ISYE 7406 HW4
pdf
School
Georgia Institute Of Technology *
*We aren’t endorsed by this school
Course
7406
Subject
Computer Science
Date
Dec 6, 2023
Type
Pages
10
Uploaded by EarlResolve98061
ISyE 7406: Data Mining & Statistical Learning
HW#4
INTRODUCTION The goal of this homework is to help better understand the statistical properties and computational challenges of local smoothing such as loess, Nadaraya-Watson (NW) kernel smoothing, and spline smoothing. For this purpose, we will compute empirical bias, empirical variances, and empirical mean square error (MSE) based on m = 1000 Monte Carlo runs, where in each run we simulate a data set of n = 101 observations from the additive noise model Yi = f (xi) + €
i with the famous Mexican hat function f (x) = (1 –
x2) exp(
−
0.5x2), −
2
π
≤
x ≤
2
π
, and €
1, · · · , €
n are independent and identically distributed (iid) N (0, 0.22). This function is known to pose a variety of estimation challenges, and below we explore the difficulties inherent in this function EXPLOTATORY DATA ANALYSIS The x-values are systematically generated as equidistant points between -
2π and 2π, comprising a fixed design of 101 points uniformly spaced at an interval of 0.1256637 units between each point. In the non-equidistant design, x-values are generated between -
2π and 2π, but the distances between them vary in such a way that x[1] - x[2] is not equal to x[2] - x[3], and this pattern continues up to x[101]. Figure 1: Plot of the equidistant design Figure 2: Plot of the non- equidistant design
Two datasets were generated through a Monte Carlo simulation, comprising 1000 runs for each smoothing model. The first dataset consisted of 101 equidistant points, while the second dataset included 101 non-equidistant points randomly generated in R. In each run, the three local smoothing methods (LOESS, NW kernel smoothing, and Spline Smoothing) were applied to the datasets, and the resulting fitted values were recorded. The analysis involved computing and visualizing the empirical bias, variance, and mean squared error (MSE). These investigations aimed to assess the performance and statistical properties of the three smoothing methods in the context of these simulated datasets, which posed a known estimation challenge due to the presence of the Mexican Hat function. METHODOLOGY A Monte Carlo simulation involving 1000 runs for each smoothing model was employed to generate three datasets. The initial model chosen was LOESS, a method that utilizes local smoothing to fit a polynomial surface based on one or more predictors. While cross-validation is typically performed to determine the optimal span, a span of 0.75 was pre-specified for this simulation. In the context of leave-
one-out cross-validation with k-folds, improvements could potentially be made by selecting the model with the lowest root mean square error of prediction (RMSEP). Here's a brief overview of the local smoothing models used: 1. LOESS (Locally Weighted Scatterplot Smoothing): LOESS is a non-parametric regression technique that combines linear regression and local weighted smoothing to fit a smooth curve to a scatterplot. It estimates the value of each data point by fitting a weighted regression model to a local subset of the data, with the weights determined by a kernel function. The level of smoothing is controlled by a smoothing parameter, which governs the size of the local subset. 2. Nadaraya-Watson (NW) Kernel Smoothing: NW kernel smoothing is another non-parametric regression technique that estimates the value of each data point as a weighted average of its neighbors, with the weights determined by a kernel function. The degree of smoothing is controlled by a bandwidth parameter, which determines the size of the neighborhood. NW kernel smoothing is computationally efficient and suitable for high-dimensional data. 3. Spline Smoothing: Spline smoothing is a parametric regression technique that fits a piecewise polynomial function to the data. The degree of the polynomial and the location of knots are determined by a smoothing parameter. Spline smoothing can handle data with complex nonlinear relationships but requires more computation compared to the other two methods. RESULTS AND FINDINGS 1.
Equidistant design The comparison of empirical bias values reveals a significant challenge at x = 0 when compared to other x-values, primarily due to the broader range of response values at this point. In accordance with the bias-
variance trade-off principle, smaller empirical bias values typically coincide with larger empirical variances, and vice versa. Notably, the LOESS estimator outperforms the other two local smoothing methods concerning empirical bias and MSE values, likely due to the choice of a relatively higher span parameter (0.75). This could lead to a degree of over-smoothing.
Conversely, Spline smoothing exhibits superior performance in terms of empirical MSE values, but this advantage may be attributed to its default tuning using generalized cross-validation. In practical scenarios, cross-validation is typically employed to fine-tune model parameters. It's essential to note that the comparison may not be entirely precise since specific tuning values were employed for the other two local smoothing methods, potentially resulting in suboptimal performance due to insufficient parameter tuning. Plots of the equidistant design's fitted mean, empirical bias, empirical variance, and empirical MSE are shown below. Figure 3: Fitted mean Figure 4: Bias
Your preview ends here
Eager to read complete document? Join bartleby learn and gain access to the full version
- Access to all documents
- Unlimited textbook solutions
- 24/7 expert homework help
Figure 5: Variance Figure 6: MSE The fitted values for the LOESS estimator with a span of 0.75, NW kernel smoothing using a Gaussian Kernel with a bandwidth of 0.2, and spline smoothing are represented by the black, red, and blue plotted lines, respectively. 2.
Non equidistant design In the presented plots, we explore the empirical bias and mean squared error (MSE) of three distinct smoothing methods: spline smoothing, kernel smoothing, and LOESS, when applied to a dataset featuring the Mexican hat function. This dataset encompasses both equidistant and non-equidistant x values. A notable observation is that x = 0 stands out, exhibiting significantly larger empirical bias and MSE compared to other x values. This highlights the inherent challenge faced by these methods in accurately estimating the function in this specific region. Interestingly, an inverse relationship between empirical bias and empirical variance is evident across all three estimators. When analyzing the non-equidistant dataset, we notice a slightly higher empirical bias in the spline smoothing method in comparison to the equidistant dataset. This may be attributed to potential over-smoothing caused by a relatively larger spar parameter. Conversely, the LOESS model in the non-equidistant setup displays generally smaller empirical bias and MSE than its equidistant counterpart. This improvement is likely linked to the use of a smaller LOESS span, which enhances the local fit and reduces both bias and MSE.
It's crucial to highlight that the results observed for the non-equidistant dataset are contingent on specific tuning parameters. In practical applications, cross-validation will be indispensable to identify the optimal tuning parameters for the three local smoothing methods. Nevertheless, it's worth noting that implementing cross-validation can be computationally intensive, especially for memory-intensive methods such as kernel and local regression. Plots of the non- equidistant design's empirical bias, empirical variance, and empirical MSE are shown below: Figure 7: Bias Figure 8: Variance
Figure 9: MSE The fitted values for the LOESS (Span = 0.3365), NW kernel smoothing (Gaussian Kernel, Bandwidth = 0.2), and spline smoothing (Spar=0.7163) estimators are shown by the black, red, and blue plotted lines, respectively In summary local smoothing methods encounter challenges in accurately estimating the Mexican hat function, particularly in the vicinity of x = 0. This challenge is evident from a statistical perspective. Empirical plots further illustrate a key principle known as the bias-variance trade-off, wherein smaller bias tends to coincide with larger variance, and vice versa. When utilizing local smoothing methods, the selection of appropriate hyperparameters is of paramount importance to achieve an optimal model fit. It's worth noting that the process of hyperparameter selection, particularly for local smoothing models, can be computationally demanding. Cross-validation techniques, such as k-fold cross-validation, are often employed to identify the most suitable hyperparameters, but they come with a computational cost. APPENDIX R CODE x2 <- read.table(file = "HW04part2-1.x.csv", header = TRUE) # Part #1 deterministic equidistant design m <- 1000 n <- 101
Your preview ends here
Eager to read complete document? Join bartleby learn and gain access to the full version
- Access to all documents
- Unlimited textbook solutions
- 24/7 expert homework help
x <- seq(-2 * pi, 2 * pi, length.out = n) yi <- (1 - x^2) * exp(-0.5 * x^2) df <- data.frame(x = x, y = yi) # Initialize matrices for fitted values fvlp <- fvnw <- fvss <- matrix(0, nrow = n, ncol = m) for (j in 1:m) { y <- yi + rnorm(length(x), sd = 0.2) fvlp[, j] <- predict(loess(y ~ x, span = 0.75), newdata = data.frame(x = x)) fvnw[, j] <- ksmooth(x, y, kernel = "normal", bandwidth = 0.2, x.points = x)$y fvss[, j] <- predict(smooth.spline(y ~ x), x = x)$y } # Calculate the means meanlp <- apply(fvlp, 1, mean) meannw <- apply(fvnw, 1, mean) meanss <- apply(fvss, 1, mean) dmin <- min(meanlp, meannw, meanss) dmax <- max(meanlp, meannw, meanss) # Plot means matplot(x, meanlp, "l", ylim = c(dmin, dmax), ylab = "Response") matlines(x, meannw, col = "red") matlines(x, meanss, col = "blue") # Define a function to calculate bias calculate_bias <- function(fv, yi) { apply(fv, 1, mean) - yi
} # Plot bias for each method lo_bias <- calculate_bias(fvlp, yi) nw_bias <- calculate_bias(fvnw, yi) ss_bias <- calculate_bias(fvss, yi) bias_min <- min(lo_bias, nw_bias, ss_bias) bias_max <- max(lo_bias, nw_bias, ss_bias) matplot(x, lo_bias, "l", ylim = c(bias_min, bias_max), ylab = "Empirical Bias") matlines(x, nw_bias, col = "red") matlines(x, ss_bias, col = "blue") # Define a function to calculate variance (MSE) calculate_variance <- function(fv, yi, m) { apply((fv - yi)^2, 1, sum) / (m - 1) } # Plot variance for each method lo_var <- calculate_variance(fvlp, yi, m) nw_var <- calculate_variance(fvnw, yi, m) ss_var <- calculate_variance(fvss, yi, m) var_min <- min(lo_var, nw_var, ss_var) var_max <- max(lo_var, nw_var, ss_var) matplot(x, lo_var, "l", ylim = c(var_min, var_max), ylab = "Empirical Variance (MSE)") matlines(x, nw_var, col = "red") matlines(x, ss_var, col = "blue") # Part #2 non-equidistant design x2 <- read.table(file = "HW04part2-1.x.csv", header = TRUE)$x
fvlp2 <- fvnw2 <- fvss2 <- list() for (j in 1:m) { y2 <- (1 - x2^2) * exp(-0.5 * x2^2) + rnorm(length(x2), sd = 0.2) fvlp2[[j]] <- predict(loess(y2 ~ x2, span = 0.3365), newdata = data.frame(x2 = x2)) fvnw2[[j]] <- ksmooth(x2, y2, kernel = "normal", bandwidth = 0.2, x.points = x2)$y fvss2[[j]] <- predict(smooth.spline(y2 ~ x2, spar = 0.7163), x = x2)$y } # Convert list of results into matrices fvlp2 <- do.call(cbind, fvlp2) fvnw2 <- do.call(cbind, fvnw2) fvss2 <- do.call(cbind, fvss2) # Calculate the means meanlp2 <- apply(fvlp2, 1, mean) meannw2 <- apply(fvnw2, 1, mean) meanss2 <- apply(fvss2, 1, mean) dmin2 <- min(meanlp2, meannw2, meanss2) dmax2 <- max(meanlp2, meannw2, meanss2) # Plot the fitted values for each method matplot(x2, meanlp2, "l", ylim = c(dmin2, dmax2), ylab = "Response (Non-Equidistant Design)") matlines(x2, meannw2, col = "red") matlines(x2, meanss2, col = "blue") # Plot bias for each method lo_bias2 <- calculate_bias(fvlp2, y2i) nw_bias2 <- calculate_bias(fvnw2, y2i)
Your preview ends here
Eager to read complete document? Join bartleby learn and gain access to the full version
- Access to all documents
- Unlimited textbook solutions
- 24/7 expert homework help
ss_bias2 <- calculate_bias(fvss2, y2i) bias_min2 <- min(lo_bias2, nw_bias2, ss_bias2) bias_max2 <- max(lo_bias2, nw_bias2, ss_bias2) plot(x2, lo_bias2, type = "l", ylim = c(bias_min2, bias_max2), ylab = "Empirical Bias (Non-Equidistant Design)") lines(x2, nw_bias2, col = "red") # Plot variance for each method lo_var2 <- calculate_variance(fvlp2, y2i, m) nw_var2 <- calculate_variance(fvnw2, y2i, m) ss_var2 <- calculate_variance(fvss2, y2i, m) var_min2 <- min(lo_var2, nw_var2, ss_var2) var_max2 <- max(lo_var2, nw_var2, ss_var2) plot(x2, lo_var2, type = "l", ylim = c(var_min2, var_max2), ylab = "Empirical Variance (Non-Equidistant Design)") lines(x2, nw_var2, col = "red") lines(x2, ss_var2, col = "blue") # Plot MSE for each method mse_min2 <- min(calculate_variance(fvlp2, y2i, m), calculate_variance(fvnw2, y2i, m), calculate_variance(fvss2, y2i, m)) mse_max2 <- max(calculate_variance(fvlp2, y2i, m), calculate_variance(fvnw2, y2i, m), calculate_variance(fvss2, y2i, m)) plot(x2, lo_var2, type = "l", ylim = c(mse_min2, mse_max2), ylab = "Empirical MSE (Non-Equidistant Design)") lines(x2, nw_var2, col = "red") lines(x2, ss_var2, col = "blue") REFERENCES ISYE 7406 lecture notes and codes
Related Documents
Recommended textbooks for you
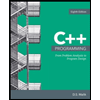
C++ Programming: From Problem Analysis to Program...
Computer Science
ISBN:9781337102087
Author:D. S. Malik
Publisher:Cengage Learning
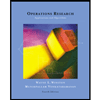
Operations Research : Applications and Algorithms
Computer Science
ISBN:9780534380588
Author:Wayne L. Winston
Publisher:Brooks Cole
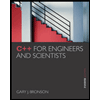
C++ for Engineers and Scientists
Computer Science
ISBN:9781133187844
Author:Bronson, Gary J.
Publisher:Course Technology Ptr
COMPREHENSIVE MICROSOFT OFFICE 365 EXCE
Computer Science
ISBN:9780357392676
Author:FREUND, Steven
Publisher:CENGAGE L
Recommended textbooks for you
- C++ Programming: From Problem Analysis to Program...Computer ScienceISBN:9781337102087Author:D. S. MalikPublisher:Cengage LearningOperations Research : Applications and AlgorithmsComputer ScienceISBN:9780534380588Author:Wayne L. WinstonPublisher:Brooks ColeC++ for Engineers and ScientistsComputer ScienceISBN:9781133187844Author:Bronson, Gary J.Publisher:Course Technology Ptr
- COMPREHENSIVE MICROSOFT OFFICE 365 EXCEComputer ScienceISBN:9780357392676Author:FREUND, StevenPublisher:CENGAGE L
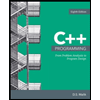
C++ Programming: From Problem Analysis to Program...
Computer Science
ISBN:9781337102087
Author:D. S. Malik
Publisher:Cengage Learning
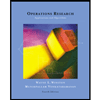
Operations Research : Applications and Algorithms
Computer Science
ISBN:9780534380588
Author:Wayne L. Winston
Publisher:Brooks Cole
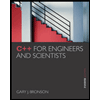
C++ for Engineers and Scientists
Computer Science
ISBN:9781133187844
Author:Bronson, Gary J.
Publisher:Course Technology Ptr
COMPREHENSIVE MICROSOFT OFFICE 365 EXCE
Computer Science
ISBN:9780357392676
Author:FREUND, Steven
Publisher:CENGAGE L