lab09-sol
pdf
School
Concordia University *
*We aren’t endorsed by this school
Course
6721
Subject
Computer Science
Date
Dec 6, 2023
Type
Pages
11
Uploaded by CaptainMaskWildcat29
COMP 6721 Applied Artificial Intelligence (Fall 2023)
Lab Exercise #09: Knowledge Graphs, Part II
Solutions
Question 1
Analyze and reason about RDF Schema class hierarchies and property inheri-
tance in a theoretical context. This exercise focuses on your understanding of
the concepts without the need to write RDF triples or code.
a) Describe the relationships and reasoning within a class hierarchy that in-
cludes
Person
,
Employee
,
Manager
, and
Intern
, where
Manager
and
Intern
are subclasses of
Employee
, and
Employee
is a subclass of
Person
.
Hierarchy Description:
Manager
and
Intern
are depicted as subclasses
of
Employee
, indicating a direct relationship.
Employee
is a subclass
of
Person
, showing that all employees are persons by definition in the
hierarchy.
Reasoning:
An individual identified as a
Manager
would inherit properties
and characteristics of both
Employee
and
Person
, due to the subclass
relationships in RDFS.
b) Discuss the implications of assigning a property
worksAt
, with a domain of
Employee
and a range of
Organization
, to an individual of type
Intern
in
terms of property inheritance in RDFS.
Property Inheritance:
The
worksAt
property, when applied to
Employee
,
inherently extends to
Intern
, as
Intern
is a subclass of
Employee
.
Explanation:
This demonstrates how RDFS handles property inheritance,
allowing subclasses to inherit properties from their superclasses, thus
an
Intern
is understood to have the
worksAt
property.
c) Conceptualize an advanced RDF Schema with a complex class hierarchy
involving
Person
,
Author
,
Academic
,
Student
,
GraduateStudent
, and so on,
and discuss the inferencing capabilities offered by such a schema.
Schema Design Description:
The schema would present
GraduateStudent
as a subclass of both
Student
and
Academic
. Further,
Academic
could
be positioned as a subclass of
Author
, which is a subclass of
Person
.
Inferencing Discussion:
Such a schema enables inferencing at multiple
levels, allowing the identification of a
GraduateStudent
as also being a
Student
,
Academic
,
Author
, and
Person
, illustrating the rich inferencing
potential in RDFS.
1
Question 2
We’ll now continue the knowledge graph exercise from the previous week. Your
next task is to develop a new
vocabulary
using RDF Schema (RDFS): The
Friends-of-Concordia-University (FOCU)
schema. This vocabulary should be
able to express:
(i) Classes for
Student
and
Professor
, both of which are subclasses of
foaf:Person
(ii) A
University
class, which is a subclass of
foaf:Organization
(iii) A property describing that a
Student
is enrolled at a
University
(iv) A property describing that a
Professor
teaches
Students
You can start with the following template (in Turtle format):
@prefix rdfs: <http://www.w3.org/2000/01/rdf-schema#> .
@prefix rdf: <http://www.w3.org/1999/02/22-rdf-syntax-ns#> .
@prefix xsd: <http://www.w3.org/2001/XMLSchema#> .
@prefix foaf: <http://xmlns.com/foaf/0.1/> .
@prefix focu: <http://focu.io/schema#> .
@prefix focudata: <http://focu.io/data#> .
# RDF Schema for the FOCU Vocabulary
focu:[CLASSNAME]
a rdfs:Class ;
rdfs:label "[LABEL]"@en .
focu:[OTHERCLASSNAME]
a rdfs:Class ;
rdfs:subClassOf foaf:[CLASS] ;
rdfs:label "[LABEL]"@en .
focu:[PROPERTY]
a rdf:Property ;
rdfs:label "[LABEL]"@en ;
rdfs:comment "[COMMENT]"@en ;
rdfs:domain uni:[DOMAIN
_
CLASS] ;
rdfs:range uni:[RANGE
_
CLASS] .
(a) Add your RDFS triples for the four definitions above by filling in the
missing parts in
[square brackets]
.
(b) Validate your new RDF schema using the tools mentioned in last week’s
lab and visualize them in form of a graph.
(c) Now add some triples using your new FOCU vocabulary that describe
yourself as a student at Concordia. Add information about yourself (age,
email) using the FOAF vocabulary as we did on the lecture Worksheet #8.
2
(d) Again, validate and visualize your triples.
Some notes:
•
There are of course existing vocabularies for universities etc. and in a
real system you would not create a new vocabulary duplicating existing
definitions (just like we re-use FOAF to describe a person here).
This
is just for exercise purposes, so that you see how to define a minimal,
working RDF Schema.
•
We are using
focu.io
as the authority (domain name):
This is again
bad practice, since you do not control this domain name and thus cannot
publish anything using this authority.
•
The template above defines
focudata:
as the namespace for the triples
describing university people; you could use another namespace here, like
the
example.org
we’ve used before. The point here is that it is not good
practice to mix schema information (in this case your FOCU schema)
with data described using this schema into the same namespace. In other
words, you want to be able to load the schema and the data separately
from each other into a system.
•
Can you add a triple that relates your new
University
definition to an
existing concept in the FOAF vocabulary?
Here’s a possible solution:
@prefix rdfs: <http://www.w3.org/2000/01/rdf-schema#> .
@prefix rdf: <http://www.w3.org/1999/02/22-rdf-syntax-ns#> .
@prefix xsd: <http://www.w3.org/2001/XMLSchema#> .
@prefix foaf: <http://xmlns.com/foaf/0.1/> .
@prefix focu: <http://focu.io/schema#> .
@prefix focudata: <http://focu.io/data#> .
# RDF Schema for the FOCU Vocabulary
focu:Student
a rdfs:Class ;
rdfs:subClassOf foaf:Person ;
rdfs:label "Student"@en .
focu:Professor
a rdfs:Class ;
rdfs:subClassOf foaf:Person ;
rdfs:label "Professor"@en .
focu:University
a rdfs:Class ;
rdfs:subClassOf foaf:Organization ;
3
Your preview ends here
Eager to read complete document? Join bartleby learn and gain access to the full version
- Access to all documents
- Unlimited textbook solutions
- 24/7 expert homework help
rdfs:label "University"@en .
focu:teaches
a rdf:Property ;
rdfs:label "teaches"@en ;
rdfs:comment "Relationship showing professors teach the students."@en ;
rdfs:domain focu:Professor ;
rdfs:range focu:Student .
focu:enrolledAt
a rdf:Property ;
rdfs:label "enrolled at"@en ;
rdfs:comment "Relationship showing students enrolled at a university."@en ;
rdfs:domain focu:Student ;
rdfs:range focu:University .
focudata:cu
a focu:University ;
owl:sameAs <http://dbpedia.org/resource/Concordia
_
University> .
focudata:john
a focu:Student ;
foaf:givenName "John" ;
foaf:familyName "Smith" ;
focu:enrolledAt focudata:cu .
focudata:rene
a focu:Professor ;
foaf:givenName "Rene" ;
foaf:familyName "Witte" ;
focu:teaches focudata:john .
Some notes:
(i)
Class Hierarchy
:
•
focu:Student
and
focu:Professor
are defined as subclasses of
foaf:Person
to leverage the well-established FOAF vocabulary.
•
focu:University
as a subclass of
foaf:Organization
provides con-
text within the Semantic Web.
(ii)
Property Definitions
:
•
focu:teaches
establishes a teaching relationship, with
focu:Professor
as domain and
focu:Student
as range.
•
focu:enrolledAt
links students to universities, indicating educational
affiliation.
4
(iii)
Integration with Existing Resources
:
•
Use of
owl:sameAs
: Linking
focu:University
with DBpedia’s re-
source using
owl:sameAs
not only identifies them as equivalent but
also enriches your dataset by incorporating a wealth of related infor-
mation and context available in DBpedia. This connection allows for
more informed and comprehensive data interpretation and querying.
(iv)
Individual Instances
:
•
Instances such as
focudata:john
and
focudata:rene
demonstrate
practical use of FOCU and FOAF vocabularies.
•
We’re using DBpedia instead of Wikidata here, for the sake of another
exercise below.
(v)
Self-Describing Nature of the Dataset
:
•
This example shows how you can make a dataset self-describing. By
relating
focu:University
to an existing class in FOAF, you provide
context and meaning to what might be an “unknown” term for ex-
ternal systems. You can further enhance this by adding relations to
other vocabularies, like DBpedia, aiding automated agents in under-
standing these terms.
(vi)
Encouraging Further Extension
:
•
Try extending the vocabulary by adding more properties or classes.
This will deepen your understanding of how RDF Schema works and
how it can be applied.
(vii)
Importance of Visualization and Validation
:
•
Remember to validate your RDF Schema.
This step is crucial for
ensuring the correctness and usability of your schema.
Also, try
visualizing it like you did in the previous lab.
5
Question 3
Using your RDFLib program from the previous week, read the FOAF+FOCU
triples about some people you created above. Next, your program should ask
for a name and retrieve all the available information from the graph. For exam-
ple, given the name Joe, it will print out all available triples like age, email, etc.:
import
rdflib
from
rdflib
import
Literal, Namespace
from
rdflib.namespace
import
FOAF
Add the user-defined namespaces:
# Create the user-defined namespaces
FOCU = Namespace(
"http://focu.io/schema#"
)
FOCUDATA = Namespace(
"http://focu.io/data#"
)
Here is the command to create a graph instance:
g = rdflib.Graph()
Now parse the graph containing your triples created in Question 1:
g.parse(
"Lab09.ttl"
,
format
=
"turtle"
)
Use the command below to start accepting user input:
user
_
input =
input
(
'Hello, I am your smart university agent. Who are you
looking for? '
)
Hello, I am your smart university agent. Who are you looking for?
> Joe
Joe is 22 years old and has the email address joe@example.com.
Implement searching and printing the information by going through the graph.
Hint:
Use the RDFLib functions you learned in the previous lab to navigate
the graph and retrieve the required information, i.e., age and email address.
Here, the user inputs a person’s first name, therefore find the triples matching
the given first name and then proceed to retrieved the required information
from the graph.
6
Your preview ends here
Eager to read complete document? Join bartleby learn and gain access to the full version
- Access to all documents
- Unlimited textbook solutions
- 24/7 expert homework help
Here is a possible solution:
# Get the subject node using the user input (i.e., the student's first name
→
)
# Note there can be multiple students with this name,
# we only process the first here to keep it simple
student =
list
(g.subjects(FOAF.givenName, Literal(user
_
input)))
if
student:
# Get the age details for the corresponding subject node
age = g.value(subject=student[0], predicate=FOAF.age)
# Get the email address details for the corresponding subject node
mail = g.value(subject=student[0], predicate=FOAF.mbox)
# Optional: Clean up the mail URI
mail =
str
(mail).replace(
'mailto:'
,
''
)
print
(user
_
input +
" is "
+
str
(age) +
" years old and has the email
→
address "
+ mail +
"."
)
else
:
print
(
"No information found for "
+ user
_
input)
7
Question 4
Next, if the person is enrolled at a university, your agent should determine the
location
of the university (using the triples about the university from DBpedia)
and print out this information as well:
Hello, I am your smart university agent. Who are you looking for?
> Jane
Jane is 24 years old and studies at Concordia University,
which is located in Montreal, Quebec, Canada
This time, we’ll use DBpedia to retrieve additional triples, so make sure the stu-
dent in your knowledge base is linked to, e.g.,
http://dbpedia.org/resource/
Concordia
_
University
.
Hint:
The only new information we need here is the location. To obtain it,
you can merge the DBpedia graph about the university into your knowledge
base and fetch all the additional information from there.
Use the following namespace declaration:
DBP = Namespace(
"http://dbpedia.org/property/"
)
and find the correct property to use for the location in DBpedia.
Here’s a possible solution:
# Get the subject node of the student
student =
list
(g.subjects(FOAF.givenName, Literal(user
_
input)))
# Get the university details for the corresponding subject node
uni = g.value(subject=student[0], predicate=FOCU.enrolledAt)
uniref = g.value(subject=uni, predicate=OWL.sameAs)
# Create a new graph, load the triples from DBpedia and retrieve the website
h = rdflib.Graph()
h.parse(uniref)
# Find the location (dbp:location) and retrieve the university's name in English
location = h.value(uniref, predicate = DBP.location)
(uniprop, uniname) = h.preferredLabel(uniref, lang=
'en'
)[0]
print
(user
_
input +
" studies at "
+ uniname +
", which is located in"
, location)
Here’s how it works:
Retrieving the University Node:
After obtaining the student node from
the graph, the script fetches the corresponding university node (
uni
) using
the predicate
FOCU.enrolledAt
.
8
Fetching DBpedia Reference:
Retrieves the DBpedia URI (
uniref
) for the
university, linked to the local university node via
OWL.sameAs
. This linkage
is an example of
Linked Data
principles in action, demonstrating how
disparate data sources can be interconnected.
Loading DBpedia Data:
A new graph (
h
) is created and RDF data from
DBpedia is loaded using
h.parse(uniref)
.
This direct loading method
from a URL is less common in practice; typically, SPARQL queries are
used for data retrieval from sources like DBpedia. However, to simplify
this lab exercise, we’re not covering the use of SPARQL.
Querying for Location:
Queries the DBpedia graph (
h
) for the university’s
location using
DBP.location
.
Outputting Results:
Prints the student’s name, university name, and loca-
tion, combining information from the local graph and DBpedia.
Note:
Additional error handling is recommended to manage cases where data
might be missing or if the DBpedia reference is not found.
You can print out additional information about the university if you like.
Question 5
We’ll now make the AI even smarter, by also supporting the query “What
is
<X>
”, where you retrieve triples about
<X>
from DBpedia, printing out the
abstract
information, e.g.:
Hello, I am your smart university agent. How can I help you?
> What is Concordia University?
Concordia University (French: Université Concordia) is a public
research university located in Montreal, Quebec, Canada. Founded in
1974 following the merger of Loyola College and Sir George Williams
University, Concordia is one of the three universities in Quebec
where English is the primary language of instruction (the others
being McGill and Bishop’s)
. . . .
To implement this, we’ll start by checking whether a “What is
<X>
” pattern is
present in the user’s input:
# Define pattern
pattern =
"What is"
if
pattern
in
user
_
input:
# Extract the entity name after the pattern,
# handling potential trailing question mark
entity
_
name = user
_
input.split(pattern, 1)[1].split(
"?"
, 1)[0].strip()
Implement the following two steps:
9
Your preview ends here
Eager to read complete document? Join bartleby learn and gain access to the full version
- Access to all documents
- Unlimited textbook solutions
- 24/7 expert homework help
(a) Create a DBpedia URI based on the input subject
<X>
and load the graph
into your program. For example, if
<X>
is
Concordia University
, create
the URI
http://dbpedia.org/resource/Concordia
_
University
.
1
(b) Use the RDFLib functions as before to navigate and retrieve the required
output from the graph.
2
You’ll need to find the right property to use;
generally, you can find an
abstract
for most entries in DBpedia.
(a) A naïve approach for converting the questing string into a URI is:
dbpedia
_
URI =
"http://dbpedia.org/resource/"
+ entity
_
name.replace(
" "
,
"
_
"
)
(b) You can then retrieve the graph from DBpedia using:
g.parse(dbpedia
_
URI)
and print out the
dbo:abstract
in the user’s language (here, we use English)
with:
DBO = Namespace(
"http://dbpedia.org/ontology/"
)
comment
_
list =
list
(g.objects(subject=rdflib.term.URIRef(dbpedia
_
URI),
→
predicate=DBO.abstract))
# Print english language comment
for
each
_
lang
in
comment
_
list:
if
each
_
lang == Literal(each
_
lang,lang=
'en'
):
print
(each
_
lang)
Converting Query to URI:
This step converts the user’s query into a DB-
pedia URI by replacing spaces with underscores and appending it to the
DBpedia resource base URI. Remember, this approach is simplified for
this lab.
Printing
dbo:abstract
:
You iterate over the abstracts associated with the
DBpedia resource to find and print the English abstract.
This method
highlights extracting specific language-based information from RDF data.
Contextual Understanding:
This exercise simulates responses similar to
those from AI chatbots, illustrating the concept of “grounding” in knowl-
edge graphs, as opposed to simple pattern-matching.
Handling Different Query Patterns:
You can extend this method for var-
ious query types, like "Who is
<X>
?", enhancing your ability to handle
diverse RDF data queries.
1
Of course, this naïve approach of converting a string to a DBpedia URI does not always work in practice,
but there are more sophisticated ways available of achieving this, for example, the DBpedia Spotlight tool:
https://www.dbpedia-spotlight.org/
.
2
Another way of implementing this would be to generate SPARQL queries, similar to how you’ve done it
on Worksheet #8. However, we’re not covering SPARQL in this lab.
10
You can then handle the case of the question pattern “Who is
<X>
” with code
similar to Question
3
above.
Notice how similar this exercise is to the functionality of AI assistants like
Google Assistant, Siri, or Alexa: By querying DBpedia for
‘What is <X>?’
,
you’re emulating their technique of using knowledge graphs to provide infor-
mative answers.
This process, known as
grounding,
is a departure from the
pattern-matching approach seen in earlier chatbots like Eliza, demonstrating
a more advanced and context-aware method of responding to user queries.
In addition to powering AI assistants, knowledge graphs have significant appli-
cations across various industries. For instance, they are instrumental in health-
care for organizing complex medical data, aiding in diagnosis and patient care.
In the financial sector, knowledge graphs facilitate fraud detection and risk
management by analyzing intricate transaction networks. While distinct from
Large Language Models (LLMs) like GPT-3, which generate responses based
on pattern recognition in extensive text data, knowledge graphs offer a struc-
tured, queryable approach to handling information. This structured approach
is particularly valuable in scenarios requiring precise, verifiable data retrieval
or where relationships between different entities are crucial.
Understanding
knowledge graphs thus provides foundational insights into a key aspect of AI,
relevant for fields ranging from semantic search and recommendation systems
to complex data analysis in research and industry.
11
Recommended textbooks for you
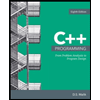
C++ Programming: From Problem Analysis to Program...
Computer Science
ISBN:9781337102087
Author:D. S. Malik
Publisher:Cengage Learning
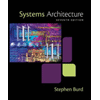
Systems Architecture
Computer Science
ISBN:9781305080195
Author:Stephen D. Burd
Publisher:Cengage Learning
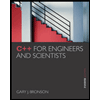
C++ for Engineers and Scientists
Computer Science
ISBN:9781133187844
Author:Bronson, Gary J.
Publisher:Course Technology Ptr
Programming Logic & Design Comprehensive
Computer Science
ISBN:9781337669405
Author:FARRELL
Publisher:Cengage
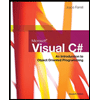
Microsoft Visual C#
Computer Science
ISBN:9781337102100
Author:Joyce, Farrell.
Publisher:Cengage Learning,
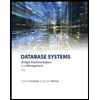
Database Systems: Design, Implementation, & Manag...
Computer Science
ISBN:9781305627482
Author:Carlos Coronel, Steven Morris
Publisher:Cengage Learning
Recommended textbooks for you
- C++ Programming: From Problem Analysis to Program...Computer ScienceISBN:9781337102087Author:D. S. MalikPublisher:Cengage LearningSystems ArchitectureComputer ScienceISBN:9781305080195Author:Stephen D. BurdPublisher:Cengage LearningC++ for Engineers and ScientistsComputer ScienceISBN:9781133187844Author:Bronson, Gary J.Publisher:Course Technology Ptr
- Programming Logic & Design ComprehensiveComputer ScienceISBN:9781337669405Author:FARRELLPublisher:CengageMicrosoft Visual C#Computer ScienceISBN:9781337102100Author:Joyce, Farrell.Publisher:Cengage Learning,Database Systems: Design, Implementation, & Manag...Computer ScienceISBN:9781305627482Author:Carlos Coronel, Steven MorrisPublisher:Cengage Learning
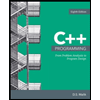
C++ Programming: From Problem Analysis to Program...
Computer Science
ISBN:9781337102087
Author:D. S. Malik
Publisher:Cengage Learning
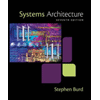
Systems Architecture
Computer Science
ISBN:9781305080195
Author:Stephen D. Burd
Publisher:Cengage Learning
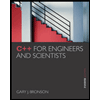
C++ for Engineers and Scientists
Computer Science
ISBN:9781133187844
Author:Bronson, Gary J.
Publisher:Course Technology Ptr
Programming Logic & Design Comprehensive
Computer Science
ISBN:9781337669405
Author:FARRELL
Publisher:Cengage
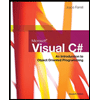
Microsoft Visual C#
Computer Science
ISBN:9781337102100
Author:Joyce, Farrell.
Publisher:Cengage Learning,
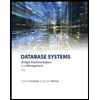
Database Systems: Design, Implementation, & Manag...
Computer Science
ISBN:9781305627482
Author:Carlos Coronel, Steven Morris
Publisher:Cengage Learning