Do not understand this paragraph, please explain what it is saying. Depending on the construction of the choice sequence, the quality inference can be vastly different. This paper meets these challenges by studying observational learning in perhaps its cleanest environment—the U.S. market of transplant kidneys. When a deceased-donor kidney is procured, compatible transplant candidates are sorted into a queue following a nationally implemented priority system. The kidney travels down the queue until a patient is willing to accept it for transplantation. It is ideal to study observational learning in this kidney market for the following reasons. First, decisions are sequential, and the sequence is constructed through a commonly known process. Second, privacy concerns and the limited decision time minimize the chance for between-patient communication. Meanwhile, observational learning is fully enabled in that all previous decisions are observable—the fact that a patient is offered a kidney unambiguously implies that all preceding patients on the queue have turned down this kidney. Third, the kidney market is unlikely to be influenced by other primary mechanisms behind uniform social behavior, such as sanctions of deviants, preference for social identification (e.g., Kuksov 2007), and network effects (e.g., Yang and Allenby 2003, Nair, Chintagunta and Dub´e 2004, Sun, Xie and Cao 2004). In particular, kidneys do not contain the “public appearance value” that partly explains the urge for possessing the right cell phone, choosing the right restaurant, or sporting the right fashion gear. This paper adopts a structural Bayesian approach to modeling observational learning. While all patients on a queue observe the objective kidney quality measures (e.g., donor age), each patient also receives a private quality signal (e.g., her physician’s recommendation). If a kidney is passed on to the second patient, she knows that the first patient’s private signal must have failed to reach a threshold determined by the first patient’s utility function. The second patient can then apply Bayes’ rule to update her quality perception of this kidney. Ceteris paribus, the first patient’s rejection decision lowers the second patient’s perception of the kidney’s quality and hence her propensity to accept. The second patient’s likely refusal in turn lowers the quality perception for subsequent patients, triggering a herd of refusals down the queue. As a result, a kidney’s chance of 3 acceptance critically depends on its choice history as well as its intrinsic quality. There are several advantages to the structural modeling approach.
Do not understand this paragraph, please explain what it is saying.
Depending on the construction of the choice sequence, the quality inference can be vastly different. This paper meets these challenges by studying observational learning in perhaps its cleanest environment—the U.S. market of transplant kidneys. When a deceased-donor kidney is procured, compatible transplant candidates are sorted into a queue following a nationally implemented priority system. The kidney travels down the queue until a patient is willing to accept it for transplantation. It is ideal to study observational learning in this kidney market for the following reasons. First, decisions are sequential, and the sequence is constructed through a commonly known process. Second, privacy concerns and the limited decision time minimize the chance for between-patient communication. Meanwhile, observational learning is fully enabled in that all previous decisions are observable—the fact that a patient is offered a kidney unambiguously implies that all preceding patients on the queue have turned down this kidney. Third, the kidney market is unlikely to be influenced by other primary mechanisms behind uniform social behavior, such as sanctions of deviants, preference for social identification (e.g., Kuksov 2007), and network effects (e.g., Yang and Allenby 2003, Nair, Chintagunta and Dub´e 2004, Sun, Xie and Cao 2004). In particular, kidneys do not contain the “public appearance value” that partly explains the urge for possessing the right cell phone, choosing the right restaurant, or sporting the right fashion gear. This paper adopts a structural Bayesian approach to modeling observational learning. While all patients on a queue observe the objective kidney quality measures (e.g., donor age), each patient also receives a private quality signal (e.g., her physician’s recommendation). If a kidney is passed on to the second patient, she knows that the first patient’s private signal must have failed to reach a threshold determined by the first patient’s utility function. The second patient can then apply Bayes’ rule to update her quality perception of this kidney. Ceteris paribus, the first patient’s rejection decision lowers the second patient’s perception of the kidney’s quality and hence her propensity to accept. The second patient’s likely refusal in turn lowers the quality perception for subsequent patients, triggering a herd of refusals down the queue. As a result, a kidney’s chance of 3 acceptance critically depends on its choice history as well as its intrinsic quality. There are several advantages to the structural modeling approach.

Step by step
Solved in 3 steps

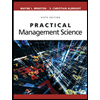
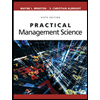