(a) Find the probability mass function of (X₁ + X₂). (b) Using the result of (a), guess what the probability mass function of X, will be and i=1 substantiate your guess by using the method of induction. In (c), (d), (e), and (f), consider the problem of testing at a = 0.038406. Ho p= 0.5 versus Ha p = 0.2 : n (c) Write down the likelihood function L(p; x), where x = (x1,x2,. .., n) is the data sample realized from the random sample X = (X1, X2,..., Xn). points. (d) Write down the expression for the Neyman-Pearson test statistic T(x) and the corresponding rejection region T as outlined in the posted notes on Neyman-Pearson Lemma. (e) When n = 5, obtain a description of the rejection region T that does not depend on the value 0.2 of the parameter p specified under Ha. (f) When n = 5, find the value of 3 up to six places of decimals.
(a) Find the probability mass function of (X₁ + X₂). (b) Using the result of (a), guess what the probability mass function of X, will be and i=1 substantiate your guess by using the method of induction. In (c), (d), (e), and (f), consider the problem of testing at a = 0.038406. Ho p= 0.5 versus Ha p = 0.2 : n (c) Write down the likelihood function L(p; x), where x = (x1,x2,. .., n) is the data sample realized from the random sample X = (X1, X2,..., Xn). points. (d) Write down the expression for the Neyman-Pearson test statistic T(x) and the corresponding rejection region T as outlined in the posted notes on Neyman-Pearson Lemma. (e) When n = 5, obtain a description of the rejection region T that does not depend on the value 0.2 of the parameter p specified under Ha. (f) When n = 5, find the value of 3 up to six places of decimals.
Calculus For The Life Sciences
2nd Edition
ISBN:9780321964038
Author:GREENWELL, Raymond N., RITCHEY, Nathan P., Lial, Margaret L.
Publisher:GREENWELL, Raymond N., RITCHEY, Nathan P., Lial, Margaret L.
Chapter13: Probability And Calculus
Section13.CR: Chapter 13 Review
Problem 6CR
Related questions
Question

Transcribed Image Text:(a) Find the probability mass function of (X₁ + X₂).
n
(b) Using the result of (a), guess what the probability mass function of EX, will be and
i=1
substantiate
your guess by using the method of induction.
In (c), (d), (e), and (f), consider the problem of testing
at a 0.038406.
Ho p= 0.5 versus Ha: p = 0.2
(c) Write down the likelihood function L(p; x), where x =
(x1, x2,
sample realized from the random sample X = (X₁, X2,..., Xn).
points.
(d) Write down the expression for the Neyman-Pearson test statistic T(x) and the
corresponding rejection region T as outlined in the posted notes on Neyman-Pearson
Lemma.
(g) When n
(e) When n = 5, obtain a description of the rejection region T that does not depend on
the value 0.2 of the parameter p specified under Ha.
(f) When n = 5, find the value of 3 up to six places of decimals.
,xn) is the data
=
= 5, use the decision rule (T, T) you formulated in (e) to test
Ho: p= 0.5 versus Ho: P < 0.5
at a = 0.038406. Calculate your Type II Error probability at p = 0.16 up to six places of
decimals.
(h) If I use an arbitrary decision rule (S, S) to test
Ho p = 0.5 versus Ho: p < 0.5
:
at a ≤ 0.038406 with n = 5, how will your Type II Error probability at p = 0.16
calculated in (g) compare with that of mine? Explain.

Transcribed Image Text:This problem explores the question of testing
Ho p= 0.5 versus Ho p < 0.5,
.
based on a random sample X₁, X2,
Xn from the Geometric (p) distribution.
Recall that there is an ambiguity surrounding the definition of the Geometric (p)
distribution, so let us unequivocally specify which distribution we are going to work
with. In the experiment of repeatedly tossing a coin with the probability of getting a Head
in a single toss of the coin equal to p = (0, 1) till a Head is obtained, let X denote the
number of Tails obtained before the experiment is terminated. Then X has the
Geometric (p) distribution.
Further recall that X₁, X2,, Xn is a random sample of size n from the Geometric (p)
distribution means X (X₁, X2, Xn) is a collection of mutually independent
random variables, with each X; having the Geometric (p) distribution. Before proceeding
further, let us recall the following definition.
Definition A collection of random variables {Y₁, Y2,..., Yr} defined on the same sample
space, with S, denoting the support of Y; for 1≤ i ≤r, is said to be mutually
independent if, V (S1, S2,
, Sr) EX S, the Cartesian product of (S₁, S2, ..., Sr),
1 = 1
are independent.
r
P(‚ñ‚ (Y₁ = $i)) = [[P(Y₁ = 81).
1=1
Remember that if {Y₁, Y2,, Yr} is a collection of mutually independent random
variables, then for any 1 ≤ m ≤r, the random variables
U = g(Y₁, Y2₂, •, Ym) and V = h(Ym+1, Ym+2,, Yr)
Expert Solution

This question has been solved!
Explore an expertly crafted, step-by-step solution for a thorough understanding of key concepts.
Step 1: Determine the variables
VIEWStep 2: Estimate the probability mass function
VIEWStep 3: Estimate the probability function
VIEWStep 4: Estimate the likelihood function
VIEWStep 5: Estimate the test statistic
VIEWStep 6: Descibe the rejection region
VIEWStep 7: Estimate the type II probability
VIEWSolution
VIEWStep by step
Solved in 8 steps with 44 images

Recommended textbooks for you
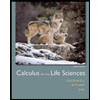
Calculus For The Life Sciences
Calculus
ISBN:
9780321964038
Author:
GREENWELL, Raymond N., RITCHEY, Nathan P., Lial, Margaret L.
Publisher:
Pearson Addison Wesley,
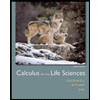
Calculus For The Life Sciences
Calculus
ISBN:
9780321964038
Author:
GREENWELL, Raymond N., RITCHEY, Nathan P., Lial, Margaret L.
Publisher:
Pearson Addison Wesley,