Here are two diagrams. Make them very explicit, similar to Example Diagram 3 (the Architecture of MSCTNN).
graph LR subgraph Teacher_Model_B [Teacher Model (Pretrained)] Input_Teacher_B[Input C (Complete Data)] --> Teacher_Encoder_B[Transformer Encoder T] Teacher_Encoder_B --> Teacher_Prediction_B[Teacher Prediction y_T] Teacher_Encoder_B --> Teacher_Features_B[Internal Features F_T] end subgraph Student_B_Model [Student Model B (Handles Missing Labels)] Input_Student_B[Input C (Complete Data)] --> Student_B_Encoder[Transformer Encoder E_B] Student_B_Encoder --> Student_B_Prediction[Student B Prediction y_B] end subgraph Knowledge_Distillation_B [Knowledge Distillation (Student B)] Teacher_Prediction_B -- Logits Distillation Loss (L_logits_B) --> Total_Loss_B Teacher_Features_B -- Feature Alignment Loss (L_feature_B) --> Total_Loss_B Partial_Labels_B[Partial Labels y_p] -- Prediction Loss (L_pred_B) --> Total_Loss_B Total_Loss_B -- Backpropagation --> Student_B_Encoder end Teacher_Prediction_B -- Logits --> Logits_Distillation_B Teacher_Features_B -- Features --> Feature_Alignment_B Feature_Alignment_B -- Feature Alignment Loss (L_feature_B) --> Knowledge_Distillation_B Logits_Distillation_B -- Logits Distillation Loss (L_logits_B) --> Knowledge_Distillation_B Partial_Labels_B -- Available Labels --> Prediction_Loss_B Prediction_Loss_B -- Prediction Loss (L_pred_B) --> Knowledge_Distillation_B style Knowledge_Distillation_B fill:#aed,stroke:#333,stroke-width:2px style Total_Loss_B fill:#fff,stroke:#333,stroke-width:2px
graph LR subgraph Teacher Model (Pretrained) Input_Teacher[Input C (Complete Data)] --> Teacher_Encoder[Transformer Encoder T] Teacher_Encoder --> Teacher_Prediction[Teacher Prediction y_T] Teacher_Encoder --> Teacher_Features[Internal Features F_T] end subgraph Student_A_Model[Student Model A (Handles Missing Values)] Input_Student_A[Input M (Data with Missing Values)] --> Student_A_Encoder[Transformer Encoder E_A] Student_A_Encoder --> Student_A_Prediction[Student A Prediction y_A] Student_A_Encoder --> Student_A_Features[Student A Features F_A] end subgraph Knowledge_Distillation_A [Knowledge Distillation (Student A)] Teacher_Prediction -- Logits Distillation Loss (L_logits_A) --> Total_Loss_A Teacher_Features -- Feature Alignment Loss (L_feature_A) --> Total_Loss_A Ground_Truth_A[Ground Truth y_gt] -- Prediction Loss (L_pred_A) --> Total_Loss_A Total_Loss_A -- Backpropagation --> Student_A_Encoder end Teacher_Prediction -- Logits --> Logits_Distillation_A Teacher_Features -- Features --> Feature_Alignment_A Feature_Alignment_A -- Feature Alignment Loss (L_feature_A) --> Knowledge_Distillation_A Logits_Distillation_A -- Logits Distillation Loss (L_logits_A) --> Knowledge_Distillation_A Ground_Truth_A -- Labels --> Prediction_Loss_A Prediction_Loss_A -- Prediction Loss (L_pred_A) --> Knowledge_Distillation_A style Knowledge_Distillation_A fill:#ccf,stroke:#333,stroke-width:2px style Total_Loss_A fill:#fff,stroke:#333,stroke-width:2px
i have also attached the diagram code for both for you reference the two diagram must be very explicit
please there were an answwer which did not satisfy my need
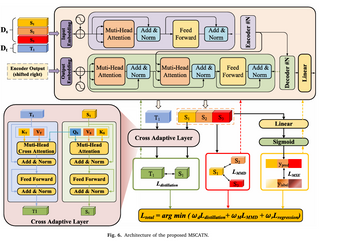

Step by stepSolved in 2 steps

- A(n) __________ is a storage location implemented in the CPU.arrow_forwardIn a CPU, _______ arithmetic generally is easier to implement than _______ arithmetic because of a simpler data coding scheme and data manipulation circuitry.arrow_forwardA problem-solving procedure that requires executing one or more comparison and branch instructions is called a(n) __________.arrow_forward
- (Practice) Show how the name KINGSLEY is stored in a computer that uses the ASCII code by drawing a diagram similar to Figure 2.7, shown previously.arrow_forward(Practice) You’re given the task of wiring and installing lights in your attic. Determine a set of subtasks to accomplish this task. (Hint: The first subtask is determining the placement of light fixtures.)arrow_forward(Practice) State whether the following are valid function names and if so, whether they’re mnemonic names that convey some idea of the function’s purpose. If they are invalid names, state why. powerdensity m1234 newamp 1234 abcd total tangent absval computed b34a 34ab volts$ a2B3 while minVal sine $sine cosine speed netdistance sum return stackarrow_forward
- Programming Logic & Design ComprehensiveComputer ScienceISBN:9781337669405Author:FARRELLPublisher:CengageSystems ArchitectureComputer ScienceISBN:9781305080195Author:Stephen D. BurdPublisher:Cengage LearningNp Ms Office 365/Excel 2016 I NtermedComputer ScienceISBN:9781337508841Author:CareyPublisher:Cengage
- EBK JAVA PROGRAMMINGComputer ScienceISBN:9781337671385Author:FARRELLPublisher:CENGAGE LEARNING - CONSIGNMENTCOMPREHENSIVE MICROSOFT OFFICE 365 EXCEComputer ScienceISBN:9780357392676Author:FREUND, StevenPublisher:CENGAGE LC++ for Engineers and ScientistsComputer ScienceISBN:9781133187844Author:Bronson, Gary J.Publisher:Course Technology Ptr
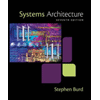
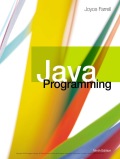
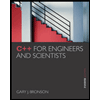