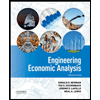
ENGR.ECONOMIC ANALYSIS
14th Edition
ISBN: 9780190931919
Author: NEWNAN
Publisher: Oxford University Press
expand_more
expand_more
format_list_bulleted
Question
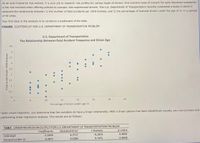
Transcribed Image Text:As an auto insurance risk analyst, it is your job to research risk profiles for various types of drivers. One common area of concern for auto insurance companies is the risk involved when offering policies to younger, less experienced drivers. The U.S. Department of Transportation recently conducted a study in which it analyzed the relationship between 1) the number of fatal accidents per 1000 licenses, and 2) the percentage of licensed drivers under the age of 21 in a sample of 42 cities.
Your first step in the analysis is to construct a scatterplot of the data.
**Figure**: Scatterplot for U.S. Department of Transportation Problem
*Scatterplot Description*
The scatterplot titled "U.S. Department of Transportation: The Relationship Between Fatal Accident Frequency and Driver Age" shows data points representing various cities. The x-axis represents the percentage of drivers under age 21, ranging from 6 to 20. The y-axis represents fatal accidents per 1000 licenses, ranging from 0 to 4.5. The plot indicates a positive trend, where an increase in the percentage of drivers under 21 corresponds to an increase in fatal accidents per 1000 licenses.
Upon visual inspection, you determine that the variables do have a linear relationship. After a linear pattern has been established visually, you now proceed with performing linear regression analysis. The results are as follows:
**Table**: Linear Regression Output for U.S. Department of Transportation Problem
| | Coefficients | Standard Error | t Statistic | p-value |
|----------------------|--------------|----------------|-------------|---------|
| Intercept | -1.5974 | 0.3717 | -4.2979 | 0.0001 |
| Percent Under 21 | 0.2871 | 0.0294 | 9.7671 | 0.0000 |
The table indicates a significant relationship between the percentage of drivers under 21 and the number of fatal accidents, with a positive coefficient for the percentage under 21 variable, suggesting that as the percentage of younger drivers increases, so do fatal accidents.
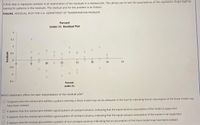
Transcribed Image Text:### Analyzing Residual Plots in Regression Analysis
**Purpose of Residual Plots**
In regression analysis, a crucial step is examining the residuals using a residual plot. This examination helps test the assumptions of the regression model by identifying patterns in the residuals.
**Figure Overview**
**Title:** Residual Plot for U.S. Department of Transportation Problem
- **Graph Components:**
- **X-axis:** Percent Under 21
- **Y-axis:** Residuals
- **Data Points:** Plotted residuals shown as "X" marks distributed across the range of the percent under 21 values.
**Interpretation**
The plot shows residuals scattered around a horizontal line at zero, with the residual spread appearing fairly consistent across different values of the "percent under 21." This consistency (or lack thereof) can provide insights into the suitability of the regression model.
**Questions for Evaluation:**
Which statement offers the best interpretation of the residual plot?
- ○ It appears that the residual plot exhibits a pattern whereby a linear model may not be adequate or the best fit, indicating that an assumption of the linear model may have been violated.
- ○ It appears that the residual plot exhibits a good pattern of constant variance, indicating that the equal variance assumption of the model is supported.
- ○ It appears that the residual plot exhibits a good pattern of constant variance, indicating that the equal variance assumption of the model is not supported.
- ○ It appears that the residual plot exhibits a pattern of non-constant variance, indicating that an assumption of the linear model may have been violated.
Understanding the pattern of residuals can indicate whether the assumptions about linearity and variance in the regression model are appropriate or if they have been violated.
Expert Solution

This question has been solved!
Explore an expertly crafted, step-by-step solution for a thorough understanding of key concepts.
This is a popular solution
Trending nowThis is a popular solution!
Step by stepSolved in 2 steps

Knowledge Booster
Learn more about
Need a deep-dive on the concept behind this application? Look no further. Learn more about this topic, economics and related others by exploring similar questions and additional content below.Similar questions
- Help!arrow_forwardAn analyst working for your firm provided an estimated log-linear demand function based on the natural logarithm of the quantity sold, price, and the average income of consumers. Results are summarized in the following table: SUMMARY OUTPUT Regression Statistics Multiple R R Square Adjusted R Square Standard Error Observations ANOVA Regression Residual Total Intercept LN Price LN Income df 0.968 0.937 0.933 0.003 30 SS MS F 2 0.003637484 0.001818742 202.48598 0.000242516 8.98206E-06 27 29 0.00388 Coefficients Standard Error 0.57 0.00 0.13 0.51 -0.08 0.15 t Stat 0.90 -19.50 1.13 P-value 0.37 0.00 0.27 Significance F 5.55598E-17 Lower 95% -0.65 -0.09 -0.12 How would a 4 percent increase in income impact the demand for your product? Demand would increase by 60 percent. Demand would increase by 0.6 percent. Demand would decrease by 60 percent. Demand would decrease by 0.6 percent. Upper 95% 1.68 -0.07 0.41arrow_forwardq11-arrow_forward
- The dependent variable in the regression in our cost driver analysis is which of the following? Company sales Total overhead cost for the entire period of time Total overhead cost per montharrow_forward6 9 13 Yi 7 18 9. 26 23 a. Which of the following scatter diagrams accurately represents the data? A. 24+ 24+ 20- 20- 16+ 16+ 12+ 12+ 8+ 8+ 4- -4 4 12 16 24 28 x -4 4 8. 12 16 20 24 28 x -4t -4t С. D. 24 -----24+ 20- 20- 16- 16- 20 B. 20 00 00 O.arrow_forwardplease answer in text form and in proper format answer with must explanation , calculation for each part and steps clearlyarrow_forward
- When running a ols regression, if my control variables are insignificant via T-test should I keep them in the regression? Are they significant?arrow_forwardplease answer in text form and in proper format answer with must explanation , calculation for each part and steps clearlyarrow_forwardConsider the following computer output of a multiple regression analysis relating annual salary to years of education and years of work experience. Regression Statistics Multiple R 0.7339 R Square 0.5386 Adjusted R Square 0.5185 Standard Error 2137.5200 Observations 49 ANOVA SS df Regression 2 245,370,679.3850 122,685,339.6925 26.8517 MS F Significance F 1.9E-08 Total Residual 46 210,173,612.6150 48 455,544,292.0000 4,568,991.5786 Coefficients Standard Error Intercept Education (Years) 14290.37278 2350.8671 2,528.5819 338.1140 Experience (Years) 829.3167 392.5627 t Stat P-value 5.6515 0.000000961 9200.6014 6.9529 0.000000011 2.1126 0.040093183 Lower 95 % Upper 95% 19,380.1442 1670.2789 3031.4553 39.129 1619.5044 Step 2 of 2: How much would you expect your salary to increase if you had one more year of education?arrow_forward
- Explain the OLS Estimator in Multiple Regression in detail?arrow_forwardYou estimated a regression with the following output. Source | SS df MS Number of obs = 223 -------------+---------------------------------- F(1, 221) = 17592.99 Model | 182392130 1 182392130 Prob > F = 0.0000 Residual | 2291176.96 221 10367.3166 R-squared = 0.9876 -------------+---------------------------------- Adj R-squared = 0.9875 Total | 184683307 222 831906.786 Root MSE = 101.82 ------------------------------------------------------------------------------ Y | Coef. Std. Err. t P>|t| [95% Conf. Interval] -------------+---------------------------------------------------------------- X | 11.97037 .0902481 132.64 0.000 11.79252 12.14823 _cons | 74.40159 10.96696 6.78 0.000 52.78839 96.01479…arrow_forwardq4-arrow_forward
arrow_back_ios
SEE MORE QUESTIONS
arrow_forward_ios
Recommended textbooks for you
- Principles of Economics (12th Edition)EconomicsISBN:9780134078779Author:Karl E. Case, Ray C. Fair, Sharon E. OsterPublisher:PEARSONEngineering Economy (17th Edition)EconomicsISBN:9780134870069Author:William G. Sullivan, Elin M. Wicks, C. Patrick KoellingPublisher:PEARSON
- Principles of Economics (MindTap Course List)EconomicsISBN:9781305585126Author:N. Gregory MankiwPublisher:Cengage LearningManagerial Economics: A Problem Solving ApproachEconomicsISBN:9781337106665Author:Luke M. Froeb, Brian T. McCann, Michael R. Ward, Mike ShorPublisher:Cengage LearningManagerial Economics & Business Strategy (Mcgraw-...EconomicsISBN:9781259290619Author:Michael Baye, Jeff PrincePublisher:McGraw-Hill Education
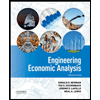
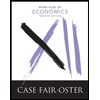
Principles of Economics (12th Edition)
Economics
ISBN:9780134078779
Author:Karl E. Case, Ray C. Fair, Sharon E. Oster
Publisher:PEARSON
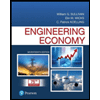
Engineering Economy (17th Edition)
Economics
ISBN:9780134870069
Author:William G. Sullivan, Elin M. Wicks, C. Patrick Koelling
Publisher:PEARSON
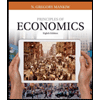
Principles of Economics (MindTap Course List)
Economics
ISBN:9781305585126
Author:N. Gregory Mankiw
Publisher:Cengage Learning
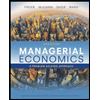
Managerial Economics: A Problem Solving Approach
Economics
ISBN:9781337106665
Author:Luke M. Froeb, Brian T. McCann, Michael R. Ward, Mike Shor
Publisher:Cengage Learning
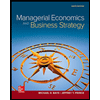
Managerial Economics & Business Strategy (Mcgraw-...
Economics
ISBN:9781259290619
Author:Michael Baye, Jeff Prince
Publisher:McGraw-Hill Education