general outcomes as well as the relationship at specific locations. o
Note: The assumption is this should be a clear “yes” – different plant types produce very different quantities of their unique product, but the data should show this o
Tip: Try a scatter plot (x=year, y=yield), with coloured labels for each crop type. •
Is there a difference in yield trends (over time) at different locations? •
What is the location that has performed best over the entire collection time? •
What is the crop type that has performed best over the entire collection time? Event Relationships (~2-5 marks each): •
Show appropriate plots of Dry Spell and Heat Wave events to determine: o
Which locations have had the most and least events, respectively? o
Is there a relationship to temperature, rainfall, yield or year? •
Show appropriate plots of Wet Spell and Cold Wave events. o
Which locations have had the most and least events, respectively? o
Is there a relationship to temperature, rainfall, yield or year? •
Show appropriate plots of Crop Damage events o
Which location has had the most events? o
Is there a relationship to temperature, rainfall, yield or year? •
Can you find a way to present correlation between multiple events, in a clear table or matrix?
8 Temporal (Year) Relationships (~2-5 marks each): •
Is there an observable relationship between crop yield and the year? o
For all locations combined? o
At each location? •
Is there an observable relationship between rain data and the year: o
For all locations combined? o
At each location? •
Is there an observable relationship between temperature and the year? o
For all locations combined? o
At each location? •
Is there a relationship between Crop Damage and the year o
For all locations combined? o
At each location?
9 Task 3 – Test & Train Data Preparation (5%) In Task 4 you will be asked to create models of the data. In this task you will need to create a short notebook file that can generate the appropriate test and train data files, and save them to an appropriate location. As before, create a notebook file for this task with an appropriate markdown header section. Name the file “
task_3.ipynb
” and the saved HTML output as “
task_3.html
”. Load in the “
clean_data.csv
” you generated in Task 1. To prepare the data, first remove any columns that you decide you don’t need for your modelling in Task 4. Make sure you comment in your code “why” you decided to drop a column. For example, you can drop the “Observer” column as we will assume it has no bearing on “Yield” or “Crop Damage” targets. If you haven’t already done so for previous tasks, convert any categorical values that might be used for a model to numerical values. For example, convert (i.e. using the map() function) the “Y” and “N” values to 1.0 and 0.0 values. Similarly, for “Crop Type” and “Location”, you can convert a column type to “category” and replace the original string values with cat codes, or just use mapping again. There are two potential target columns - the crop “Yield” and “Crop Damage”. The outcome of a model for each respectively would be a numerical estimate (yield prediction) and a Boolean “Y” or “N” (represented as 1 or 0 perhaps). We want to create two datasets, one for each target situation. Dataset (a) should include all relevant columns except “Yield” in X
(drop from X) and separate the “Yield” column only as the target y
. The second dataset (b) should include all relevant columns (include “Yield” this time but drop “Crop Damage” from X
) in X,
and separate the “Crop Damage” column as its target y
. Then for each dataset, create a split 80:20 for training and testing, use a set “
random_state
” value so that it is repeatable, and save the data to the following respective filenames in the same location as the notebook file. Take care to use the exact upper or lowercase filenames (capital “X”, lower “y” etc) as specified. Dataset Usage File Name (a) “Yield” is target y Training data (80%)
a_X_train.csv a_y_train.csv Testing data (20%)
a_X_test.csv a_y_test.csv (b) “Crop Damage” is target y Training data (80%)
b_X_train.csv b_y_train.csv Testing data(20%)
b_X_test.csv b_y_test.csv Note: You do not need to upload these files to canvas, but your notebook script must generate the files and save them to the same folder where the notebook file is the notebook is run. Task 3 Checklist:
•
Create
the appropriate notebook code file with the exact filename of “
task_3.ipynb
”. •
Include the required header
section and update with your specific student details. •
Load
the clean data file you created in Task 1. (Don’t re-do the steps. Just load the clean data.) •
Convert
and remap
values if needed. •
Drop
columns if not appropriate for modelling in Task 4 •
Create
(a) and (b) datasets, then create
training and testing splits for each and save
to file. •
Save
the notebook file and a HTML version of the work. •
Upload
the two required files to Canvas for this task. Submit to “Assignment 3-Task 3” in Canvas the following files: •
Notebook file + HTML version, “
task_3.ipynb
” and “
task_3.html
”
Your preview ends here
Eager to read complete document? Join bartleby learn and gain access to the full version
- Access to all documents
- Unlimited textbook solutions
- 24/7 expert homework help
10 Task 4 – Modelling & Assessment (25%) We now want to see if we can model and predict either the “Yield” outcome or the “Crop Damage” outcome using the historic data. You may have already seen an indication of an underlying relationship in the data from the previous exploration you have done for Task 3, depending on the questions you selected to explore. If so, it is recommended you select this dataset to model. Select one of the datasets to model – (a) or (b). Then load the appropriate four data files and make sure the data is ready for model training and assessment (testing). Select, train and evaluate (test) at least 5 different models and present a comparison of the results: 1.
Select 2 Learning Algorithms (L): Select two (2) different learning algorithms from the scikit-learn package that might be appropriate for modelling the dataset you have selected. For example, you might select K-Nearest Neighbours (K-NN) and Artificial Neural Networks (ANNs). Both have various configuration options that could be adjusted. 2.
Create 5 Algorithm + Configuration (M) Create a total of at least five (5) different algorithm and configuration combinations to try modelling with. For example, two different configurations of a KNN (different N values, say N=6 and N=10) and three ANN configurations (different hidden layer value, say 3, 7, 10 hidden neurons). 3.
Assess Each Algorithm + Configuration:
For each of the 5 “algorithm + configuration” combinations, train on the training data (multiple times if appropriate) and then finally assess the trained model using the test data that the model has not seen before. You will need metrics to compare the model to the test data. Present the assessment results as a suitable table so that it is easy to identify which approach worked best.
4.
Select the Best
Select the algorithm + configuration approach that worked the best based on the data you collected. Note: If you want to demonstrate a more sophisticated assessment approach, and understand it, you can implement K-Fold Cross Validation for each combination in M. Also, as some learning algorithms are stochastic (i.e. random, such as ANNs) you may need try training each multiple times and see how well they work on average – a single training instance may not be appropriate to say if the technique or model will work. Task 4 Checklist:
•
Create
the appropriate notebook code file with the exact filename of “
task_4.ipynb
”. •
Include the required header
section and update with your specific student details. •
Decide what dataset you will try to model. •
Load
the test and train dataset for the chosen model. •
Decide
on two (2) different learning algorithms to try. •
Create
five (5) unique Algorithm + Configurations to test. •
Assess each approach and present results appropriately. •
Save
the notebook file and a HTML version of the work. •
Upload
the two required files to Canvas for this task. Submit to “Assignment 3 - Task 4” in Canvas the following files: •
Notebook file + HTML version, “
task_4.ipynb
” and “
task_4.html
”
11 Task 5 – Communication (20%) Prepare a video presentation of, at most, 3 minutes in duration to showcase the methods you used and report the main findings of your exploration and modelling. Note that in such a short time it is not possible to talk about everything in detail! Your goal is to communication the main details and key outcomes very briefly so that the audience would know what you found out. Video format: •
3 minutes (or less) duration •
Voice-over audio of your own voice. •
Resolution is small ~ SD only (640x480, 720x480), mp4. Canvas may not accept large file sizes. •
Don’t try to include very small text or high detail graphics. In your presentation •
Show the main things you did to prepare the data •
Show the key findings from your exploration •
Show the models you selected •
Show the model validation result •
Show the best model based on your results Important Tip / Advice: 3 mins x 60 seconds = 180 seconds. 180 seconds / 5 topics = 36 seconds each topic! So, remember it is only the “high-lights” or “main outcomes” you want to mention, and you do NOT have time for more than that! J
Submit to “Assignment 3 - Task 5” in Canvas the following files: •
Your video to file named “
task_5.mp4
”
12 Submission Requirements See the Canvas Assignment details for the specific due date and time for this assignment. Submission for this assignment is divided into separate Assignments in Canvas for each Task. Make sure you submit the correct files, and only the expected files, to Canvas. Assignments submitted after the specified date and time are subjected to late submission penalties. For detailed information, refer to the relevant section in the Unit Outline under the menu “Syllabus” in Canvas. Note: Please make sure to clean your code before submission to remove all unnecessary code. Make sure you “Restart and Run All” and that you see all the data printed and all the graphs displayed as expected in your file. Make sure saved “HTML” files have been saved from the JupyterLab interface only and present correctly in a browser. Please do NOT submit any other unnecessary files. Marks will be deducted if you do.
Extensions will only be permitted in exceptional circumstances. You should always backup your code and other assignment-related documents frequently to avoid potential loss of progress. Note that any accidental loss of progress, working while studying, and/or a heavy load of assignments will not be accepted as the exceptional circumstances for an extension. For detailed information, please refer to the relevant section in the Unit Outline under the menu “Syllabus” in Canvas.
Your preview ends here
Eager to read complete document? Join bartleby learn and gain access to the full version
- Access to all documents
- Unlimited textbook solutions
- 24/7 expert homework help
13 Assessment Criteria The table below shows task number, summary details and points awarded when your work is assessed. A detailed rubric is available in the Canvas unit website under “Assignments” > “Assignment 3” for each specific task. See there for complete details. Deductions will occur if General Requirements, as stated earlier, are not followed. Assessment Task, Summary Details and Points. Task Summary Details Points 1 Data Loading & Preparation Created appropriate notebook file with format and header details. Supplied data is correctly loaded and prepared including handling of duplicates, missing values, and value conversions. Data combined correctly. Appropriate column headings used. Some plots and descriptive methods used to identify and correct for issues. Clean data is saved and uploaded. No excessive or unnecessary notebook content and effective communication demonstrated. 20 2 Exploration Created appropriate notebook file with format and header details. Loads clean data from Task 1. Explores and presents and answers to an appropriate number of the provided questions. Questions and answers formatted appropriately (headings). All baseline questions answered, some intermediate and complex questions answered or attempted. Evidence of understanding data and question limitations shown in work.
30 3 Test & Train Data Preparation Created appropriate notebook file with format and header details. Loads clean data from Task 1, converts, remaps, drops values and columns as needed. Creates dataset (a) and (b), splits datasets into test and train sets and saves to file. Files named as required. Split is seeded as required.
5 4 Modelling & Assessment Create appropriate notebook file with format and header details. Appropriate dataset selected and loaded from files (from Task 3). Learning algorithms selected, configured, trained and assessed. Evidence of appropriate comparison and selection.
25 5 Communication Required topics are covered (~5x3), presented in the correct format with voice over (~5). 20 Total 100
Related Documents
Recommended textbooks for you
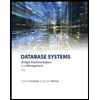
Database Systems: Design, Implementation, & Manag...
Computer Science
ISBN:9781305627482
Author:Carlos Coronel, Steven Morris
Publisher:Cengage Learning
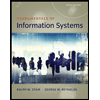
Fundamentals of Information Systems
Computer Science
ISBN:9781337097536
Author:Ralph Stair, George Reynolds
Publisher:Cengage Learning
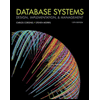
Database Systems: Design, Implementation, & Manag...
Computer Science
ISBN:9781337627900
Author:Carlos Coronel, Steven Morris
Publisher:Cengage Learning
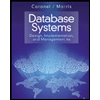
Database Systems: Design, Implementation, & Manag...
Computer Science
ISBN:9781285196145
Author:Steven, Steven Morris, Carlos Coronel, Carlos, Coronel, Carlos; Morris, Carlos Coronel and Steven Morris, Carlos Coronel; Steven Morris, Steven Morris; Carlos Coronel
Publisher:Cengage Learning
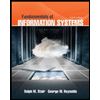
Fundamentals of Information Systems
Computer Science
ISBN:9781305082168
Author:Ralph Stair, George Reynolds
Publisher:Cengage Learning
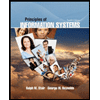
Principles of Information Systems (MindTap Course...
Computer Science
ISBN:9781285867168
Author:Ralph Stair, George Reynolds
Publisher:Cengage Learning
Recommended textbooks for you
- Database Systems: Design, Implementation, & Manag...Computer ScienceISBN:9781305627482Author:Carlos Coronel, Steven MorrisPublisher:Cengage LearningFundamentals of Information SystemsComputer ScienceISBN:9781337097536Author:Ralph Stair, George ReynoldsPublisher:Cengage LearningDatabase Systems: Design, Implementation, & Manag...Computer ScienceISBN:9781337627900Author:Carlos Coronel, Steven MorrisPublisher:Cengage Learning
- Database Systems: Design, Implementation, & Manag...Computer ScienceISBN:9781285196145Author:Steven, Steven Morris, Carlos Coronel, Carlos, Coronel, Carlos; Morris, Carlos Coronel and Steven Morris, Carlos Coronel; Steven Morris, Steven Morris; Carlos CoronelPublisher:Cengage LearningFundamentals of Information SystemsComputer ScienceISBN:9781305082168Author:Ralph Stair, George ReynoldsPublisher:Cengage LearningPrinciples of Information Systems (MindTap Course...Computer ScienceISBN:9781285867168Author:Ralph Stair, George ReynoldsPublisher:Cengage Learning
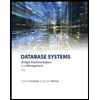
Database Systems: Design, Implementation, & Manag...
Computer Science
ISBN:9781305627482
Author:Carlos Coronel, Steven Morris
Publisher:Cengage Learning
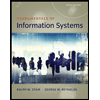
Fundamentals of Information Systems
Computer Science
ISBN:9781337097536
Author:Ralph Stair, George Reynolds
Publisher:Cengage Learning
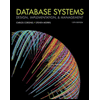
Database Systems: Design, Implementation, & Manag...
Computer Science
ISBN:9781337627900
Author:Carlos Coronel, Steven Morris
Publisher:Cengage Learning
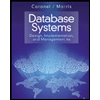
Database Systems: Design, Implementation, & Manag...
Computer Science
ISBN:9781285196145
Author:Steven, Steven Morris, Carlos Coronel, Carlos, Coronel, Carlos; Morris, Carlos Coronel and Steven Morris, Carlos Coronel; Steven Morris, Steven Morris; Carlos Coronel
Publisher:Cengage Learning
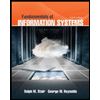
Fundamentals of Information Systems
Computer Science
ISBN:9781305082168
Author:Ralph Stair, George Reynolds
Publisher:Cengage Learning
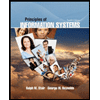
Principles of Information Systems (MindTap Course...
Computer Science
ISBN:9781285867168
Author:Ralph Stair, George Reynolds
Publisher:Cengage Learning